A Context-Based Decision-Making Trust Scheme for Malicious Detection in Connected and Autonomous Vehicles
2022 International Conference on Computing, Electronics & Communications Engineering (iCCECE)(2022)
摘要
The fast-evolving Intelligent Transportation Systems (ITS) are crucial in the 21st century, promising answers to congestion and accidents that bother people worldwide. ITS applications such as Connected and Autonomous Vehicle (CAVs) update and broadcasts road incident event messages, and this requires significant data to be transmitted between vehicles for a decision to be made in real-time. However, broadcasting trusted incident messages such as accident alerts between vehicles pose a challenge for CAVs. Most of the existing-trust solutions are based on the vehicle's direct interaction base reputation and the psychological approaches to evaluate the trustworthiness of the received messages. This paper provides a scheme for improving trust in the received incident alert messages for real-time decision-making to detect malicious alerts between CAVs using direct and indirect interactions. This paper applies artificial intelligence and statistical data classification for decision-making on the received messages. The model is trained based on the US Department of Technology Safety Pilot Deployment Model (SPMD). An Autonomous Decision-making Trust Scheme (ADmTS) that incorporates a machine learning algorithm and a local trust manager for decision-making has been developed. The experiment showed that the trained model could make correct predictions such as 98% and 0.55% standard deviation accuracy in predicting false alerts on the 25% malicious data
更多查看译文
关键词
context-based,decision-making,features selection and classifications,machine learning,trust
AI 理解论文
溯源树
样例
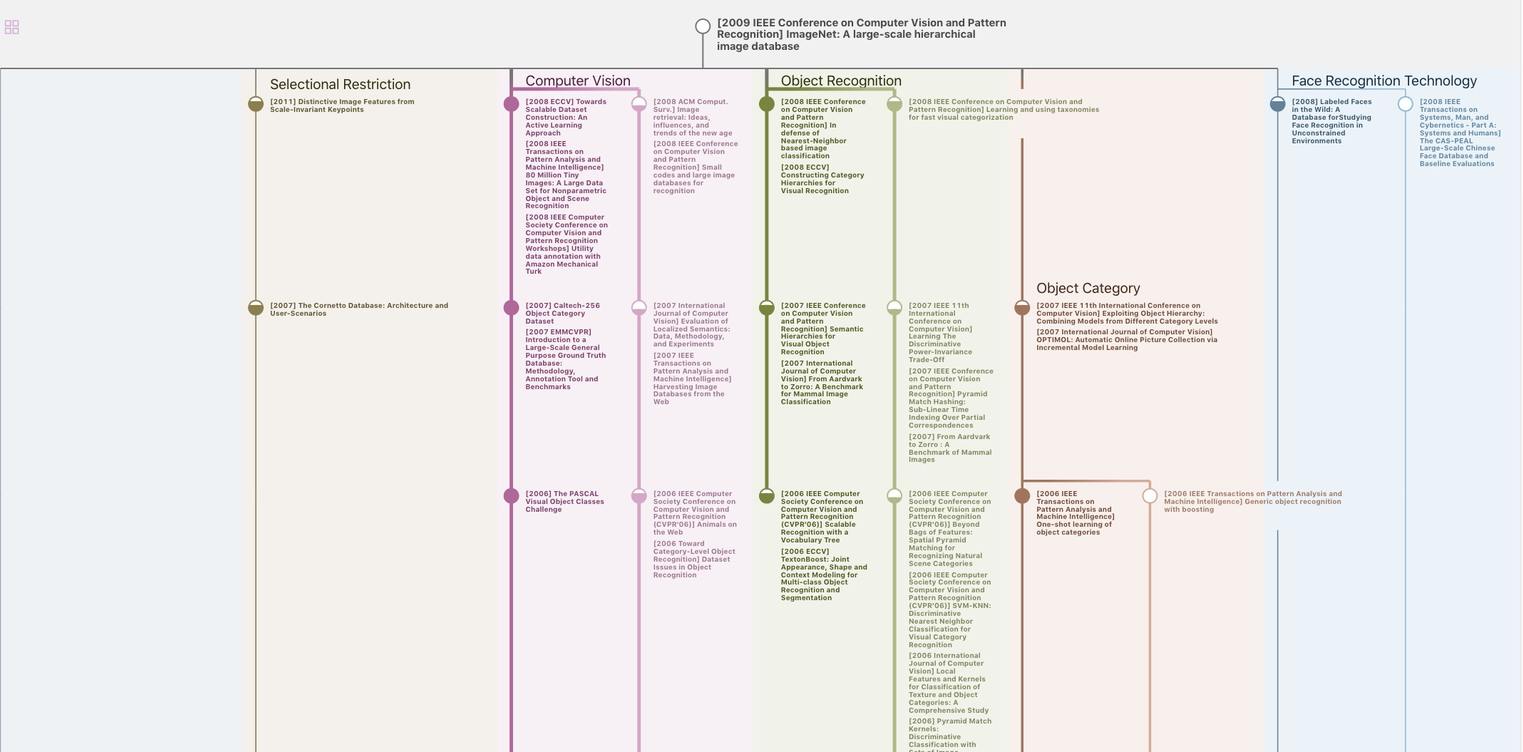
生成溯源树,研究论文发展脉络
Chat Paper
正在生成论文摘要