Efficient Approach to Automated Pavement Manhole Detection with Modified Faster R-CNN
Intelligent Transportation Infrastructure(2022)
摘要
Abstract Presently, more and more attention has been paid to the detection of road facilities. Pavement manhole is an important type of road facilities, and can result in tangible impacts on driving safety and comfort. This paper proposes a robust method based on a modification of the Faster Region Convolutional Neural Network (Faster R-CNN) to detect pavement manholes automatically. We establish a manually-annotated image library that consists of 1245 manhole images collected by 1-mm laser imaging system, and implement the modified Faster R-CNN architecture to locate manholes exclusively under realistic and complex environments. Compared with the original Faster R-CNN, the proposed modification is to replace the feature extractor used in the original Faster R-CNN with a more-efficient backbone ResNet50, and implement Feature Pyramid Network (FPN) to fuse multi-scale features. The experimental results demonstrate that the modified Faster R-CNN outperforms the original Faster R-CNN and other state-of-the-art models, including YOLOv4, EfficientDet and YOLOX. The F1-score and Overall-IOU achieved by the modified Faster R-CNN on 250 testing images are 98.15% and 92.07% respectively. To further verify the robustness of the proposed method, the modified Faster R-CNN is applied to process manhole images which are taken randomly by a smart phone and thus highly differ from manhole images acquired by the laser imaging system. It is found that the modified Faster R-CNN can also yield similar detection efficiency even for images representing highly dissimilar viewing angles and unforeseen scenarios, implying the benefits of deep-learning-based object detection algorithms to intelligent investigation of pavement manholes.
更多查看译文
AI 理解论文
溯源树
样例
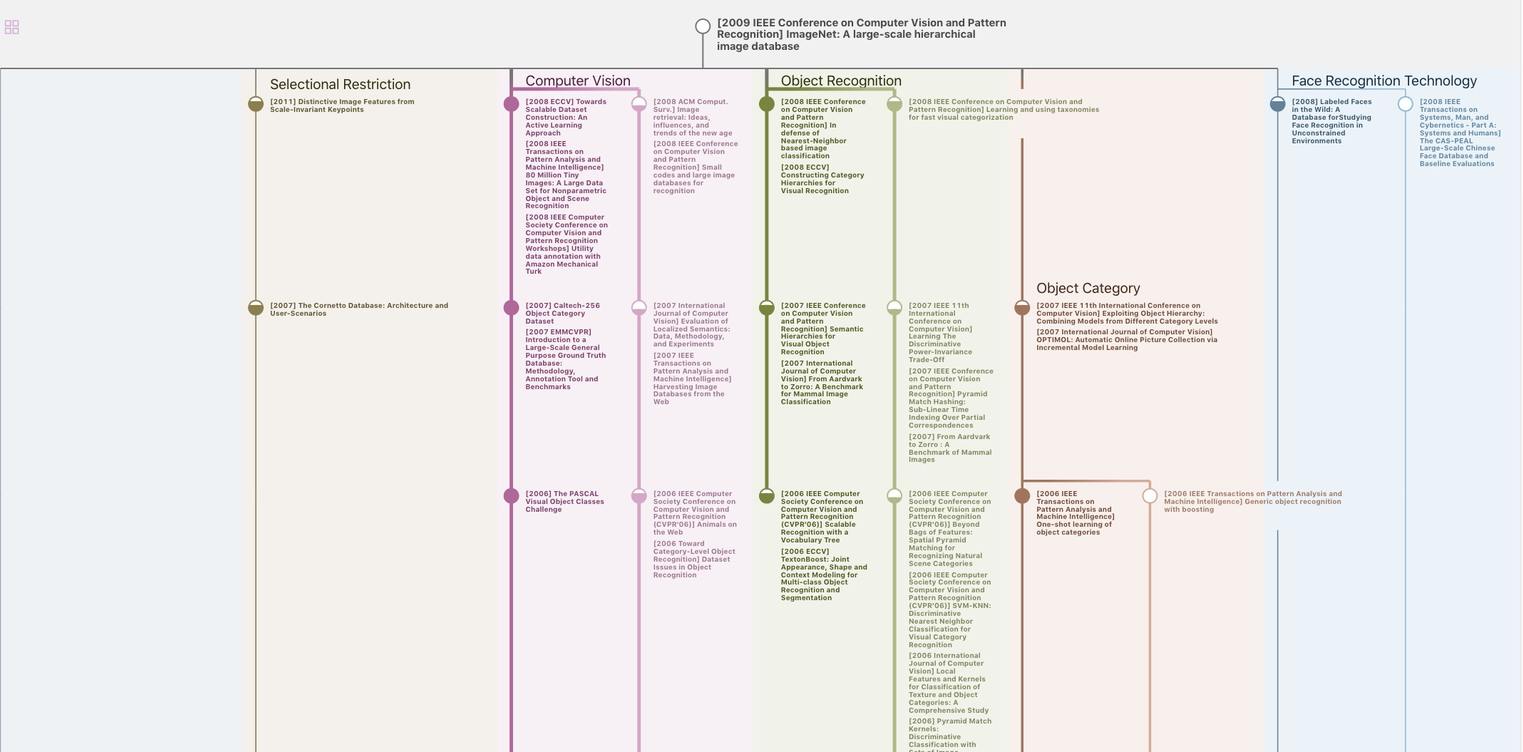
生成溯源树,研究论文发展脉络
Chat Paper
正在生成论文摘要