Extreme learning machine models for predicting the n-octanol/water partition coefficient (Kow) data of organic compounds
Journal of Environmental Chemical Engineering(2022)
摘要
The partition coefficient of n-octanol/water (Kow) is generally considered a valuable characteristic in many natural sciences fields. Predictive quantitative structure-property and relationship (QSPR) models for Kow are encouraged since the experimental data are not always available. Extreme learning machine (ELM) method with the advantages of fast learning speed and good generalization ability has been applied to various domains. This work introduced COSMO descriptors (Sσ-profile) of molecules as descriptors and the ELM algorithm to construct estimation models for Kow of organics. Four ELM models were developed and their outcomes were compared with the MLR models produced by the same descriptors. Results revealed that the proposed ELM models were reliable and applicable to predicting the logKow values of compounds, especially the ELM-4 model with the best correlation coefficient R2 (0.949) and root-mean-square error RMSE (0.358). Therefore, the proposed approaches are effective and feasible, and can be extensively generalized to forecast other important properties of chemicals.
更多查看译文
关键词
ELM,Octanol-water partition coefficient,COSMO σ-profile,Organic chemicals
AI 理解论文
溯源树
样例
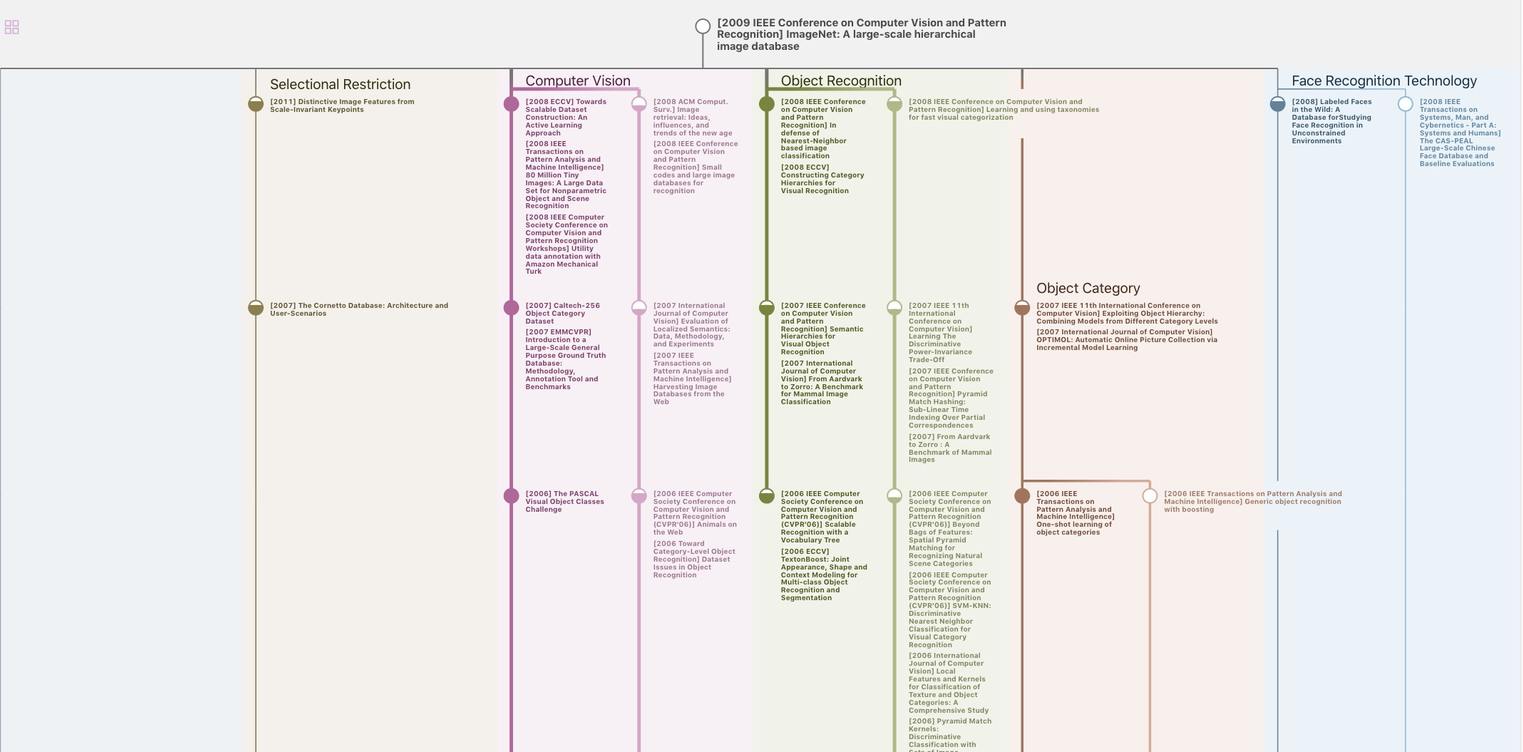
生成溯源树,研究论文发展脉络
Chat Paper
正在生成论文摘要