Real-to-Sim: Predicting Residual Errors of Robotic Systems with Sparse Data using a Learning-based Unscented Kalman Filter
arxiv(2023)
摘要
Achieving highly accurate dynamic or simulator models that are close to the real robot can facilitate model-based controls (e.g., model predictive control or linear-quadradic regulators), model-based trajectory planning (e.g., trajectory optimization), and decrease the amount of learning time necessary for reinforcement learning methods. Thus, the objective of this work is to learn the residual errors between a dynamic and/or simulator model and the real robot. This is achieved using a neural network, where the parameters of a neural network are updated through an Unscented Kalman Filter (UKF) formulation. Using this method, we model these residual errors with only small amounts of data -- a necessity as we improve the simulator/dynamic model by learning directly from real-world operation. We demonstrate our method on robotic hardware (e.g., manipulator arm, and a wheeled robot), and show that with the learned residual errors, we can further close the reality gap between dynamic models, simulations, and actual hardware.
更多查看译文
关键词
unscented kalman filter,robotic systems,residual errors,real-to-sim,learning-based
AI 理解论文
溯源树
样例
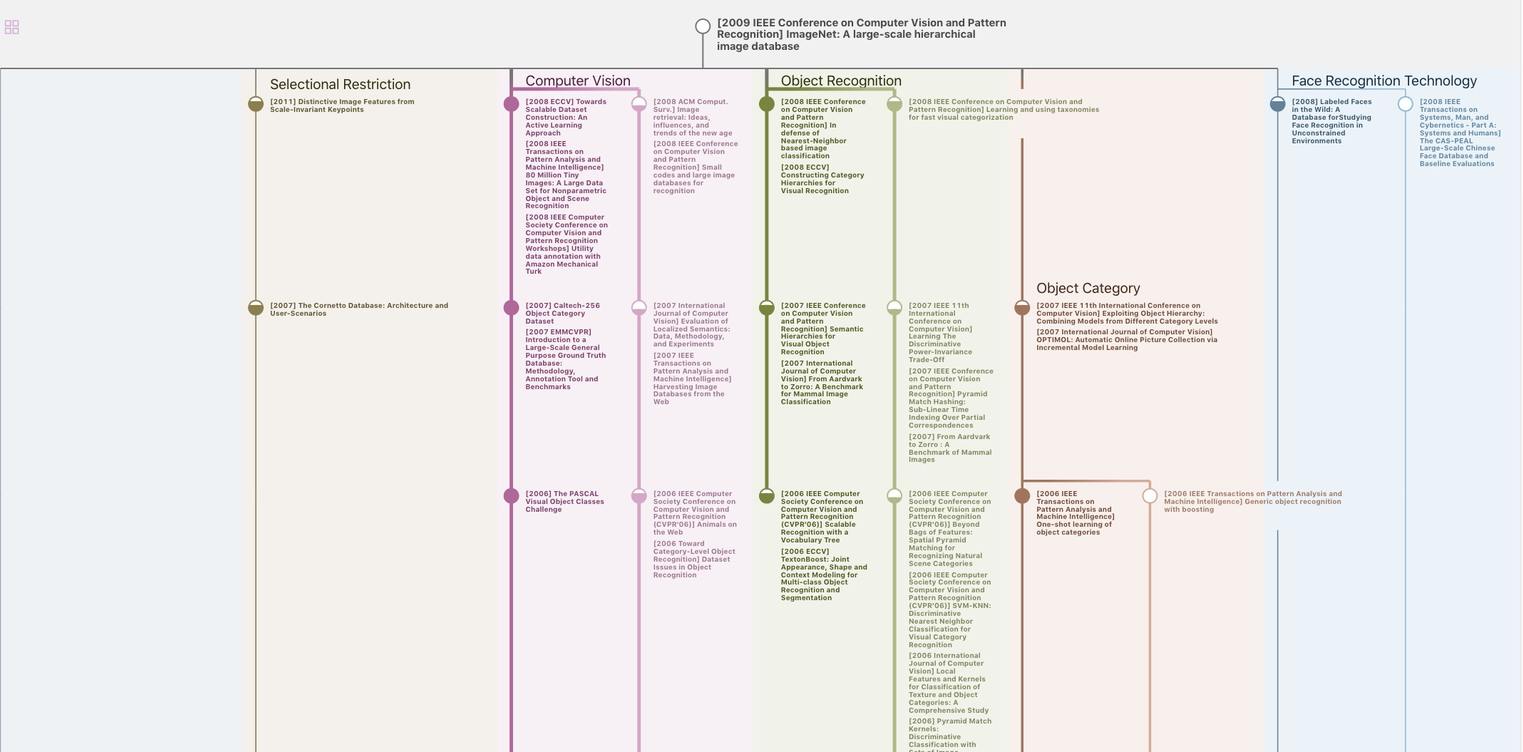
生成溯源树,研究论文发展脉络
Chat Paper
正在生成论文摘要