Deep reinforcement learning in an ultrafiltration system: Optimizing operating pressure and chemical cleaning conditions
Chemosphere(2022)
摘要
Enhancing engineering efficiency and reducing operating costs are permanent subjects that face all engineers over the world. To effectively improve the performance of filtration systems, it is necessary to determine an optimal operating condition beyond conventional methods of periodic and empirical operation. Herein, this paper proposes an effective approach to finding an optimal operating strategy using deep reinforcement learning (DRL), particularly for an ultrafiltration (UF) system. Deep learning was developed to represent the UF system utilizing a long-short term memory and provided an environment for DRL. DRL was designed to control three actions; operating pressure, cleaning time, and cleaning concentration. Ultimately, DRL proposed the UF system to actively change the operating pressure and cleaning conditions over time toward better water productivity and operating efficiency. DRL denoted ∼20.9% of specific energy consumption can be reduced by increasing average water flux (39.5–43.7 L m−2 h−1) and reducing operating pressure (0.617–0.540 bar). Moreover, the optimal action of DRL was reasonable to achieve better performance beyond the conventional operation. Crucially, this study demonstrated that due to the nature of DRL, the approach is tractable for engineering systems that have structurally complex relationships among operating conditions and resultants.
更多查看译文
关键词
Deep reinforcement learning,Machine learning,Ultrafiltration,Chemical cleaning,Optimization
AI 理解论文
溯源树
样例
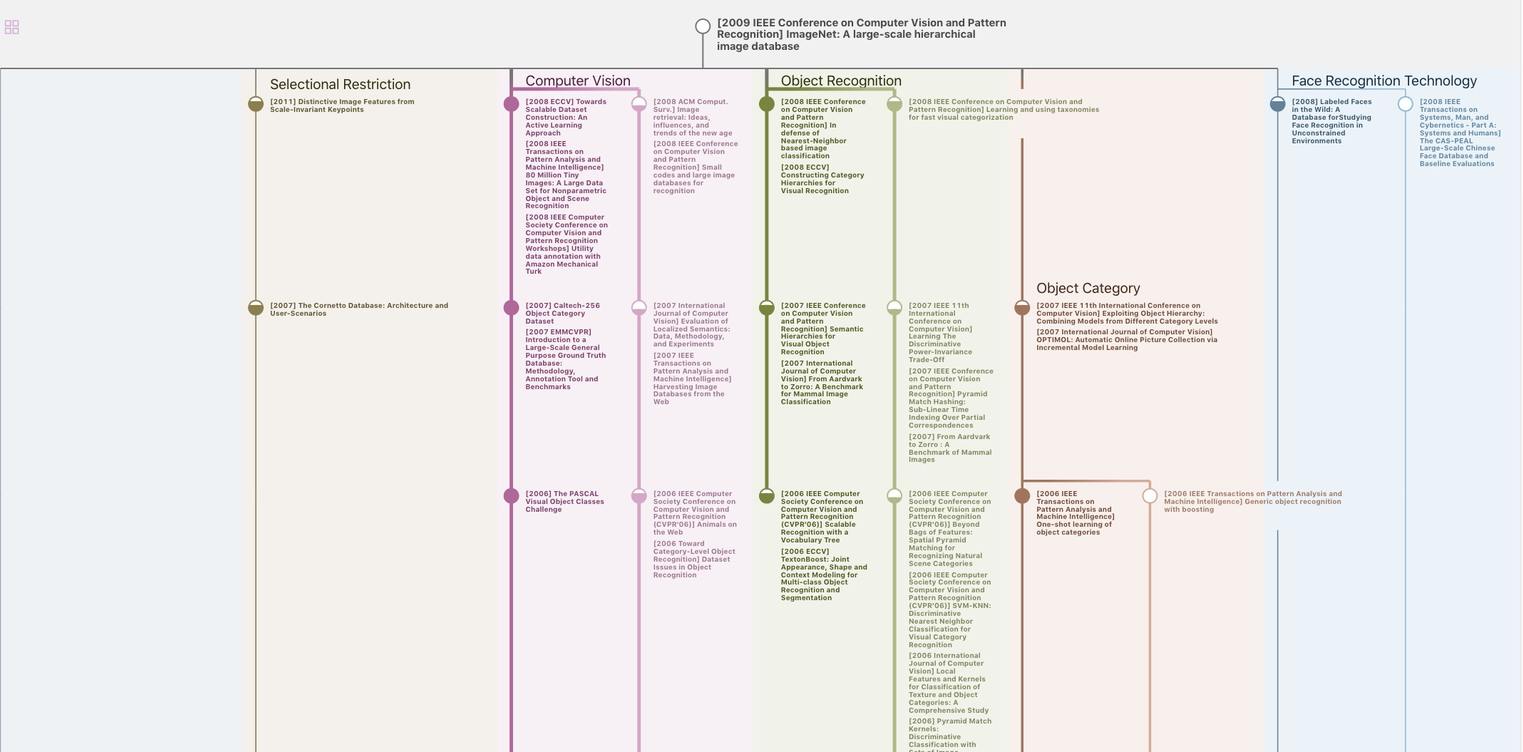
生成溯源树,研究论文发展脉络
Chat Paper
正在生成论文摘要