An End-to-End Convolutional Network for Estimating the Essential Matrixan End-to-End Convolutional Network for Estimating the Essential Matrix
SSRN Electronic Journal(2022)
摘要
Essential matrix (E-matrix) estimation is a crucial aspect of pose estimation. In this study, we developed an end-to-end method (E-net) to estimate the E-matrix without correspondences. A pair of the corresponding images was placed in the twin transformer architecture to simultaneously extract the features. We developed a feature matching module for matching the extracted features based on their commonalities. To avoid excessive network parameters, matched features with their weights obtained by multilayer perceptron were transmitted to the flatten layer, where the Max-Pooling was used to eliminate their useless portions. We further constructed three self-defined layers to ensure that E-matrix is rank-2 with 5 degrees of freedom using reserved helpful features. Besides, we presented two self-defined loss functions (Loss1 and Loss2) to train the E-net and improve the estimated E-matrix's accuracy. E-net's performance was evaluated on the KITTI and TUM SLAM datasets using two self-defined metrics, M1 (mean value of matching error) and M2 (mean squared value of matching error). The E-net achieved M1 0.107 and M2 0.091 on the KITTI dataset and M1 0.253 and M2 0.144 on the TUM SLAM dataset. The results demonstrated that the E-net trained with self-defined loss functions outperforms other algorithms when compared to the 5-point algorithm of M1 10.411 and M2 8.332.
更多查看译文
关键词
E-matrix,Estimation layer,Guarantee layer,Self-defined loss function
AI 理解论文
溯源树
样例
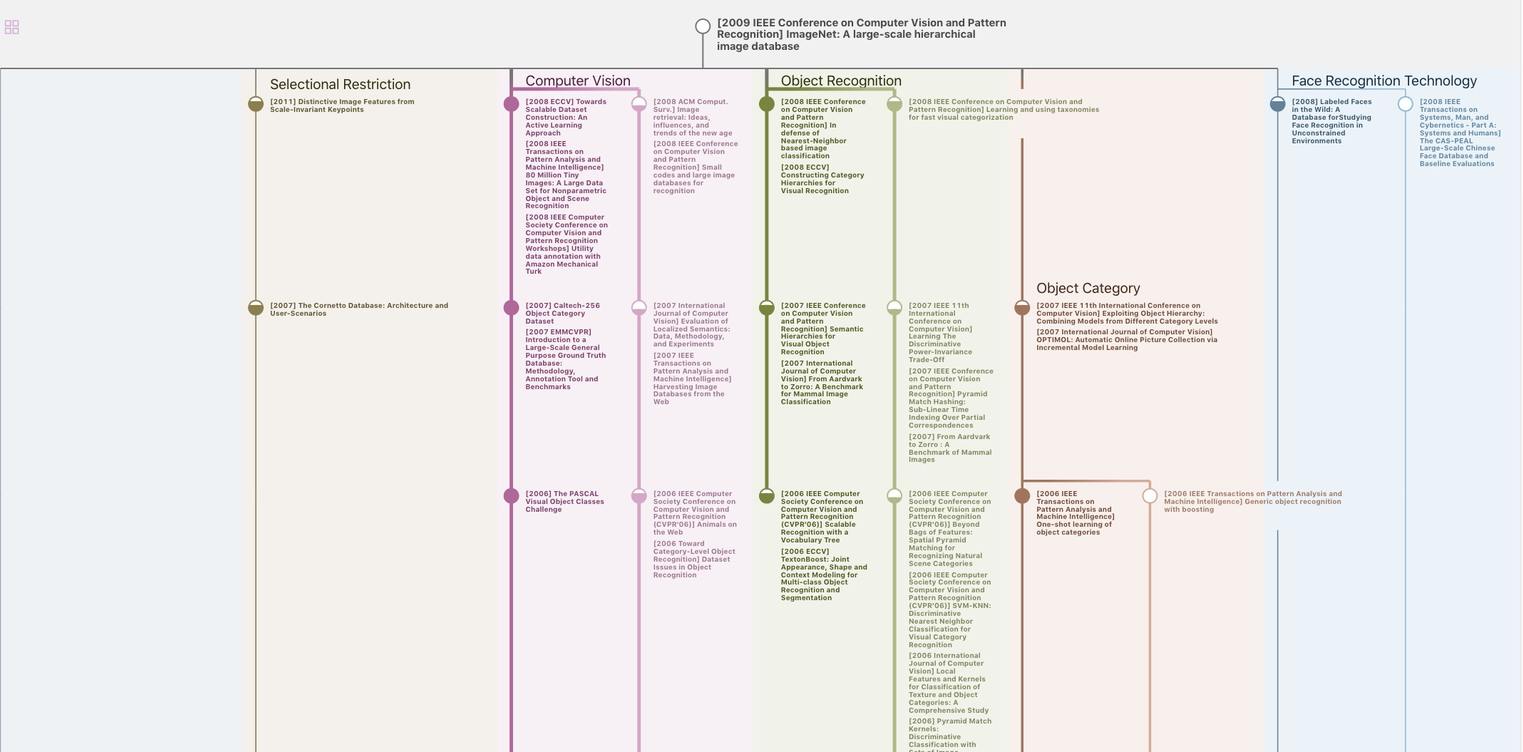
生成溯源树,研究论文发展脉络
Chat Paper
正在生成论文摘要