Accelerating Model Synchronization for Distributed Machine Learning in an Optical Wide Area Network
Journal of optical communications and networking(2022)
摘要
Geo-distributed machine learning (Geo-DML) adopts a hierarchical training architecture that includes local model synchronization within the data center and global model synchronization (GMS) across data centers. However, the scarce and heterogeneous wide area network (WAN) bandwidth can become the bottleneck of training performance. An intelligent optical device (i.e., reconfigurable optical all-drop multiplexer) makes the modern WAN topology reconfigurable, which has been ignored by most approaches to speed up Geo-DML training. Therefore, in this paper, we study scheduling algorithms to accelerate model synchronization for Geo-DML training with consideration of the reconfigurable optical WAN topology. Specifically, we use an aggregation tree for each Geo-DML training job, which helps to reduce model synchronization communication overhead across the WAN, and propose two efficient algorithms to accelerate GMS for Geo-DML: MOptree, a model-based algorithm for single job scheduling, and MMOptree for multiple job scheduling, aiming to reconfigure the WAN topology and trees by reassigning wavelengths on each fiber. Based on the current WAN topology and job information, mathematical models are built to guide the topology reconstruction, wavelength, and bandwidth allocation for each edge of the trees. The simulation results show that MOptree completes the GMS stage up to 56.16% on average faster than the traditional tree without optical-layer reconfiguration, and MMOptree achieves up to 54.6% less weighted GMS time.
更多查看译文
关键词
Wide area networks,Topology,Bandwidth,Data centers,Training,Synchronization,Data models
AI 理解论文
溯源树
样例
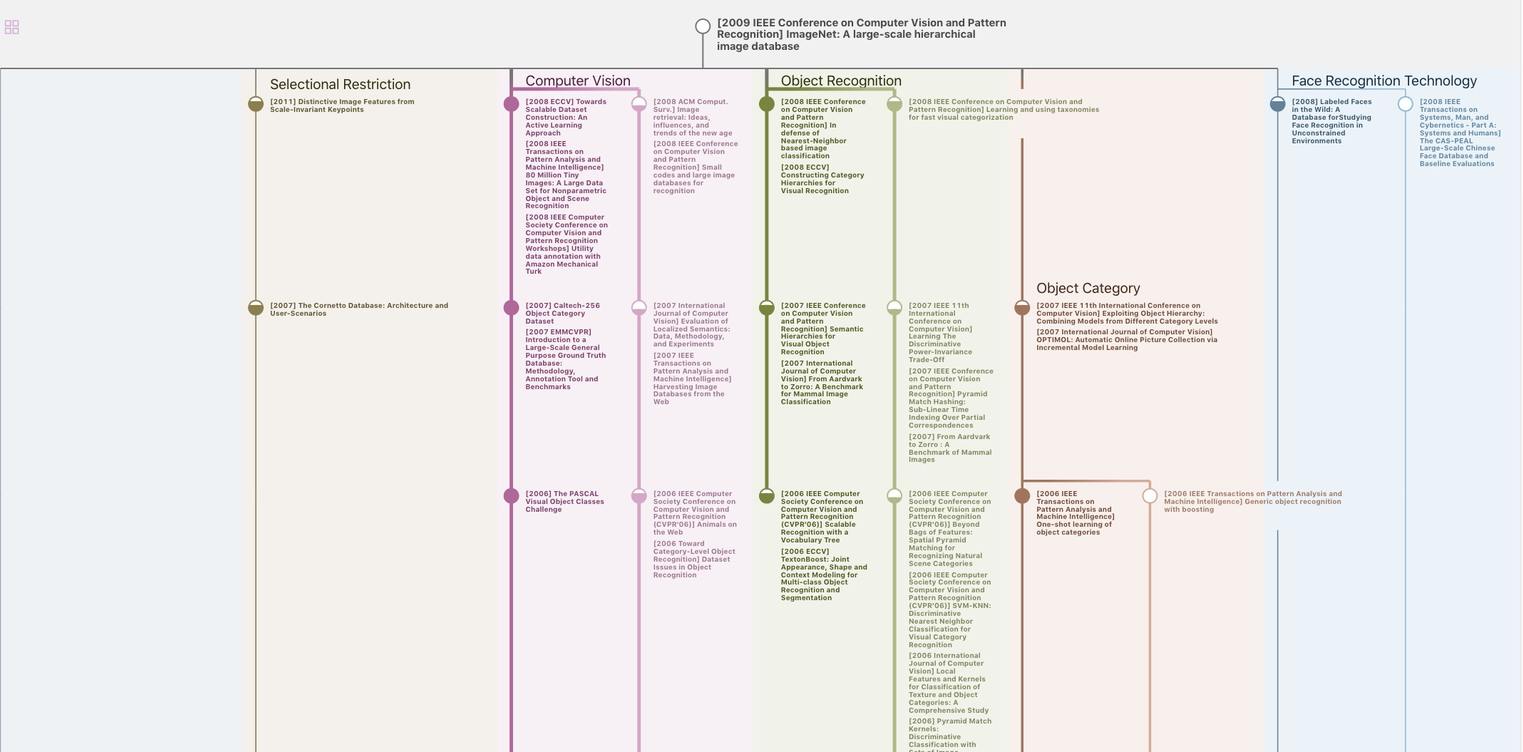
生成溯源树,研究论文发展脉络
Chat Paper
正在生成论文摘要