Improved Genetic Algorithm for Substation Location Optimization
2022 IEEE 4th International Conference on Power, Intelligent Computing and Systems (ICPICS)(2022)
Power Grid Planning & Research Center Guizhou Power Grid Co
Abstract
Aiming at the substation location optimization with multi constraints and multi indexes, an improved genetic algorithm is proposed. Combined with the characteristics and requirements of substation location, the real number coding strategy and elite retention scheme are adopted. The minimum annual cost of substation planning is regarded as the fitness to realize the adaptive search of spatial solution in spatial range, which can effectively solve the problems of local optimal solution and premature. The probability of mutation and crossover is determined according to individual fitness differentiation, which improves the convergence speed and solution accuracy of the algorithm. The example results show that the algorithm has good optimization ability, convergence characteristics, simple operation and fast running speed. It can better meet the needs of substation location selection.
MoreTranslated text
Key words
location selection,genetic algorithm,fitness,substation
求助PDF
上传PDF
View via Publisher
AI Read Science
AI Summary
AI Summary is the key point extracted automatically understanding the full text of the paper, including the background, methods, results, conclusions, icons and other key content, so that you can get the outline of the paper at a glance.
Example
Background
Key content
Introduction
Methods
Results
Related work
Fund
Key content
- Pretraining has recently greatly promoted the development of natural language processing (NLP)
- We show that M6 outperforms the baselines in multimodal downstream tasks, and the large M6 with 10 parameters can reach a better performance
- We propose a method called M6 that is able to process information of multiple modalities and perform both single-modal and cross-modal understanding and generation
- The model is scaled to large model with 10 billion parameters with sophisticated deployment, and the 10 -parameter M6-large is the largest pretrained model in Chinese
- Experimental results show that our proposed M6 outperforms the baseline in a number of downstream tasks concerning both single modality and multiple modalities We will continue the pretraining of extremely large models by increasing data to explore the limit of its performance
Upload PDF to Generate Summary
Must-Reading Tree
Example
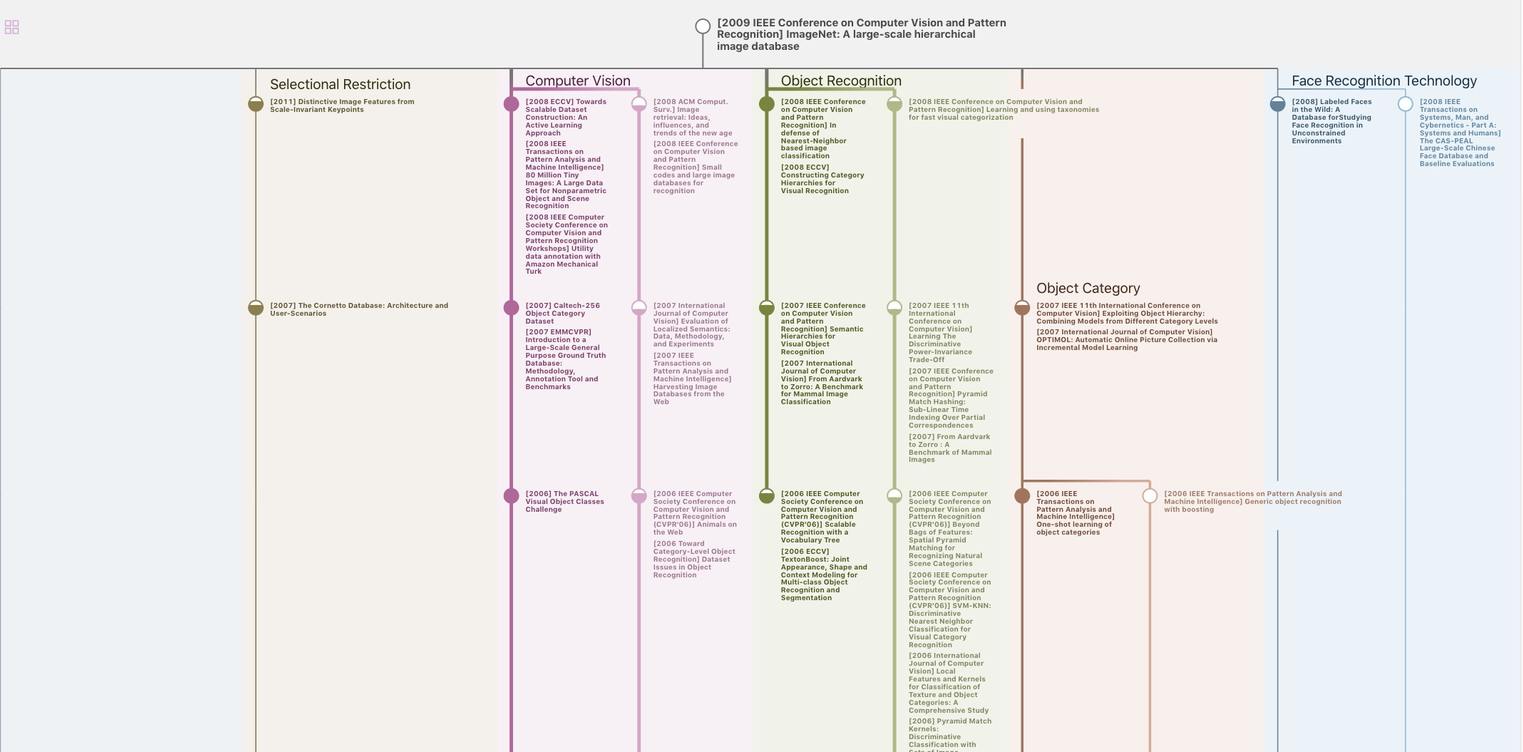
Generate MRT to find the research sequence of this paper
Related Papers
2014
被引用157 | 浏览
2015
被引用10 | 浏览
2017
被引用38 | 浏览
2019
被引用19 | 浏览
Data Disclaimer
The page data are from open Internet sources, cooperative publishers and automatic analysis results through AI technology. We do not make any commitments and guarantees for the validity, accuracy, correctness, reliability, completeness and timeliness of the page data. If you have any questions, please contact us by email: report@aminer.cn
Chat Paper
GPU is busy, summary generation fails
Rerequest