A Riemannian Deep Learning Representation to Describe Gait Parkinsonian Locomotor Patterns.
Annual International Conference of the IEEE Engineering in Medicine and Biology Society (EMBC)(2022)
摘要
Parkinson is the second most common neurodegenerative disease, mainly related to progressive locomotor alterations caused by dopamine deficiency. The gait kinematic is a principal disease biomarker that associates patterns like the step length, flexed posture, and bradykinesia with disease progression. Nonetheless, these patterns are analyzed from invasive setups that only capture coarse dynamics at advanced disease stages. This work introduces a very compact and robust deep representation that effectively learns a Riemannian manifold to describe locomotor parkinsonian patterns. Contrary to traditional deep strategies, the presented framework fully explores data geometry from input mean covariance matrices representing video sequences. These symmetric positive definite (SPD) matrices lie in a Riemannian manifold. Then such matrices are projected to the SPD net, which learns a bank of SPD matrices from a non-linear training, that may be exploited in a hierarchical composition from a set of layers. In a final layer, a projection in a Euclidean space allows learning the discriminatory patterns of PD w.r.t control population. In a retrospective study with a total of 22 patients (11 Parkinson's and 11 controls), the proposed approach achieves a remarkable classification between Parkinson's and control video sequences, correctly labeling all Parkinson's patients, and outperforming typical 3D convolutional representations. Clinical relevance- The study of gait parkinsonian descrip-tors computed from a Riemannian geometry, built from a deep representation.
更多查看译文
关键词
riemannian deep learning representation,deep learning,gait
AI 理解论文
溯源树
样例
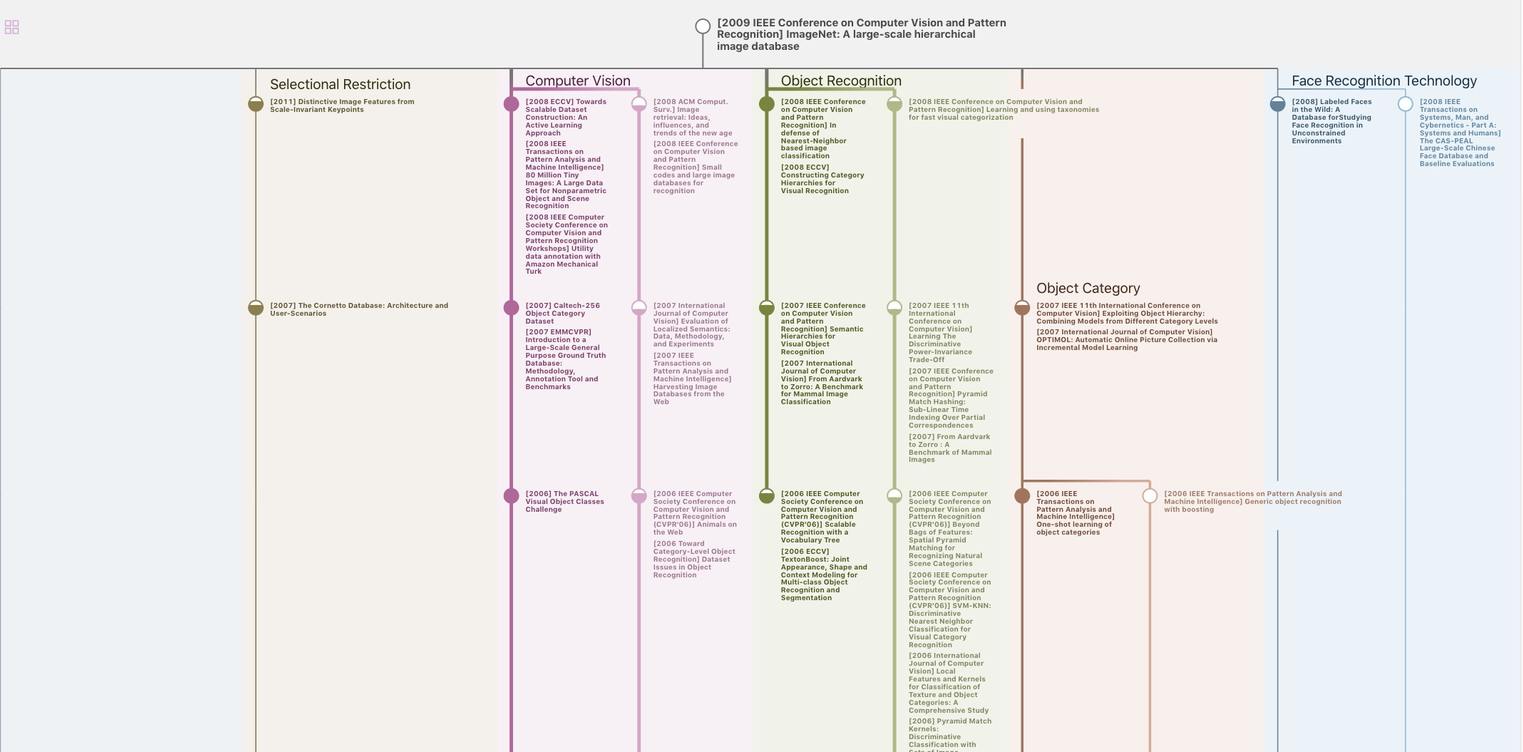
生成溯源树,研究论文发展脉络
Chat Paper
正在生成论文摘要