Image embedding and user multi-preference modeling for data collection sampling
SSRN Electronic Journal(2023)
摘要
This work proposes an end-to-end user-centric sampling method aimed at selecting the images from an image collection that are able to maximize the information perceived by a given user. As main contributions, we first introduce novel metrics that assess the amount of perceived information retained by the user when experiencing a set of images. Given the actual information present in a set of images, which is the volume spanned by the set in the corresponding latent space, we show how to take into account the user’s preferences in such a volume calculation to build a user-centric metric for the perceived information. Finally, we propose a sampling strategy seeking the minimum set of images that maximize the information perceived by a given user. Experiments using the coco dataset show the ability of the proposed approach to accurately integrate user preference while keeping a reasonable diversity in the sampled image set.
更多查看译文
关键词
Perceived information, Representation learning, User preference modeling, ResNeXt, COCO dataset, Image collection sampling
AI 理解论文
溯源树
样例
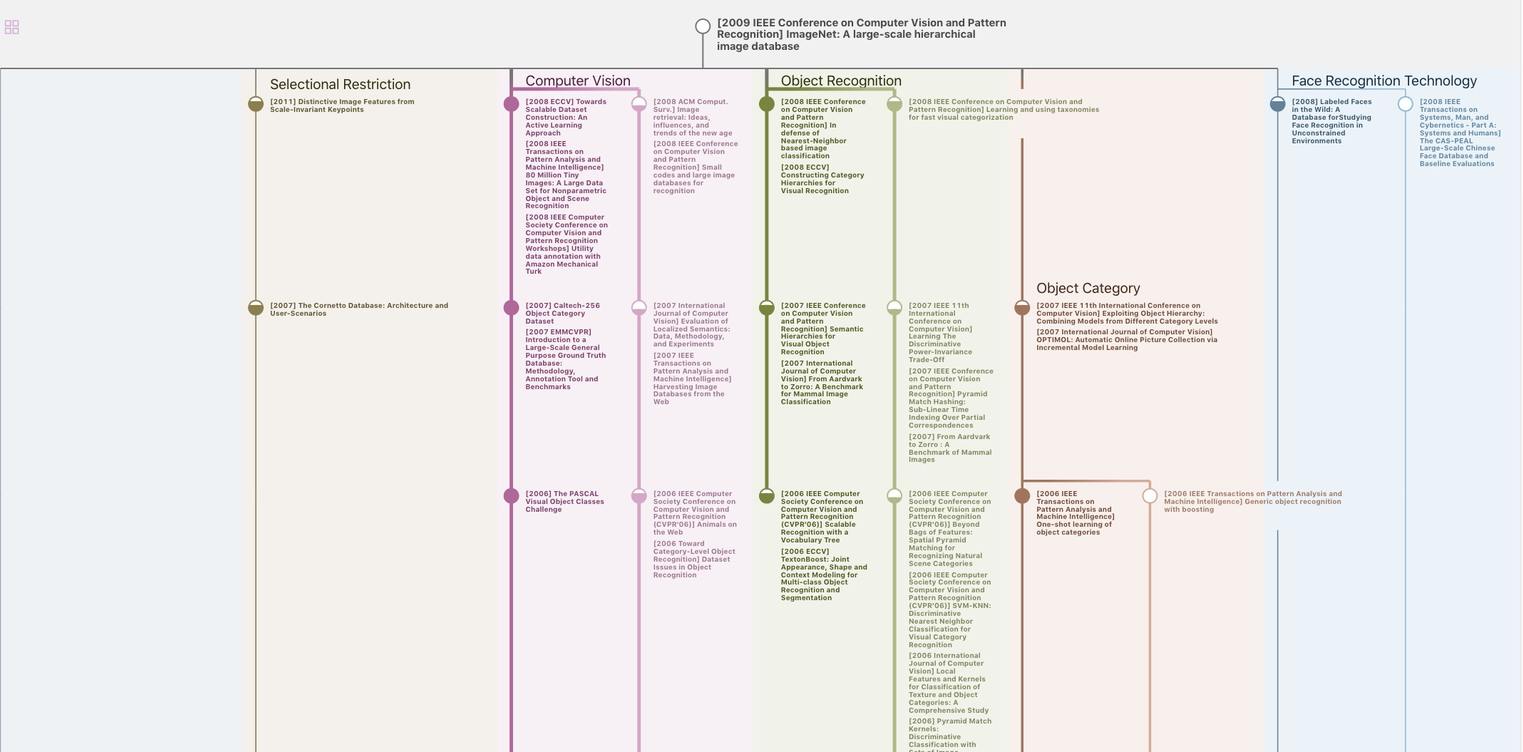
生成溯源树,研究论文发展脉络
Chat Paper
正在生成论文摘要