GASN: A Graph Attention Stochastic Network against Adversarial Attack
2022 8th International Conference on Big Data and Information Analytics (BigDIA)(2022)
摘要
In recent years, graph neural networks (GNNs) has shown its unique advantages in graph data research and analysis because it considers the attributes of graph nodes and topology information at the same time. However, graph neural networks is as vulnerable to counter attack as traditional deep learning, and the performance of the model can be seriously degraded by adding small disturbances. At present, researchers have proposed various methods to improve the robustness of graph neural network model for confrontation and defense, but the existing working methods are too complex and make great changes to the model, which can only be applied to specific graph neural network models. To solve the above problems, we propose a graph attention stochastic neural network defense method, which uses multivariate Gaussian distribution to replace the node vector representation in the hidden layer of graph neural network, uses the variance of Gaussian distribution to absorb the impact of attack, and adds a mean based attention mechanism, that is, when convolution aggregation is carried out, by measuring the difference of the mean value of multivariate Gaussian distribution, the weight is allocated to further reduce the impact of attack on the model. Experiments on three common datasets show that the model proposed in this paper shows excellent performance in resisting counter attacks.
更多查看译文
关键词
robustness,adversarial attack,stochastic neural network,attention mechanism,graph neural network
AI 理解论文
溯源树
样例
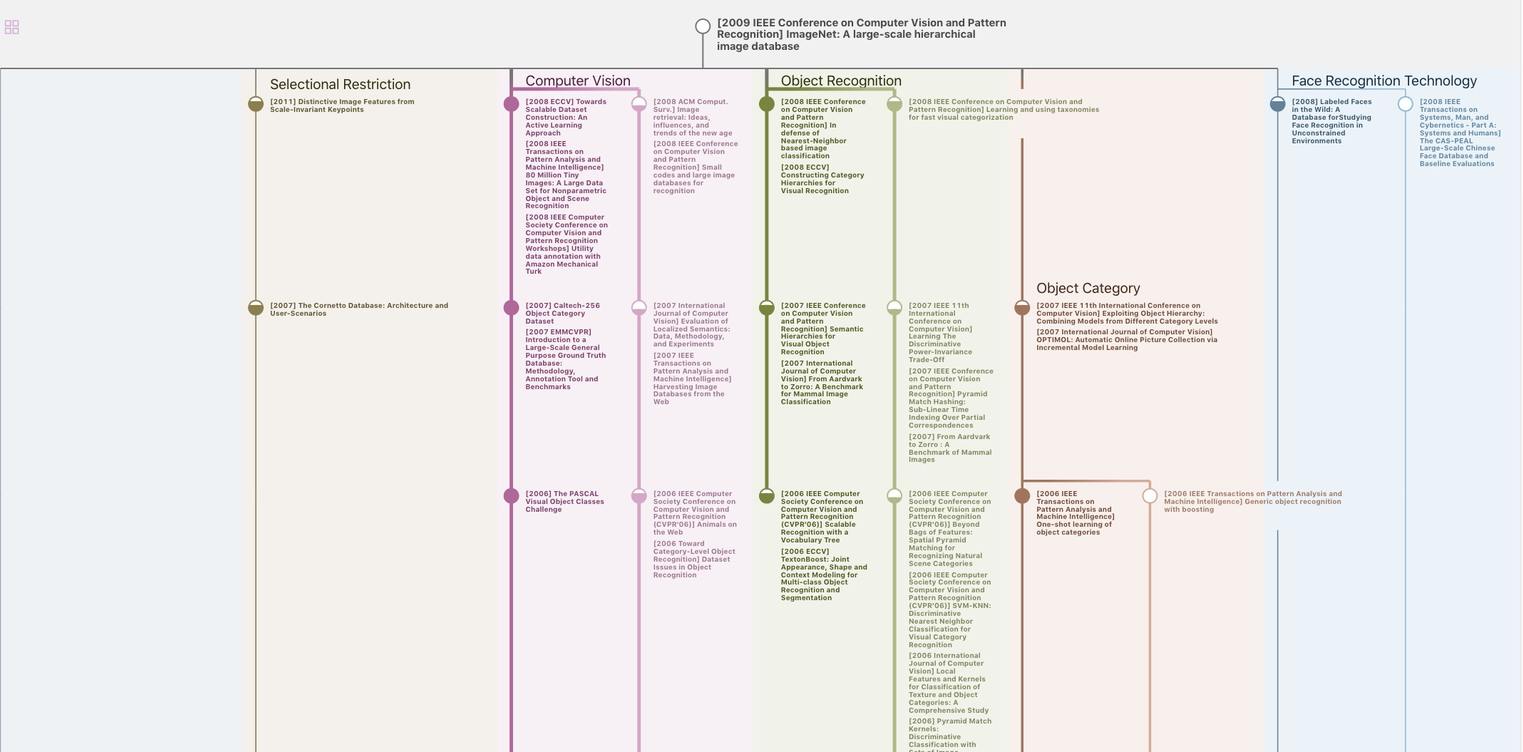
生成溯源树,研究论文发展脉络
Chat Paper
正在生成论文摘要