Learning to Predict on Octree for Scalable Point Cloud Geometry Coding
2022 IEEE 5th International Conference on Multimedia Information Processing and Retrieval (MIPR)(2022)
摘要
Octree-based point cloud representation and compression have been adopted by the MPEG G-PCC standard. However, it only uses handcrafted methods to predict the probability that a leaf node is non-empty, which is then used for entropy coding. We propose a novel approach for predicting such probabilities for geometry coding, which applies a denoising neural network to a “noisy” context cube that includes both neighboring decoded voxels as well as uncoded voxels. We further propose a convolution-based model to upsample the decoded point cloud at a coarse resolution on the decoder side. Integration of the two approaches significantly improves the rate-distortion performance for geometry coding compared to the original G-PCC standard and other baseline methods for dense point clouds. The proposed octree-based entropy coding approach is naturally scalable, which is desirable for dynamic rate adaptation in point cloud streaming systems.
更多查看译文
关键词
Point Cloud,Point Cloud Coding,Point Cloud Compression
AI 理解论文
溯源树
样例
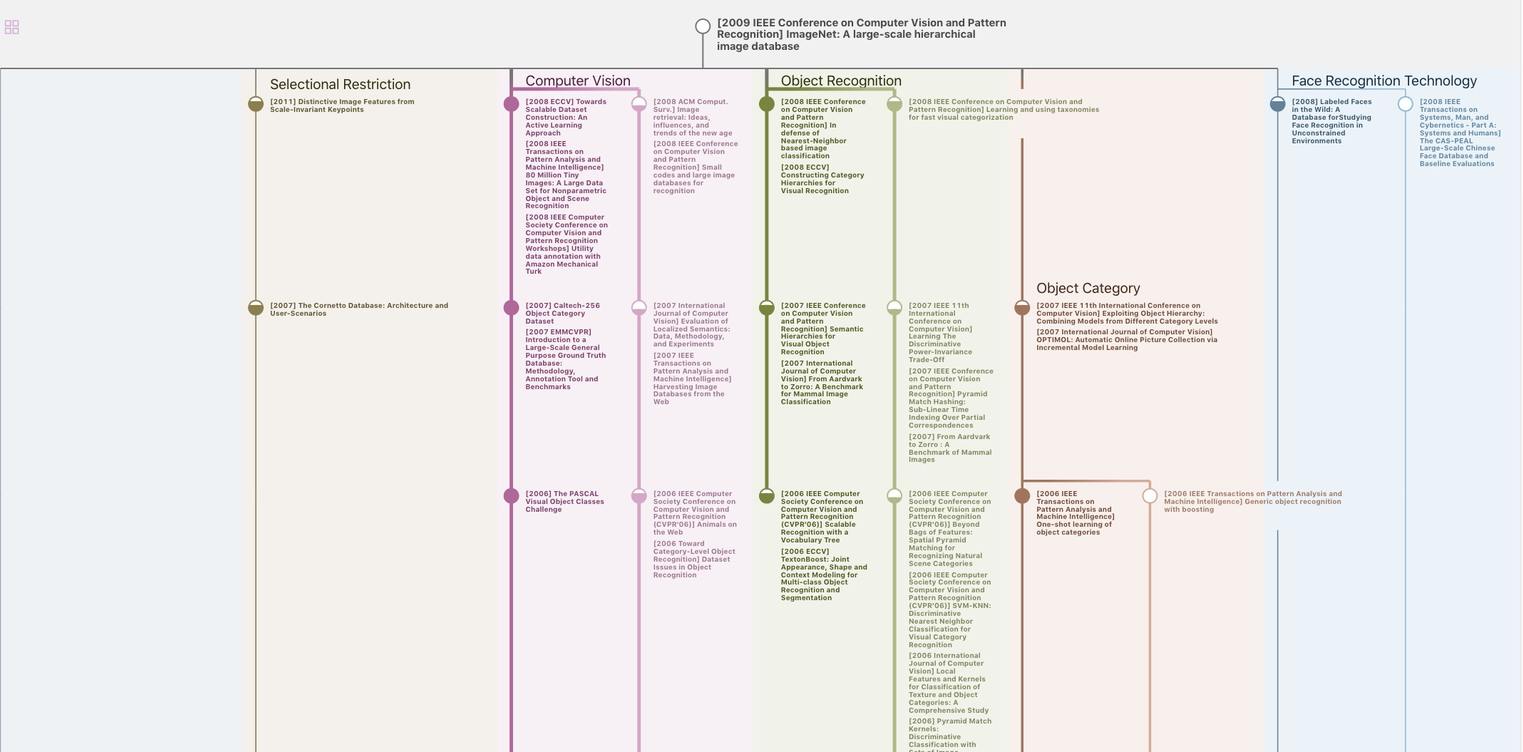
生成溯源树,研究论文发展脉络
Chat Paper
正在生成论文摘要