Counterexample-Driven Genetic Programming for Symbolic Regression With Formal Constraints
IEEE TRANSACTIONS ON EVOLUTIONARY COMPUTATION(2023)
摘要
In symbolic regression with formal constraints, the conventional formulation of regression problem is extended with desired properties of the target model, like symmetry, monotonicity, or convexity. We present a genetic programming algorithm that solves such problems using a satisfiability modulo theories solver to formally verify the candidate solutions. The essence of the method consists in collecting the counterexamples resulting from model verification and using them to improve search guidance. The method is exact upon successful termination, the produced model is guaranteed to meet the specified constraints. We compare the effectiveness of the proposed method with standard constraint-agnostic machine learning regression algorithms on a range of benchmarks and demonstrate that it outperforms them on several performance indicators.
更多查看译文
关键词
Constraints,genetic programming (GP),satisfiability modulo theories (SMTs),symbolic regression (SR)
AI 理解论文
溯源树
样例
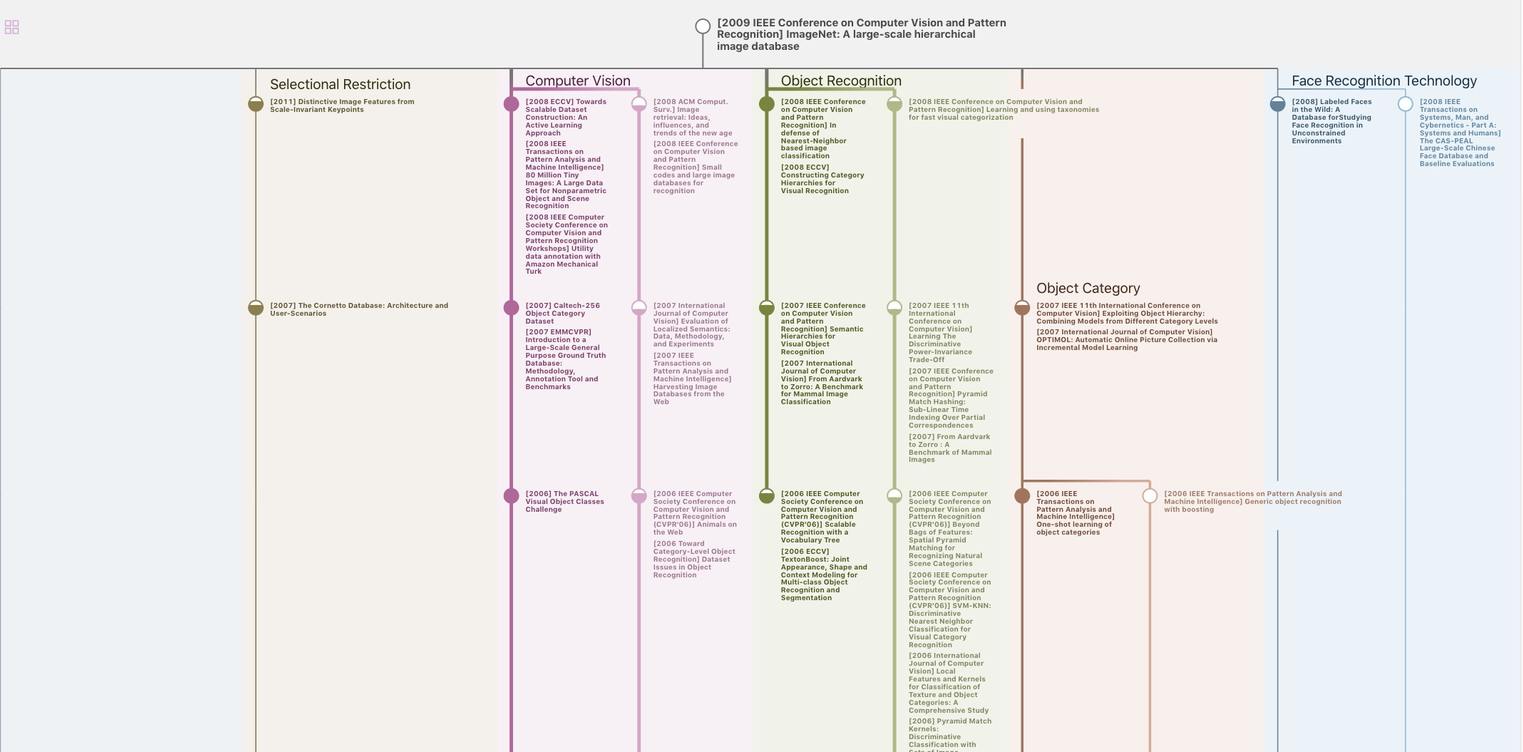
生成溯源树,研究论文发展脉络
Chat Paper
正在生成论文摘要