Harvesting the Ly alpha forest with convolutional neural networks
MONTHLY NOTICES OF THE ROYAL ASTRONOMICAL SOCIETY(2022)
摘要
We develop a machine learning based algorithm using a convolutional neural network (CNN) to identify low H I column density Ly alpha absorption systems (log N-H I/cm(-2) < 17) in the Ly alpha forest, and predict their physical properties, such as their H I column density (log N-H I/cm(-2)), redshift (z(H I)), and Doppler width (b(H I)). Our CNN models are trained using simulated spectra (S/N similar or equal to 10), and we test their performance on high quality spectra of quasars at redshift z similar to 2.5-2.9 observed with the High Resolution Echelle Spectrometer on the Keck I telescope. We find that similar to 78 per cent of the systems identified by our algorithm are listed in the manual Voigt profile fitting catalogue. We demonstrate that the performance of our CNN is stable and consistent for all simulated and observed spectra with S/N greater than or similar to 10. Our model can therefore be consistently used to analyse the enormous number of both low and high S/N data available with current and future facilities. Our CNN provides state-of-the-art predictions within the range 12.5 <= log N-H I/cm(-2) < 15.5 with a mean absolute error of Delta(log N-H (I)/cm(-2) = 0.13, Delta(z(H I)) = 2.7 x 10(-5), and Delta(b(H I)) = 4.1 km s(-1). The CNN prediction costs < 3 min per model per spectrum with a size of 120 000 pixels using a laptop computer. We demonstrate that CNNs can significantly increase the efficiency of analysing Ly alpha forest spectra, and thereby greatly increase the statistics of Ly alpha absorbers.
更多查看译文
关键词
methods: data analysis, galaxies: high-redshift, intergalactic medium, quasars: absorption lines
AI 理解论文
溯源树
样例
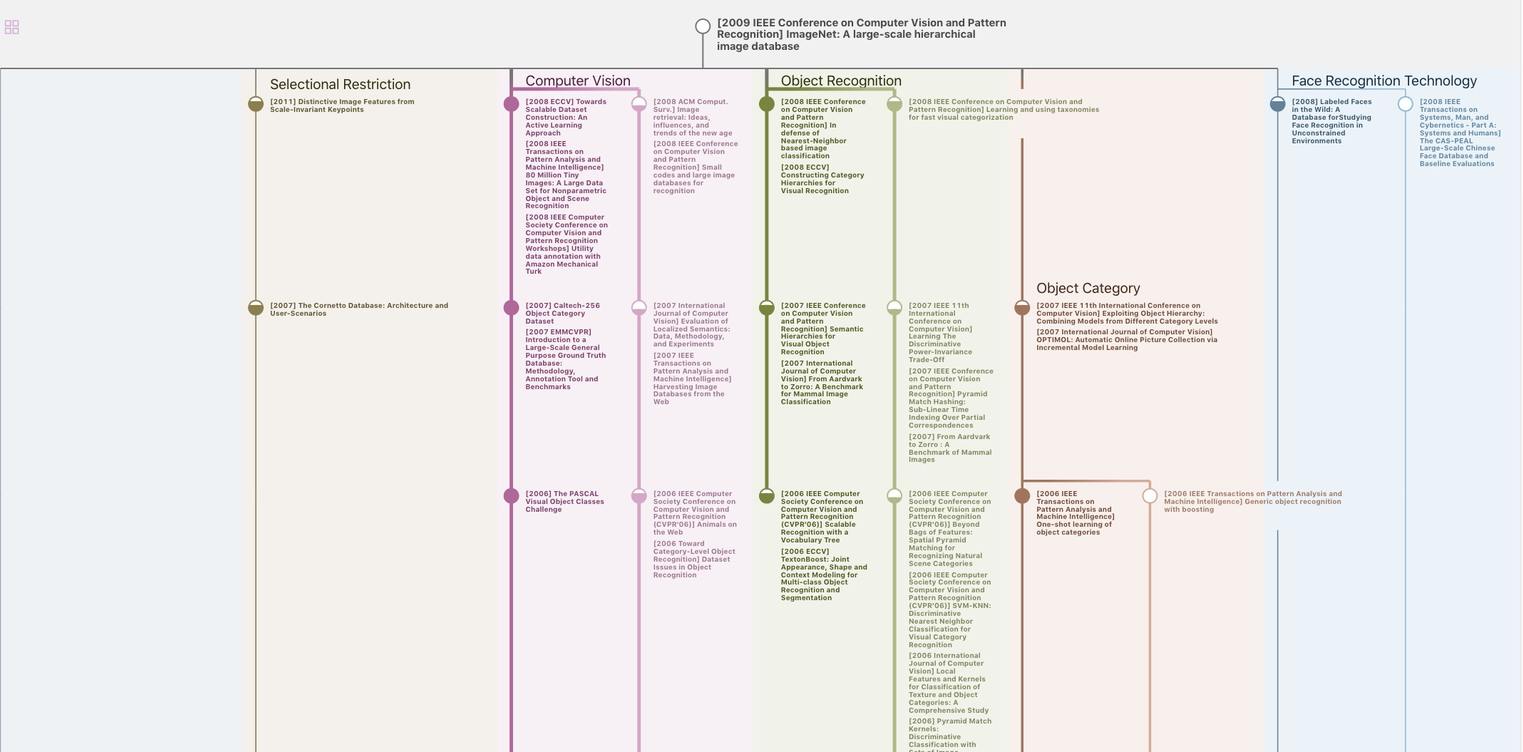
生成溯源树,研究论文发展脉络
Chat Paper
正在生成论文摘要