"Is your explanation stable?": A Robustness Evaluation Framework for Feature Attribution
Computer and Communications Security(2022)
摘要
ABSTRACTNeural networks have become increasingly popular. Nevertheless, understanding their decision process turns out to be complicated. One vital method to explain a models' behavior is feature attribution, i.e., attributing its decision to pivotal features. Although many algorithms are proposed, most of them aim to improve the faithfulness (fidelity) to the model. However, the real environment contains many random noises, which may cause the feature attribution maps to be greatly perturbed for similar images. More seriously, recent works show that explanation algorithms are vulnerable to adversarial attacks, generating the same explanation for a maliciously perturbed input. All of these make the explanation hard to trust in real scenarios, especially in security-critical applications. To bridge this gap, we propose Median Test for Feature Attribution (MeTFA) to quantify the uncertainty and increase the stability of explanation algorithms with theoretical guarantees. MeTFA is method-agnostic, i.e., it can be applied to any feature attribution method. MeTFA has the following two functions: (1) examine whether one feature is significantly important or unimportant and generate a MeTFA-significant map to visualize the results; (2) compute the confidence interval of a feature attribution score and generate a MeTFA-smoothed map to increase the stability of the explanation. Extensive experiments show that MeTFA improves the visual quality of explanations and significantly reduces the instability while maintaining the faithfulness of the original method. To quantitatively evaluate MeTFA's faithfulness and stability, we further propose several robust faithfulness metrics, which can evaluate the faithfulness of an explanation under different noise settings. Experiment results show that the MeTFA-smoothed explanation can significantly increase the robust faithfulness. In addition, we use two typical applications to show MeTFA's potential in the applications. First, when being applied to the SOTA explanation method to locate context bias for semantic segmentation models, MeTFA-significant explanations use far smaller regions to maintain 99%+ faithfulness. Second, when testing with different explanation-oriented attacks, MeTFA can help defend vanilla, as well as adaptive, adversarial attacks against explanations.
更多查看译文
AI 理解论文
溯源树
样例
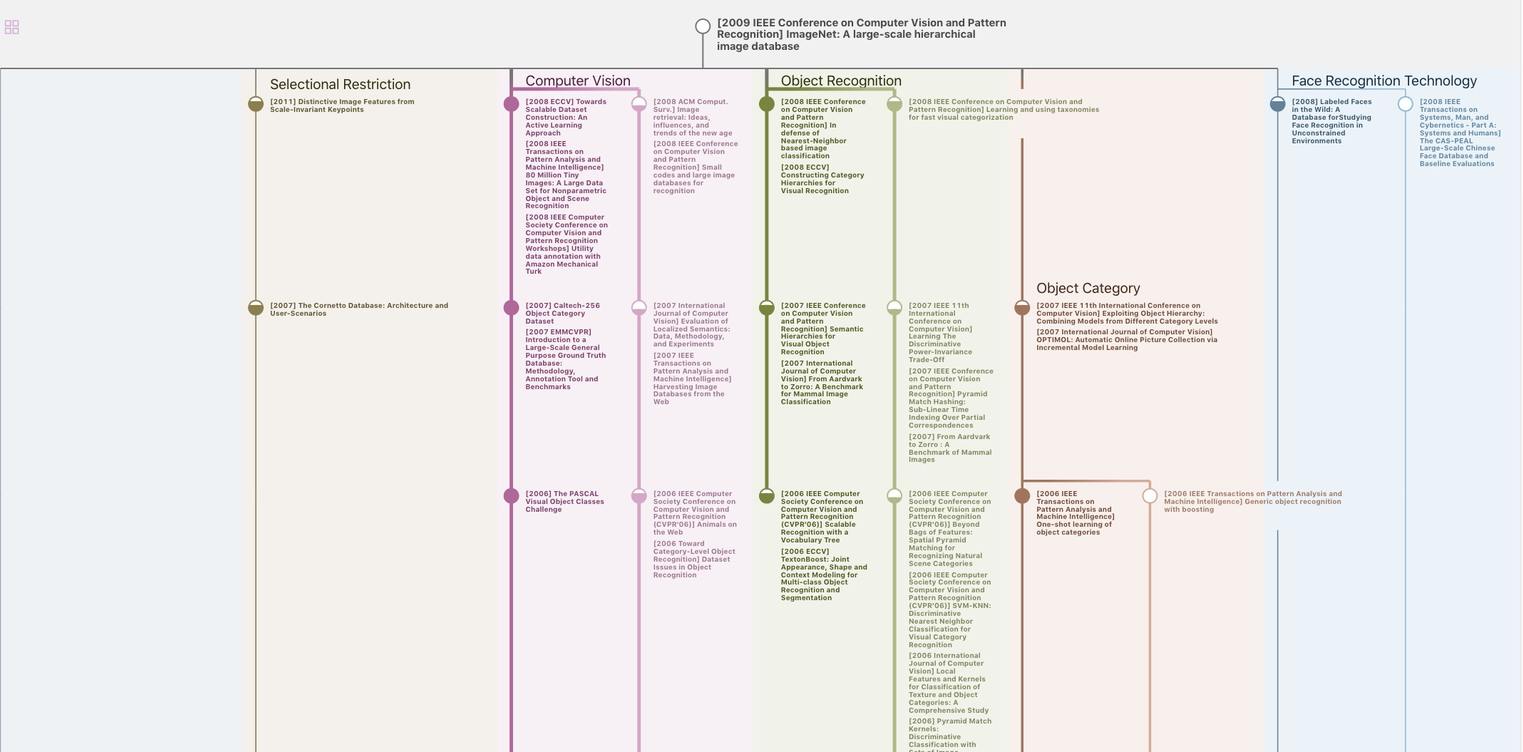
生成溯源树,研究论文发展脉络
Chat Paper
正在生成论文摘要