Ensemble Method Using Real Images, Metadata and Synthetic Images for Control of Class Imbalance in Classification.
Artificial life and robotics(2022)
摘要
Binary classification and anomaly detection face the problem of class imbalance in data sets. The contribution of this paper is to provide an ensemble model that improves image binary classification by reducing the class imbalance between the minority and majority classes in a data set. The ensemble model is a classifier of real images, synthetic images, and metadata associated with the real images. First, we apply a generative model to synthesize images of the minority class from the real image data set. Secondly, we train the ensemble model jointly with synthesized images of the minority class, real images, and metadata. Finally, we evaluate the model performance using a sensitivity metric to observe the difference in classification resulting from the adjustment of class imbalance. Improving the imbalance of the minority class by adding half the size of the majority class we observe an improvement in the classifier's sensitivity by 12% and 24% for the benchmark pre-trained models of RESNET50 and DENSENet121 respectively.
更多查看译文
关键词
Image classification,Patient metadata,Chest X-rays,Pneumonia detection,Imbalance data,Image synthesis
AI 理解论文
溯源树
样例
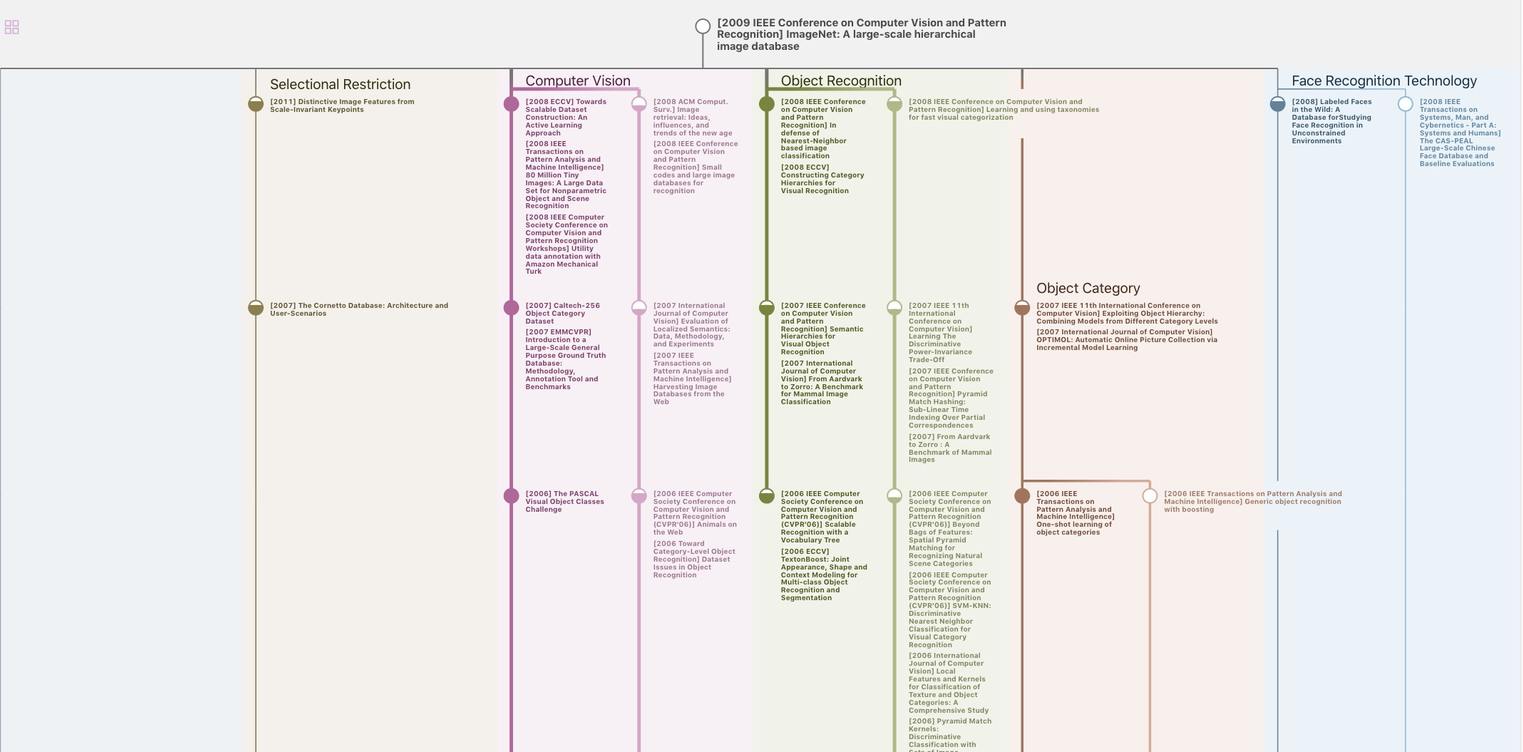
生成溯源树,研究论文发展脉络
Chat Paper
正在生成论文摘要