On Explainability in AI-Solutions: A Cross-Domain Survey
Lecture Notes in Computer Science Computer Safety, Reliability, and Security. SAFECOMP 2022 Workshops(2022)
摘要
Artificial Intelligence (AI) increasingly shows its potential to outperform predicate logic algorithms and human control alike. In automatically deriving a system model, AI algorithms learn relations in data that are not detectable for humans. This great strength, however, also makes use of AI methods dubious. The more complex a model, the more difficult it is for a human to understand the reasoning for the decisions. As currently, fully automated AI algorithms are sparse, every algorithm has to provide a reasoning for human operators. For data engineers, metrics such as accuracy and sensitivity are sufficient. However, if models are interacting with non-experts, explanations have to be understandable. This work provides an extensive survey of literature on this topic, which, to a large part, consists of other surveys. The findings are mapped to ways of explaining decisions and reasons for explaining decisions. It shows that the heterogeneity of reasons and methods of and for explainability lead to individual explanatory frameworks.
更多查看译文
关键词
Artificial intelligence, Explainability, Survey, Cross-domain
AI 理解论文
溯源树
样例
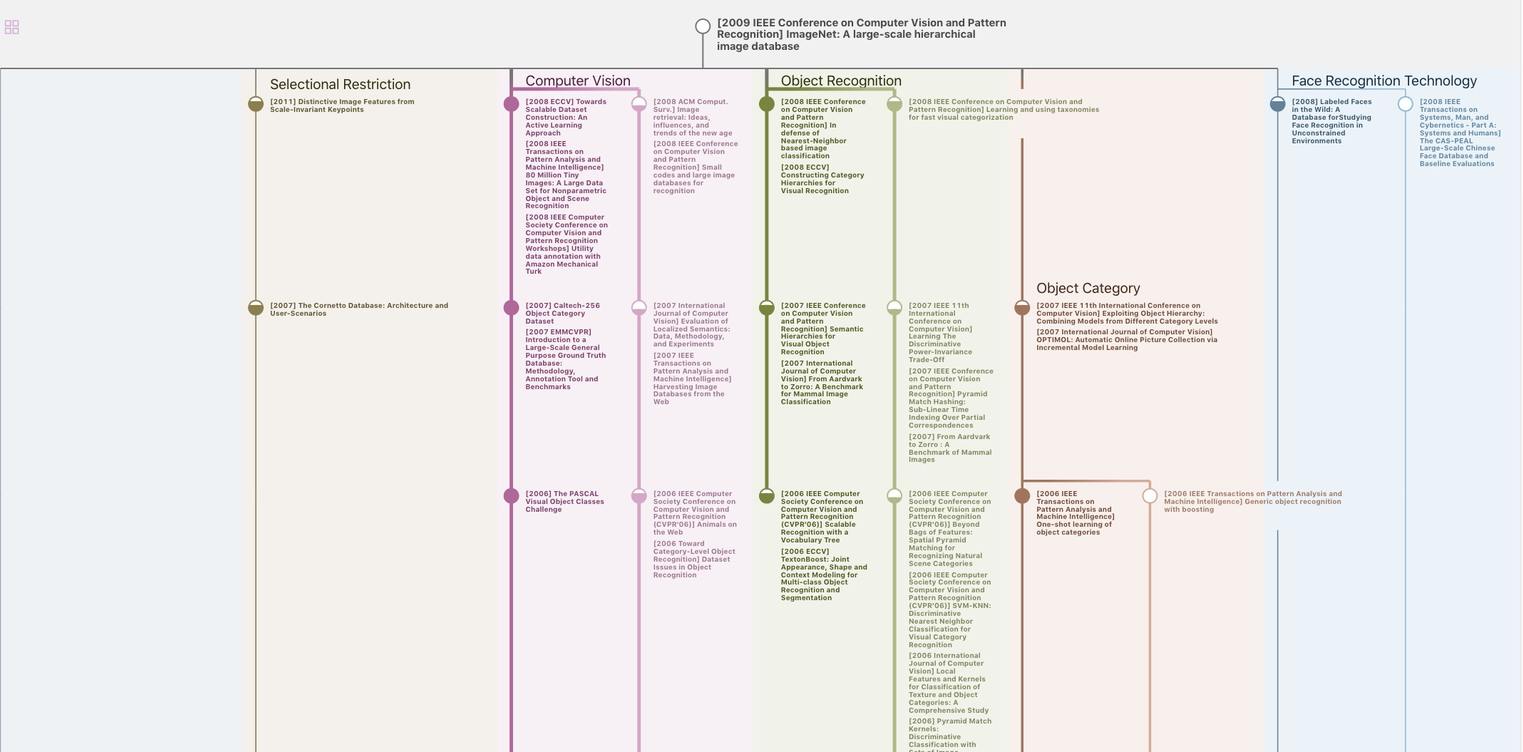
生成溯源树,研究论文发展脉络
Chat Paper
正在生成论文摘要