About the Confusion-Matrix-based Assessment of the Results of Imbalanced Data Classification
Informatika(2021)
The United Institute of Informatics Problems of the National Academy of Sciences of Belarus
Abstract
When applying classifiers in real applications, the data imbalance often occurs when the number of elements of one class is greater than another. The article examines the estimates of the classification results for this type of data. The paper provides answers to three questions: which term is a more accurate translation of the phrase "confusion matrix", how preferable to represent data in this matrix, and what functions to be better used to evaluate the results of classification by such a matrix. The paper demonstrates on real data that the popular accuracy function cannot correctly estimate the classification errors for imbalanced data. It is also impossible to compare the values of this function, calculated by matrices with absolute quantitative results of classification and normalized by classes. If the data is imbalanced, the accuracy calculated from the confusion matrix with normalized values will usually have lower values, since it is calculated by a different formula. The same conclusion is made for most of the classification accuracy functions used in the literature for estimation of classification results. It is shown that to represent confusion matrices it is better to use absolute values of object distribution by classes instead of relative ones, since they give an idea of the amount of data tested for each class and their imbalance. When constructing classifiers, it is recommended to evaluate errors by functions that do not depend on the data imbalance, that allows to hope for more correct classification results for real data.
MoreTranslated text
Key words
classification,imbalanced data,confusion matrix,classification accuracy functions,accuracy paradox,neural network,disease diagnosis
求助PDF
上传PDF
View via Publisher
AI Read Science
AI Summary
AI Summary is the key point extracted automatically understanding the full text of the paper, including the background, methods, results, conclusions, icons and other key content, so that you can get the outline of the paper at a glance.
Example
Background
Key content
Introduction
Methods
Results
Related work
Fund
Key content
- Pretraining has recently greatly promoted the development of natural language processing (NLP)
- We show that M6 outperforms the baselines in multimodal downstream tasks, and the large M6 with 10 parameters can reach a better performance
- We propose a method called M6 that is able to process information of multiple modalities and perform both single-modal and cross-modal understanding and generation
- The model is scaled to large model with 10 billion parameters with sophisticated deployment, and the 10 -parameter M6-large is the largest pretrained model in Chinese
- Experimental results show that our proposed M6 outperforms the baseline in a number of downstream tasks concerning both single modality and multiple modalities We will continue the pretraining of extremely large models by increasing data to explore the limit of its performance
Upload PDF to Generate Summary
Must-Reading Tree
Example
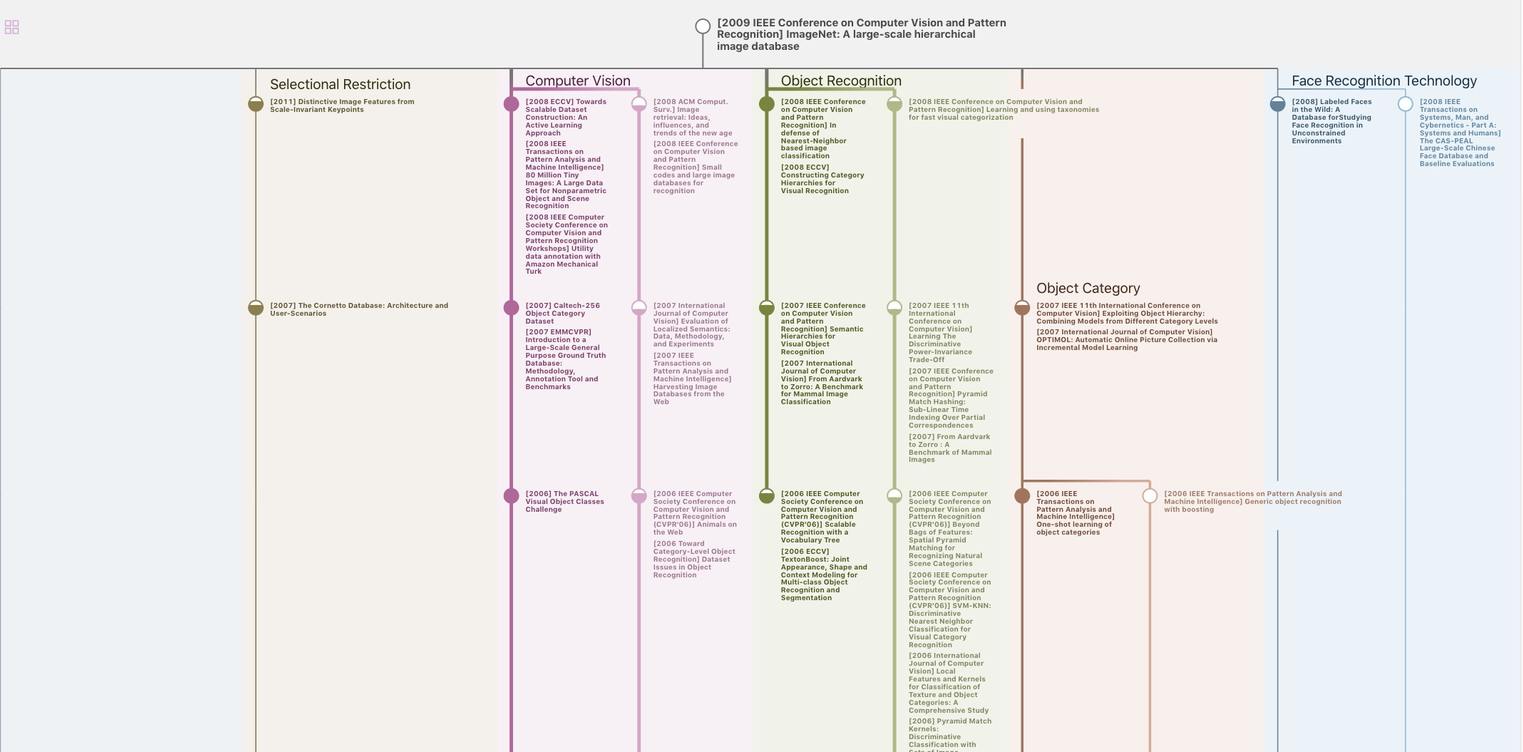
Generate MRT to find the research sequence of this paper
Related Papers
2001
被引用2897 | 浏览
2014
被引用160 | 浏览
Data Disclaimer
The page data are from open Internet sources, cooperative publishers and automatic analysis results through AI technology. We do not make any commitments and guarantees for the validity, accuracy, correctness, reliability, completeness and timeliness of the page data. If you have any questions, please contact us by email: report@aminer.cn
Chat Paper
GPU is busy, summary generation fails
Rerequest