AEPUS: a Tool for the Automated Extraction of Pennation Angles in Ultrasound Images with Low Signal-to-noise Ratio for Plane-Wave Imaging
2022 44th Annual International Conference of the IEEE Engineering in Medicine & Biology Society (EMBC)(2022)
摘要
The penetrating ability of ultrasound (US) com-bined with its real-time operation make it the perfect tool for investigating muscle contraction mechanics during complex functional tasks, e.g., locomotion. Changes in fascicle lengths and pennation angles of muscle fascicles strongly correlate with the capacity of skeletal muscles to produce forces, thereby represent fundamental parameters to be tracked. While the gold standard for extracting these features from US images is still based on manual annotation, the availability of recording devices capable of generating big data of muscle dynamics makes such manual approach unfeasible, setting the need for automated muscle images annotation tools. Existing approaches, however, are seriously limited, also in view of the continuous developments and technology ad-vancements for ultrafast US and plane-wave imaging. In fact, they rely on conventional (slow) B-mode imaging, make use of point tracking approaches (which often fail due to out-of-plane motion), or can only operate on very high quality images. To overcome all these limitations, we present AEPUS, an automated image labeling tool capable of extracting pennation angles from low quality images using a very small number of plane waves, therefore making it capable of exploiting all the benefits of ultrafast US. Clinical Relevance - Ultrasound is a standard research tool to investigate alterations of spastic muscles in children with Cerebral Palsy. We propose a reliable and time-efficient method to track muscle features in ultrasound images and support clinical biomechanists in their analyses.
更多查看译文
关键词
Child,Humans,Muscle Contraction,Muscle, Skeletal,Signal-To-Noise Ratio,Ultrasonography
AI 理解论文
溯源树
样例
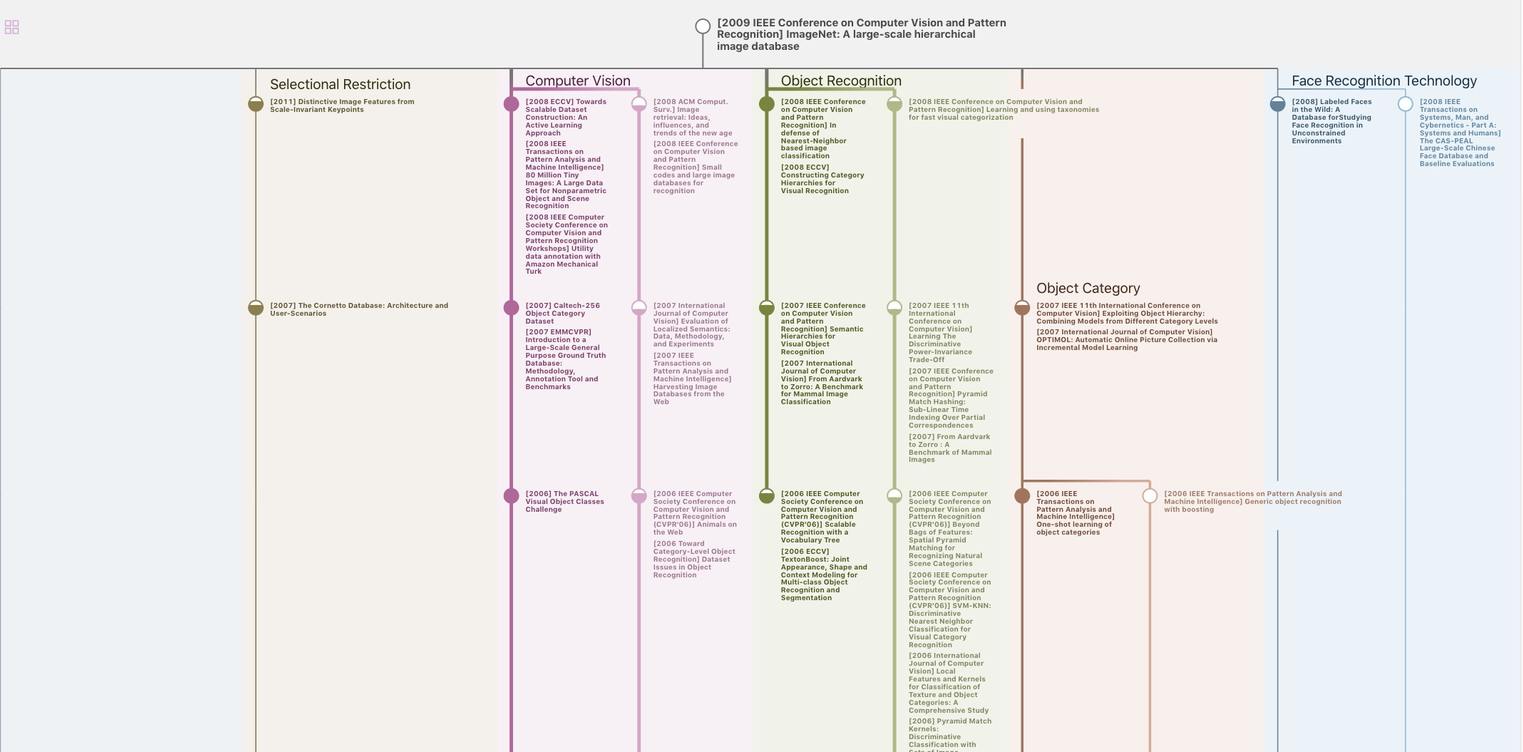
生成溯源树,研究论文发展脉络
Chat Paper
正在生成论文摘要