Towards a Dynamic Inter-Sensor Correlations Learning Framework for Multi-Sensor-Based Wearable Human Activity Recognition
PROCEEDINGS OF THE ACM ON INTERACTIVE MOBILE WEARABLE AND UBIQUITOUS TECHNOLOGIES-IMWUT(2022)
摘要
Multi-sensor-based wearable human activity recognition (WHAR) is a research hotspot in the field of ubiquitous computing. Extracting effective features from multi-sensor data is essential to improve the performance of activity recognition. Despite the excellent achievements of previous works, the challenge remains for modelling the dynamic correlations between sensors. In this paper, we propose a lightweight yet efficient GCN-based dynamic inter-sensor correlations learning framework called DynamicWHAR for automatically learning the dynamic correlations between sensors. DynamicWHAR is mainly composed of two modules: Initial Feature Extraction and Dynamic Information Interaction. Firstly, Initial Feature Extraction module performs data-to-feature transformation to extract the initial features of each sensor. Subsequently, Dynamic Information Interaction module explicitly models the specific interaction intensity between any two sensors, and performs dynamic information aggregation between sensors by the learned interaction intensity. Extensive experiments on four diverse WHAR datasets and two different resource-constrained devices validate that DynamicWHAR outperforms the SOTA models in both recognition performance and computational complexity.
更多查看译文
关键词
human activity recognition,wearable sensors,graph convolution network,information fusion
AI 理解论文
溯源树
样例
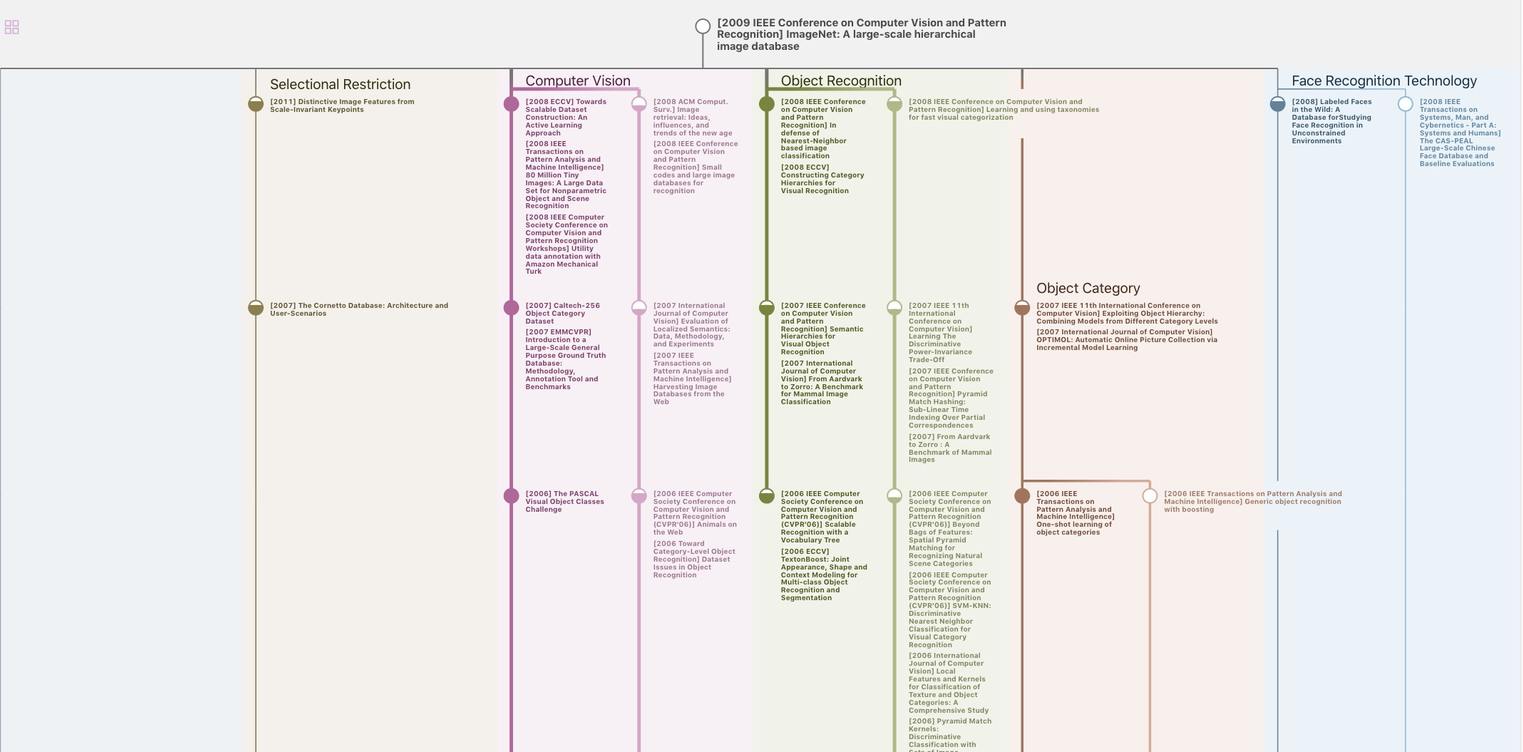
生成溯源树,研究论文发展脉络
Chat Paper
正在生成论文摘要