Dynamic Data-driven Microscopic Traffic Simulation using Jointly Trained Physics-guided Long Short-term Memory
ACM Transactions on Modeling and Computer Simulation(2022)
摘要
Symbiotic simulation systems that incorporate data-driven methods (such as machine/deep learning) are effective and efficient tools for just-in-time (JIT) operational decision making. With the growing interest on Digital Twin City, such systems are ideal for real-time microscopic traffic simulation. However, learning-based models are heavily biased towards the training data and could produce physically inconsistent outputs. In terms of microscopic traffic simulation, this could lead to unsafe driving behaviours causing vehicle collisions in the simulation. As for symbiotic simulation, this could severely affect the performance of real-time base simulation model resulting in inaccurate or unrealistic forecasts, which could, in turn, mislead JIT what-if analysis. To overcome this issue, a physics-guided data-driven modelling paradigm should be adopted so that the resulting model could capture both accurate and safe driving behaviours. However, very few works exist in the development of such a car-following model that can balance between simulation accuracy and physical consistency. Therefore, in this paper, a new “jointly-trained physics-guided Long Short-term Memory (JTPG-LSTM)” neural network, is proposed and integrated to a dynamic data-driven simulation system to capture dynamic car-following behaviours. An extensive set of experiments was conducted to demonstrate the advantages of the proposed model from both modelling and simulation perspectives.
更多查看译文
关键词
Data-driven modelling, car-following, physics-guided machine learning, online learning, just-in-time simulation, real-time simulation, symbiotic simulation, Digital Twin
AI 理解论文
溯源树
样例
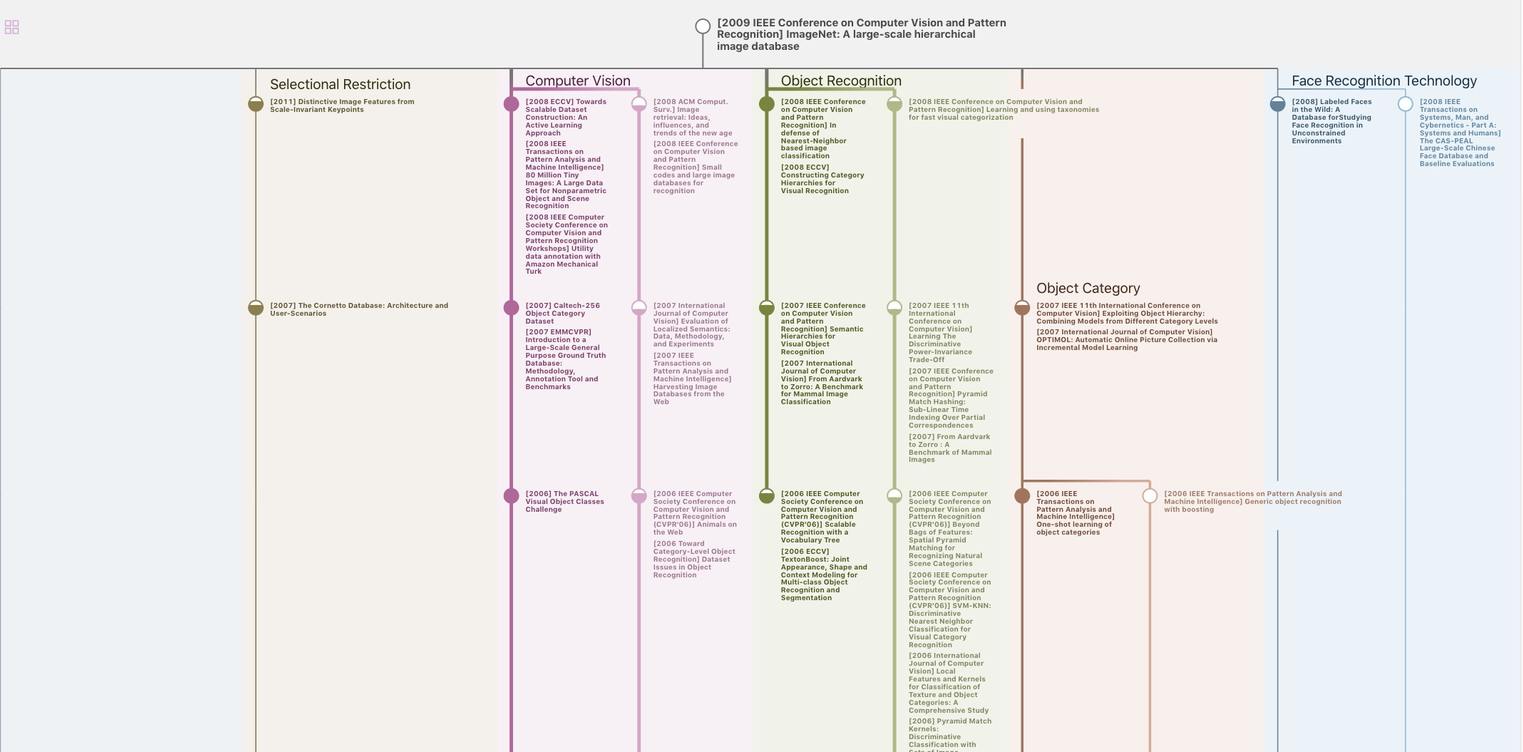
生成溯源树,研究论文发展脉络
Chat Paper
正在生成论文摘要