Effect of state transition triggered by reinforcement learning in evolutionary prisoner’s dilemma game
Neurocomputing(2022)
摘要
Cooperative behavior is essential for conflicts between collective and individual benefits, and evolutionary game theory provides a key framework to solve this problem. Decision-making of human or automata agent occurs not only in a static environment, but also in the dynamic interactive environment. Since the reinforcement learning algorithm is well performed at explaining the problem in regard to state, action, and environment, we propose a game model with individual state transition which is influenced by the self-regarding Q-learning algorithm. In detail, we at the first time investigate a two-state two-action game, where agents can choose either to participate in the network game (i.e., active agent) or to cut off all the links based on its Q-table (i.e., inactive agent), involving in a dynamic interactive environment. Through numerical simulations, it is shown that cooperation can reach the maximal level in the moderate value space of fixed reward obtained by inactive agents. In particular, long-term expectations and large learning rates are more productive in promoting cooperation. Furthermore, when the dynamic interactive environment reaches a stable state, the number of active neighbors of active cooperators is larger than that of active defectors, which is further larger than the number of active neighbors of inactive agents. Finally, we testify the results of theoretical analysis from the perspective of state transition.
更多查看译文
关键词
Social dilemma,Q-learning,State transition,Action selection,Cooperation
AI 理解论文
溯源树
样例
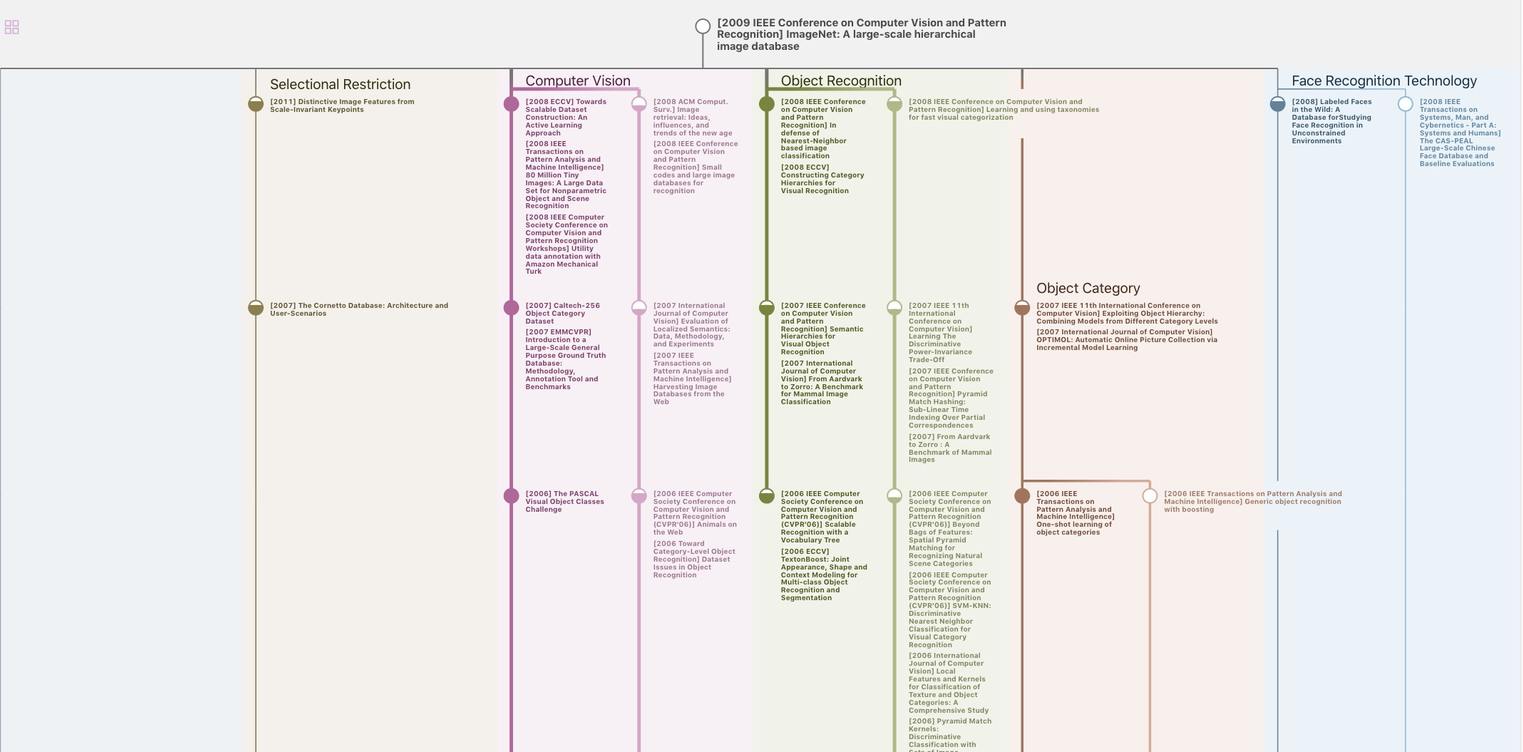
生成溯源树,研究论文发展脉络
Chat Paper
正在生成论文摘要