DeepActsNet: Spatial and Motion features from Face, Hands, and Body Combined with Convolutional and Graph Networks for Improved Action Recognition
CoRR(2020)
摘要
Existing action recognition methods mainly focus on joint and bone information in human body skeleton data due to its robustness to complex backgrounds and dynamic characteristics of the environments. In this paper, we combine body skeleton data with spatial and motion information from face and two hands, and present Deep Action Stamps (DeepActs), a novel data representation to encode actions from video sequences. We also present DeepActsNet, a deep learning based model with modality-specific Convolutional and Graph sub-networks for highly accurate action recognition based on Deep Action Stamps. Experiments on three challenging action recognition datasets (NTU60, NTU120, and SYSU) show that DeepActs produce considerable improvements in the recognition performance of standard convolutional and graph networks. Experiments also show that the fusion of modality-specific convolutional and structural features learnt by our DeepActsNet yields consistent improvements in action recognition accuracy over the state-of-the-art on the target datasets.
更多查看译文
AI 理解论文
溯源树
样例
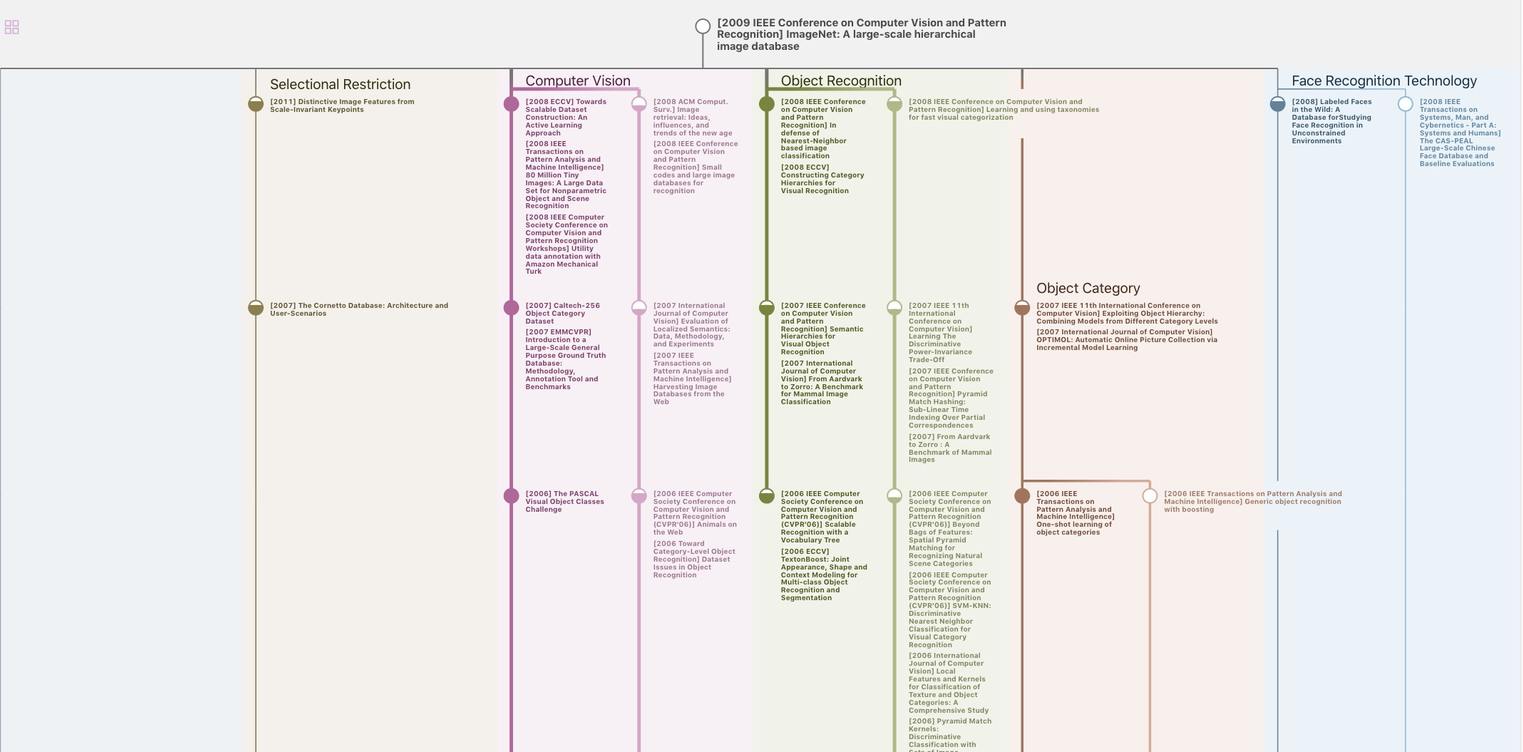
生成溯源树,研究论文发展脉络
Chat Paper
正在生成论文摘要