The Effectiveness of Narrowing the Window size for LD & HD EMG Channels based on Novel Deep Learning Wavelet Scattering Transform Feature Extraction Approach.
Annual International Conference of the IEEE Engineering in Medicine and Biology Society (EMBC)(2022)
摘要
The use of the Electromyogram (EMG) signals as a source of control to command externally powered prostheses is often challenged by the signal complexity and non-stationary behavior. Mainly, two factors affect classification accuracy: selecting the optimum feature extraction methods and overlapping segmentation/window size. Nowadays, studies attempt to use deep learning (DL) methods to improve classification accuracy. However, DL models are frequently hampered by their requirements of a vast quantity of training data to attain decent performance and the high computing costs. Therefore, researchers tried to replace the deep learning models with other low computational cost methods like deep wavelet scattering transform (DWST) as a feature extraction technique. In terms of windows size, selecting a larger window size increases the classification accuracy, but at the same time, it increases the processing time, which makes the system unsuitable for real-time applications. Accordingly, researchers attempted to minimise the size of the overlapping windows as much as possible without impacting classification performance. This work suggests to utilise DWST transform to achieve two goals (a) extracting the features from EMG signal with low computational cost. Even though many studies have used DWST approaches to extract features from other biological signals, but not been examined before for EMG signals. (b) study the effect of extracting the features from high-density EMG datasets (HD EMG) and low-density EMG datasets (LD EMG), reducing the analysis window size by up to 32msec with minimal impact on classification performance. The outcomes of the proposed method are compared with other well-known feature extraction algorithms to validate these achievements. The proposed strategy exceeds other methods by more than 25% in accuracy.
更多查看译文
关键词
hd emg channels,feature extraction,deep learning
AI 理解论文
溯源树
样例
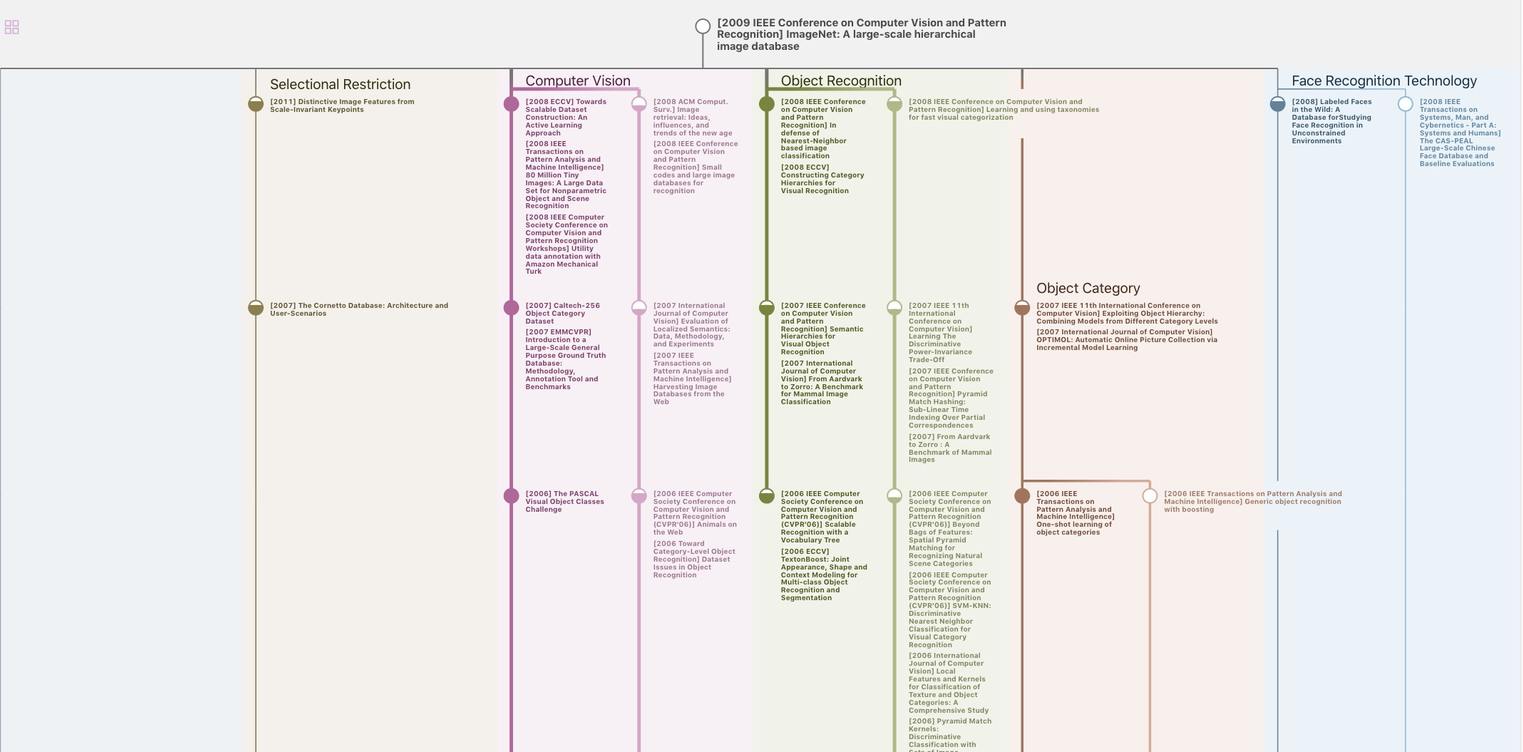
生成溯源树,研究论文发展脉络
Chat Paper
正在生成论文摘要