Optimizing Demonstrated Robot Manipulation Skills for Temporal Logic Constraints.
IEEE/RJS International Conference on Intelligent RObots and Systems (IROS)(2022)
摘要
For performing robotic manipulation tasks, the core problem is determining suitable trajectories that fulfill the task requirements. Various approaches to compute such trajectories exist, being learning and optimization the main driving techniques. Our work builds on the learning-from-demonstration (LfD) paradigm, where an expert demonstrates motions, and the robot learns to imitate them. However, expert demonstrations are not sufficient to capture all sorts of task specifications, such as the timing to grasp an object. In this paper, we propose a new method that considers formal task specifications within LfD skills. Precisely, we leverage Signal Temporal Logic (STL), an expressive form of temporal properties of systems, to formulate task specifications and use black-box optimization (BBO) to adapt an LfD skill accordingly. We demonstrate our approach in simulation and on a real industrial setting using several tasks that showcase how our approach addresses the LfD limitations using STL and BBO.
更多查看译文
关键词
demonstrated robot manipulation skills,temporal logic
AI 理解论文
溯源树
样例
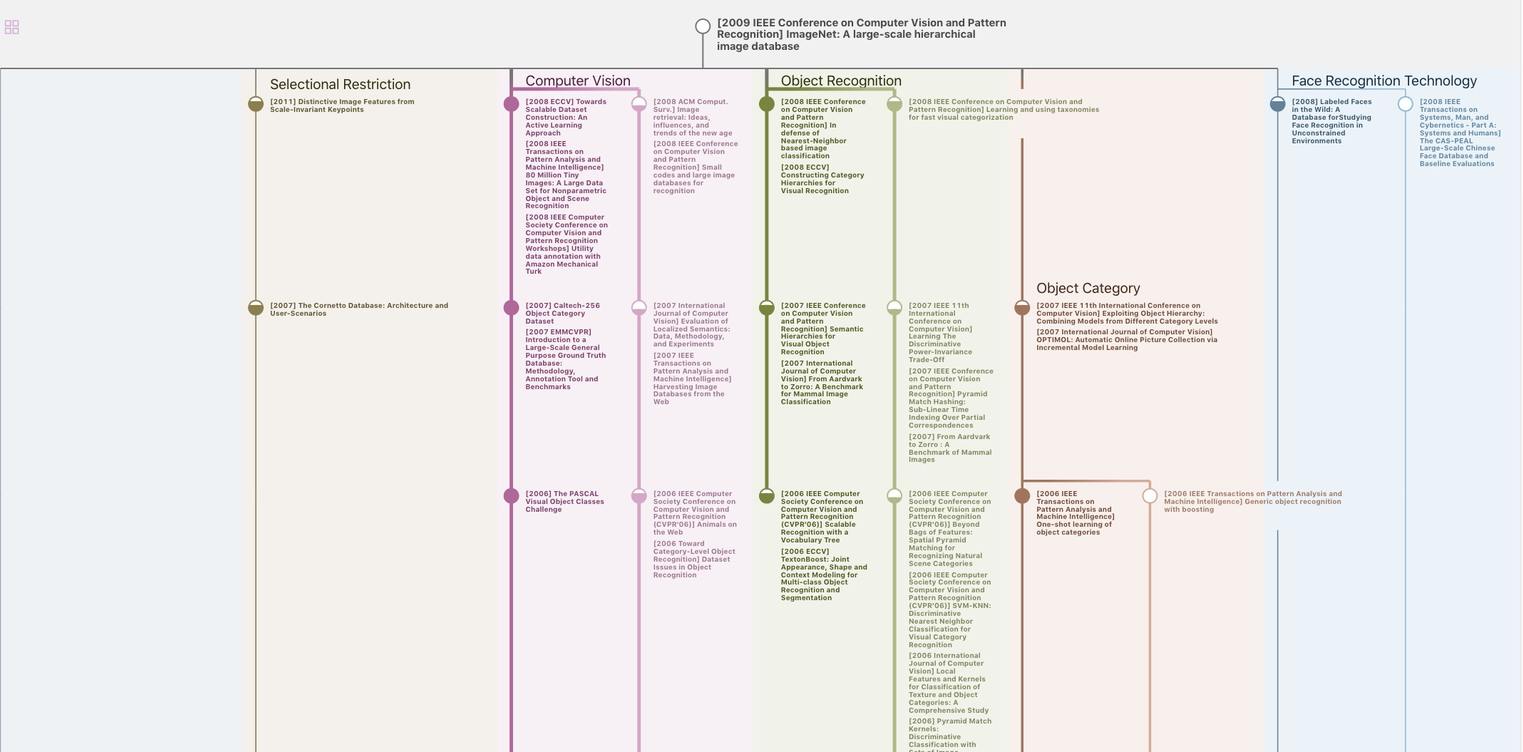
生成溯源树,研究论文发展脉络
Chat Paper
正在生成论文摘要