Stochastic Resonance Governs Memory Consolidation Accuracy in a Neural Network Model.
Annual International Conference of the IEEE Engineering in Medicine and Biology Society (EMBC)(2022)
摘要
The formation and recollection of memories is a multi-step neural process subject to errors. We propose a computational model of memory nodes receiving input from a colored tic-tac-toe board. We report memory errors during consolidation and reconsolidation when different noise levels are introduced into the model. The model is based on Hebbian plasticity and attempts to store the color and position of an X or O from the board. Memory nodes simulating neurons use an integrate-and-fire model to represent the correct or incorrect storage of the board information by scaling synaptic weights. We explored how baseline firing rate, which we considered analogous to noise in storing memory, impacted the creation of correct and incorrect memories. We found that a higher firing rate was associated with fewer accurate memories. Interestingly, the ideal amount of noise for correct memory storage was nonzero. This phenomenon is known as stochastic resonance, wherein random noise enhances processing. We also examined how many times our model could reactivate a memory before making an error. We found an exponentially decaying response, with a low firing rate yielding more stable memories. Even though our model incorporates only two memory nodes, it provides a basis for examining the consolidation and retrieval of memory storage based on the unique visual input of a tic-tac-toe board. Further work may incorporate different inputs, more nodes, and increased network complexity. Clinical Relevance- This model enables investigation of how the human cortex may utilize and exploit noise during information processing.
更多查看译文
关键词
memory,consolidation,resonance,neural network
AI 理解论文
溯源树
样例
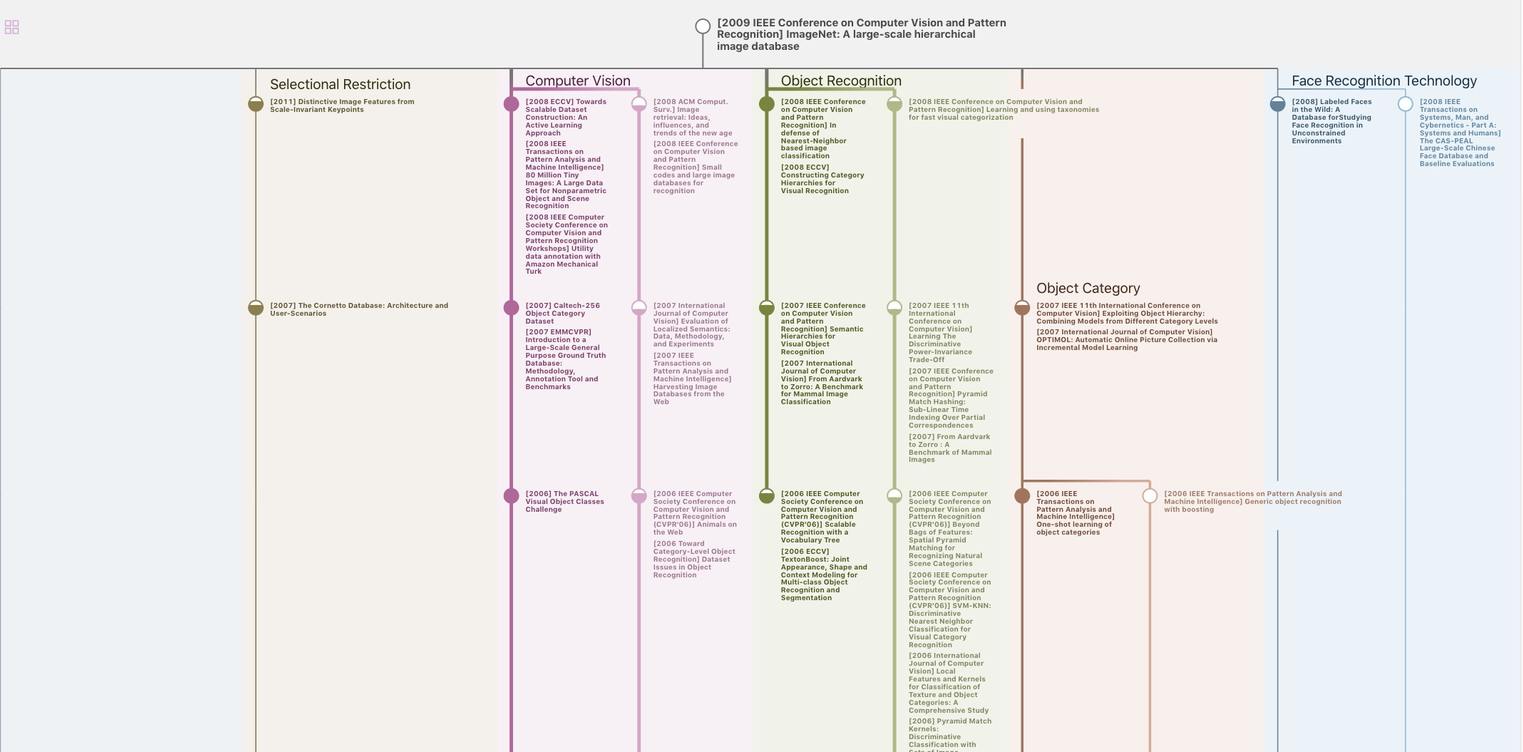
生成溯源树,研究论文发展脉络
Chat Paper
正在生成论文摘要