Effective Data Augmentation, Filters, and Automation Techniques for Automatic 12-Lead ECG Classification Using Deep Residual Neural Networks.
Annual International Conference of the IEEE Engineering in Medicine and Biology Society (EMBC)(2022)
摘要
Automatic electrocardiogram (ECG) analysis plays a critical role in early detection and diagnosis of cardiac abnormalities and diseases. Data augmentation and automation strategies have been proposed to enhance the robustness of the machine and deep learning model for the classification of cardiac abnormalities. Here we propose 15 data augmentation and 6 filters, and an automation method using an end-to-end deep residual neural network (ResNet) model for automatic cardiac abnormalities detection from 12-lead ECG recordings. We evaluate the effectiveness of data augmentation/filtering and automation techniques using the proposed ResNet-based model on the China Physiological Signal Challenge (CPSC) dataset consisting of 9 diagnostic classes. The average F1 scores across 9 classes on the CPSC dataset trained with three data augmentation (baseline wander addition, dropout, and scaling) and a filter (sigmoid compression) were significantly higher than that without using augmentation/filters (baseline). The highest average F1 score with sigmoid compression method was significantly higher (relative improvement of 2.04 %) than the baseline while horizontal and vertical flipping augmentations were detrimental to the classification performance. Additionally, the results show that the random combination of four selected data augmentation and filter using the modified RandAugment technique provided a significantly higher average F1 score (relative improvement of 2.54 %) compared to the baseline. The proposed data augmentation, filters, and automation techniques provide an effective solution to improve the classification performance of the end-to-end deep learning model from ECG recordings without changing the model hyperparameters and structure.
更多查看译文
关键词
ecg,effective data augmentation,deep residual neural networks,neural networks
AI 理解论文
溯源树
样例
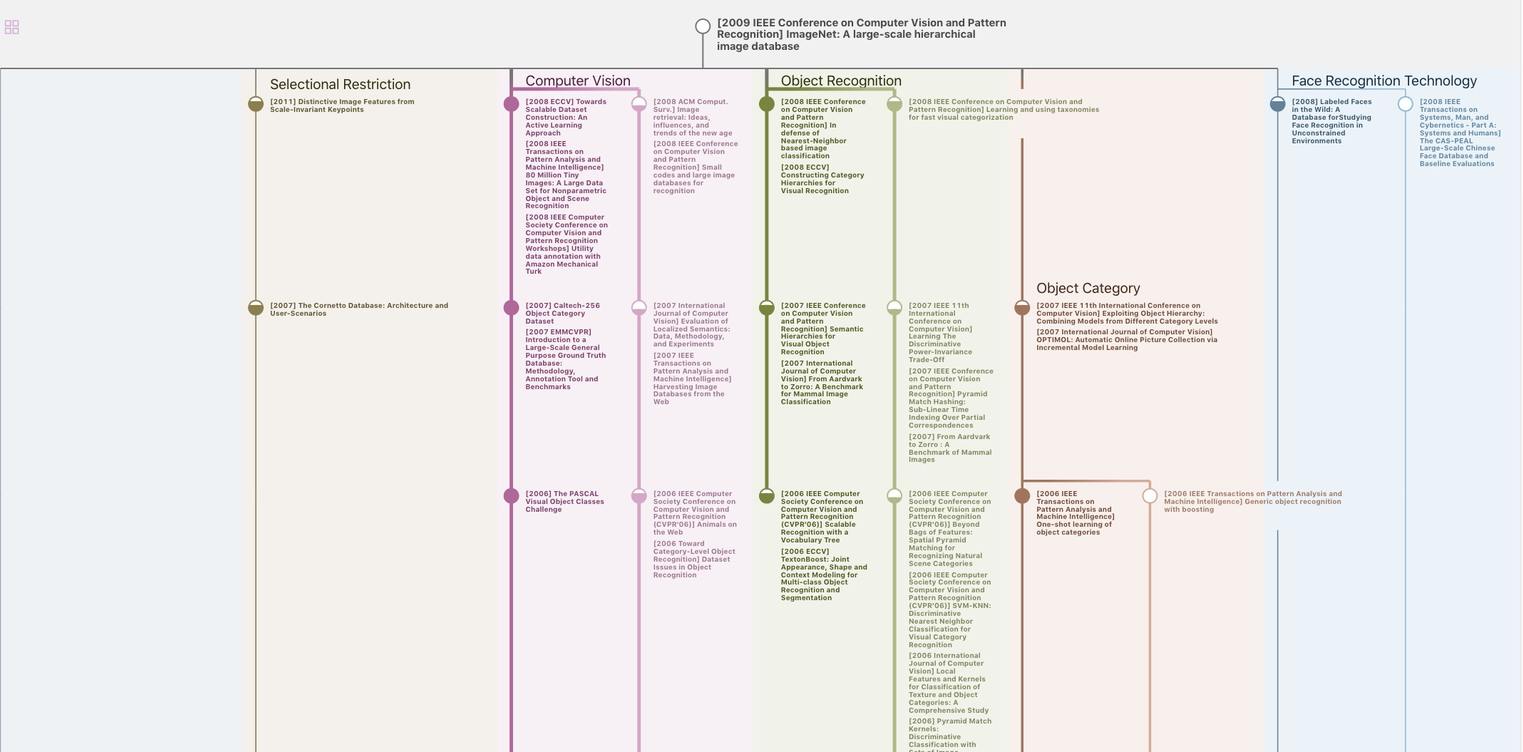
生成溯源树,研究论文发展脉络
Chat Paper
正在生成论文摘要