SURE-based Stopping Strategy for Fine-tunable Supervised PET Image Denoising
2021 IEEE Nuclear Science Symposium and Medical Imaging Conference (NSS/MIC)(2021)
摘要
Previously, based on the unsupervised deep learning method, conditional deep image prior (CDIP), we showed the possibility of finetuning during the testing phase after supervised learning. However, one barrier for CDIP finetuning is that it needs early stopping before the network output converges to the noise which is hard to determine. In this work, we proposed to construct the finetuning loss based on Stein’s unbiased risk estimator (SURE), which can provide an unbiased estimation of the true mean square error (MSE). We demonstrated that using SURE-based finetuning loss, the network output can be close to the ground truth while not converging to the noise. Quantification results based on seven real patient datasets show that the proposed method is more stable during finetuning than CDIP.
更多查看译文
关键词
pet,stopping strategy,sure-based,fine-tunable
AI 理解论文
溯源树
样例
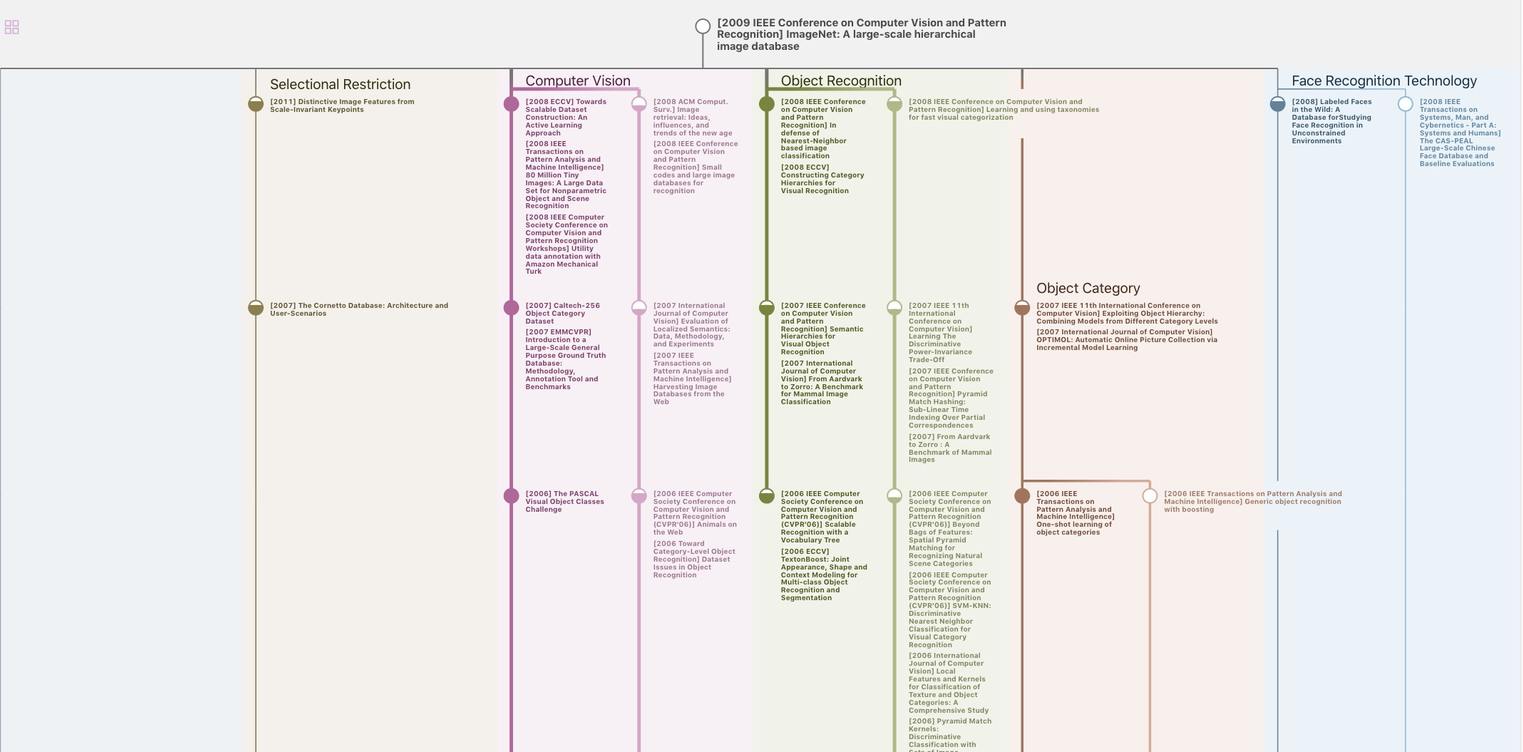
生成溯源树,研究论文发展脉络
Chat Paper
正在生成论文摘要