Deep Neural Network Augmented Wireless Channel Estimation for Preamble-Based OFDM PHY on Zynq System on Chip
arxiv(2023)
摘要
Reliable and fast channel estimation is crucial for next-generation wireless networks supporting a wide range of vehicular and low-latency services. Recently, deep learning (DL)-based channel estimation has been explored as an efficient alternative to conventional least-square (LS) and linear minimum mean square error (LMMSE) approaches. Most of these DL approaches have not been realized on system on chip (SoC), and preliminary study shows that their complexity exceeds the complexity of the entire physical layer (PHY). The high latency of DL is another concern. This article considers the design and implementation of deep neural network (DNN) augmented LS (LSDNN)-based channel estimation for preamble-based orthogonal frequency-division multiplexing (OFDM) PHY on SoC. We demonstrate the gain in performance compared with the conventional LS and LMMSE approaches. Via software–hardware codesign, word-length optimization, and reconfigurable architectures, we demonstrate the superiority of the LSDNN over LS and LMMSE for a wide range of signal-to-noise ratio (SNR), number of pilots, preamble types, and wireless channels. Furthermore, we evaluate the performance, power, and area (PPA) of the LS and LSDNN application-specific integrated circuit (ASIC) implementations in 45-nm technology. We demonstrate that word-length optimization can substantially improve PPA for the proposed architecture in ASIC implementations.
更多查看译文
关键词
Wireless communication, Channel estimation, OFDM, Symbols, Wireless sensor networks, Signal to noise ratio, Training, Application-specific integrated circuit (ASIC), channel estimation, Index Terms, deep learning (DL), field-programmable gate array (FPGA), hardware-software codesign, least-square (LS), linear minimum mean square error (LMMSE), system on chip (SoC)
AI 理解论文
溯源树
样例
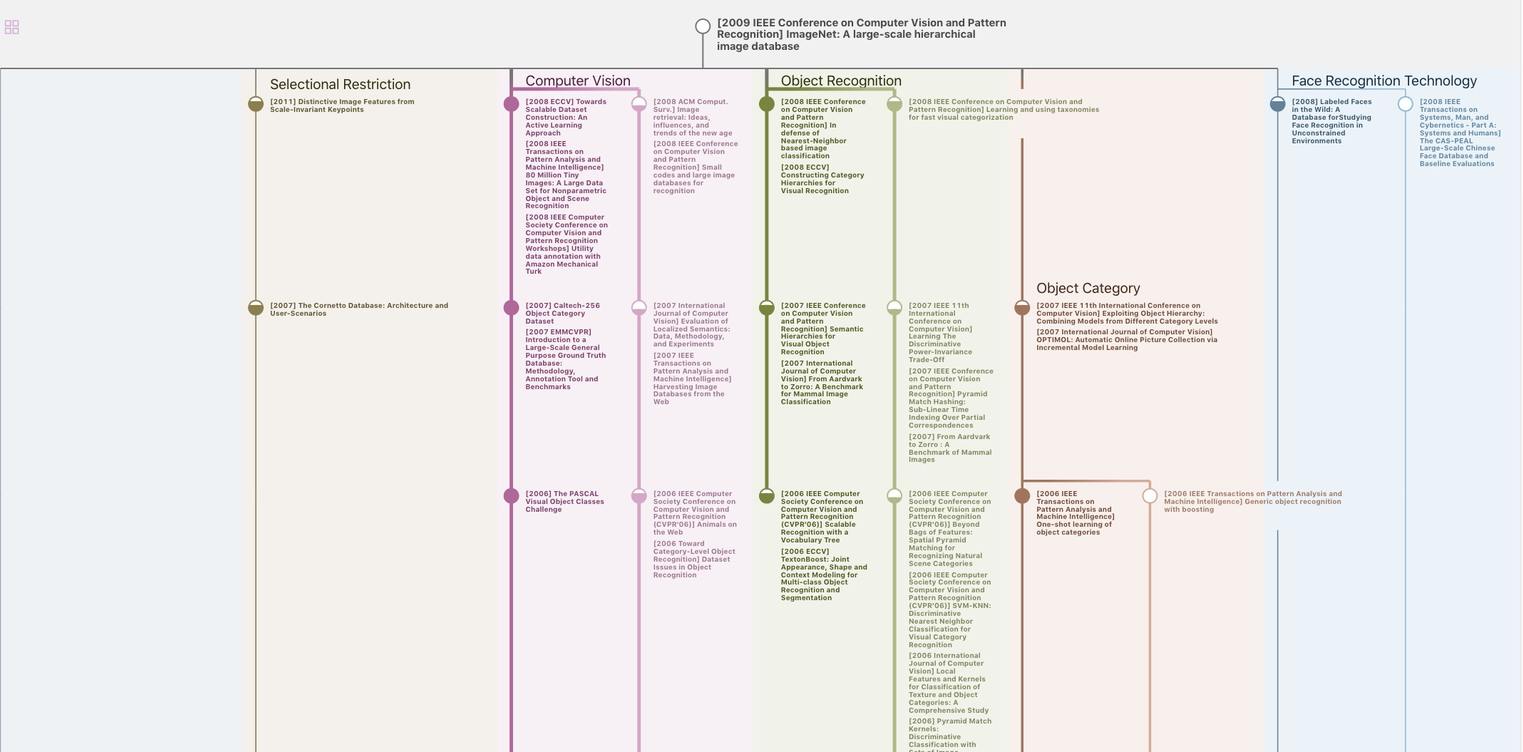
生成溯源树,研究论文发展脉络
Chat Paper
正在生成论文摘要