An AE-LSTM Based Diagnosis Method of Wind Turbine Blade Icing
2022 8th International Conference on Big Data and Information Analytics (BigDIA)(2022)
摘要
Blade icing, a typical fault of wind turbines, is one of the main reasons for impacting the operation and production efficiency of wind turbines. Therefore, it is of great significance to implement the diagnosis of this fault. Considering that the Auto-Encoder (AE) performs well in extracting data features, and the Long Short-Term Memory network (LSTM) excels at processing time series data, a diagnosis method based on AE-LSTM is proposed, in which the AE is used to learn normal data and the reconstruction error is considered as spatial information, while the LSTM is utilized to learn trends of time series data and the predicted fault probability is regarded as temporal information. A diagnosis threshold can be calculated to judge the operation state by further fusing spatial and temporal information into a composite index. The proposed method is applied to real operation data of a wind turbine for blade icing fault diagnosis, and the AE-LSTM method is compared with seven other approaches on three evaluation criteria. The proposed method can not only obtain superior diagnosis performance by fusing spatial and temporal information, but also mine the deep characteristics of faults through its composite index. Different from conventional diagnosis approaches that only predict the status label, the proposed method applies a numerical composite index to reflect operation status. By analyzing trend characteristics of composite index, diagnosis result correction, fault period identification and high-fault period warning can be further realized, which provides powerful support for in-depth work.
更多查看译文
关键词
wind turbines,blade icing,fault diagnosis,AE,LSTM
AI 理解论文
溯源树
样例
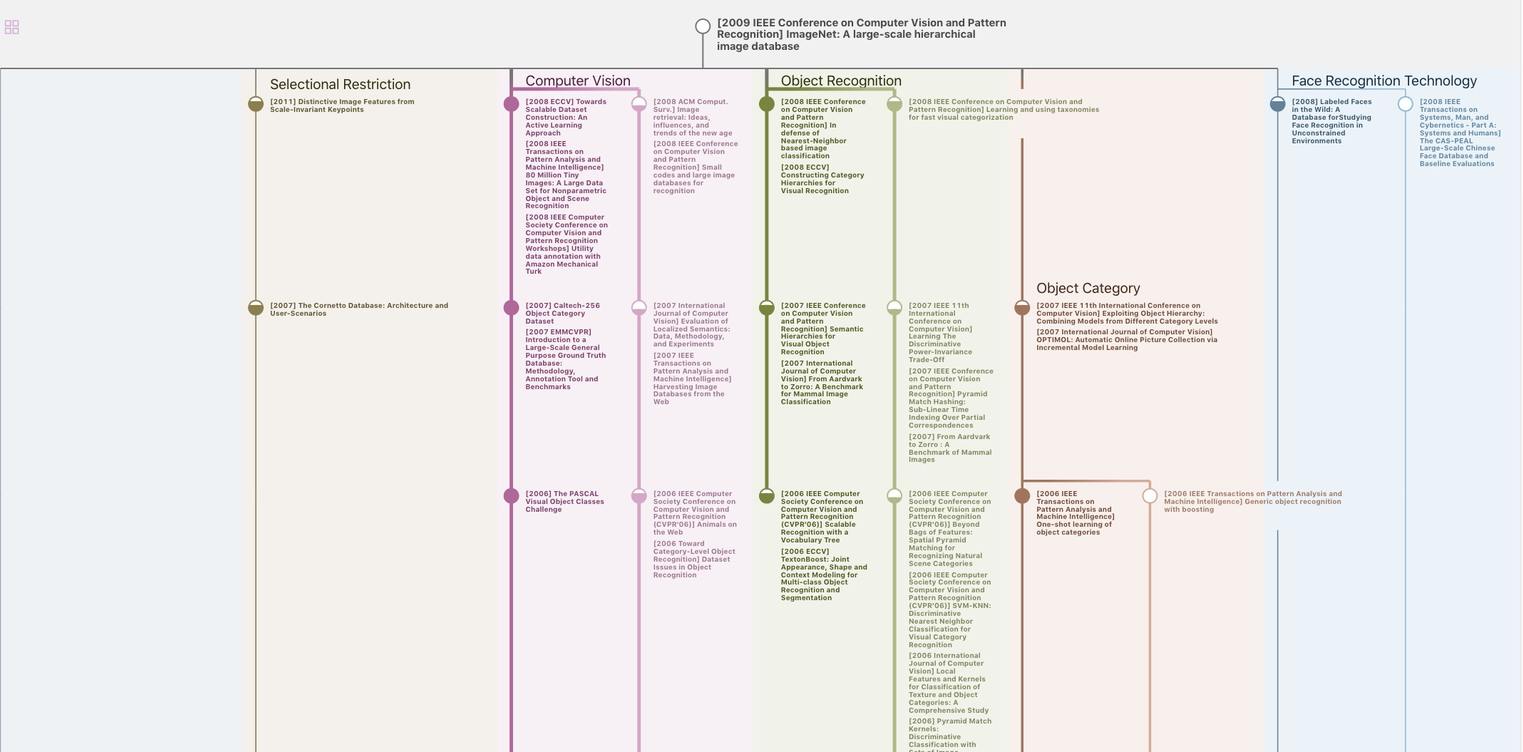
生成溯源树,研究论文发展脉络
Chat Paper
正在生成论文摘要