Thermal conductivity prediction of UO2-BeO composite fuels and related decisive features discovery via convolutional neural network
Acta Materialia(2022)
摘要
Improved thermal conductivity (TC) in UO2-based fuels is critically important for the security of nuclear reactors. Optimizing the microstructure of UO2 pellets mixed with high thermally conducting materials such as BeO is an effective way to achieve this goal. In this paper, we investigate the relationship between the TC of the UO2/BeO composite and their corresponding microstructures by using a convolutional neural network (CNN). The dataset is created by the finite element method. Using the deep transfer learning technique, a well-established CNN (ResNet101) successfully predicts the TC based on the highly similar microstructures while the lightweight CNN fails. Meanwhile, the microstructural features impacting the TC of the UO2/BeO composite are determined by the ablation class activation map and subsequently verified. Moreover, a proposed microstructural feature contributes to the improved performance of the state-of-the-art machine learning model on our task, indicating its essential role in the TC of the UO2/BeO composite. This work demonstrates that the critical microstructural features that significantly affect the TC can be discovered by deep learning-based methods and may provide a guideline for enhanced TC of UO2/BeO fuel via careful design of its microstructure.
更多查看译文
关键词
Structure-property correlation,Deep transfer learning,UO2/BeO composite fuels,Thermal conductivity,Microsctructural features
AI 理解论文
溯源树
样例
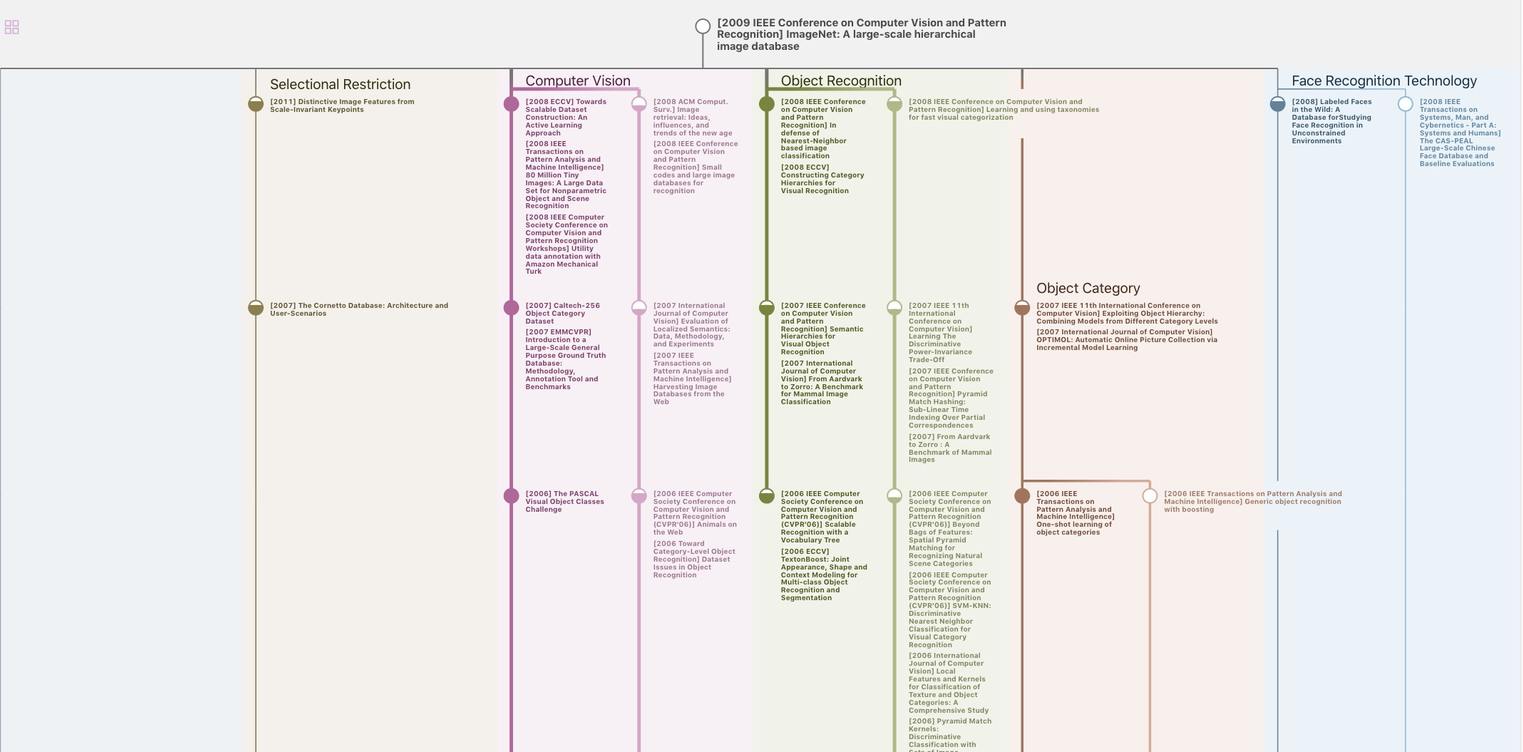
生成溯源树,研究论文发展脉络
Chat Paper
正在生成论文摘要