Machine learning guided 3D printing of carbon microlattices with customized performance for supercapacitive energy storage
Carbon(2023)
摘要
Three-dimensional (3D) printing has stood out as a reliable technology to construct carbon microlattice electrodes for supercapacitors (SCs) in the field of custom areal electrochemical performance needed. The complex structural parameters of 3D-printed (3DP) electrodes make customization of the 3DP electrodes low efficient and time-consuming. Herein, we integrate machine learning (ML) to deeply unravel the influence of typical structural parameters of 3DP electrodes made of graphene and carbon nanotubes (CNTs). The dependence of areal performance on electrode structures was established through selecting only 9 experimental points combined with random forest (RF) algorithm, where the valuable information and the hidden correlations were quickly extracted. By using the as-established model, the electrodes with desired performance could be printed based on structural parameters directly selected from the model, offering an essentially improved performance for target performance. Specifically, the areal performance could be tuned from 0.032 to 1.6 F cm−2, covering an extremely large range. Moreover, the electrochemical surface area (ECSA) and finite element analysis (FEA) were employed to analyze the dependence of areal performance on structural parameters, agreeing well with the model information. The idea proposed in this work could largely increase the efficiency of developing new electrode architectures for desired performance.
更多查看译文
关键词
Machine learning,Random forest,3D printing,Structural parameters
AI 理解论文
溯源树
样例
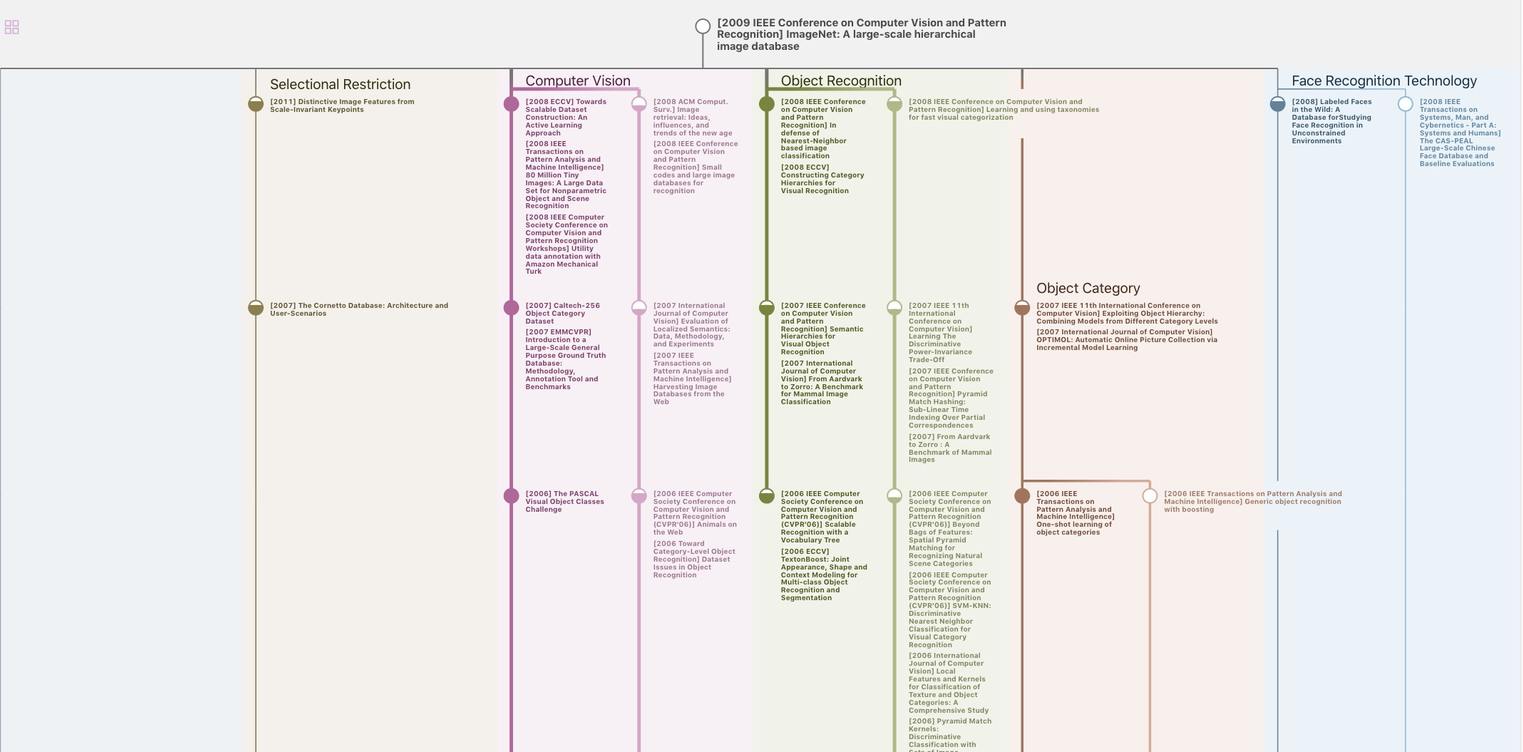
生成溯源树,研究论文发展脉络
Chat Paper
正在生成论文摘要