Predicting diversity changes in subalpine moorland ecosystems based on geometry of species distributions and realistic area loss
JOURNAL OF VEGETATION SCIENCE(2022)
摘要
Question How does plant species richness respond to simulated area loss based on the realistic geometry of area loss in subalpine moorland ecosystems? Location Hakkoda mountain range, Aomori, Japan. Methods We constructed species distribution models based on relationships between species distributions and environmental conditions in subalpine moorland ecosystems. We then simulated moorland area loss based on the realistic geometry of area loss from the past (1967) to the present (2019) to predict future changes in plant diversity. Here, we defined the realistic geometry of area loss as the plausible spatial pattern of future habitat loss. Finally, we analyzed how the rate of species loss in response to the realistic area loss can be explained by a range of factors including spatial patterns in species distributions, total number of species present, and environmental variables for the focal moorland. Results Within each moorland site, areas prone or those less prone to be lost were distributed non-randomly at a local scale. In general, the patterns of species loss caused by the realistic area loss differed from those caused by random area loss. At most sites, realistic area loss caused a relatively small decline in species richness up to a certain threshold of area loss, and accelerating decline thereafter. None of the factors can explain the rate of decrease in species richness caused by the realistic area loss. At the species level, however, species with lower occurrence rates at a given site can be lost earlier than those with higher occurrence rates by the realistic area loss. Conclusions Patterns of habitat loss and species distributions are not spatially random, and the classical approach based on the species-area relationship assuming random area loss can thus either under- or overestimate the risk of species loss.
更多查看译文
关键词
climate change,generalized additive model,global warming,historical landscape,kriging,random forest
AI 理解论文
溯源树
样例
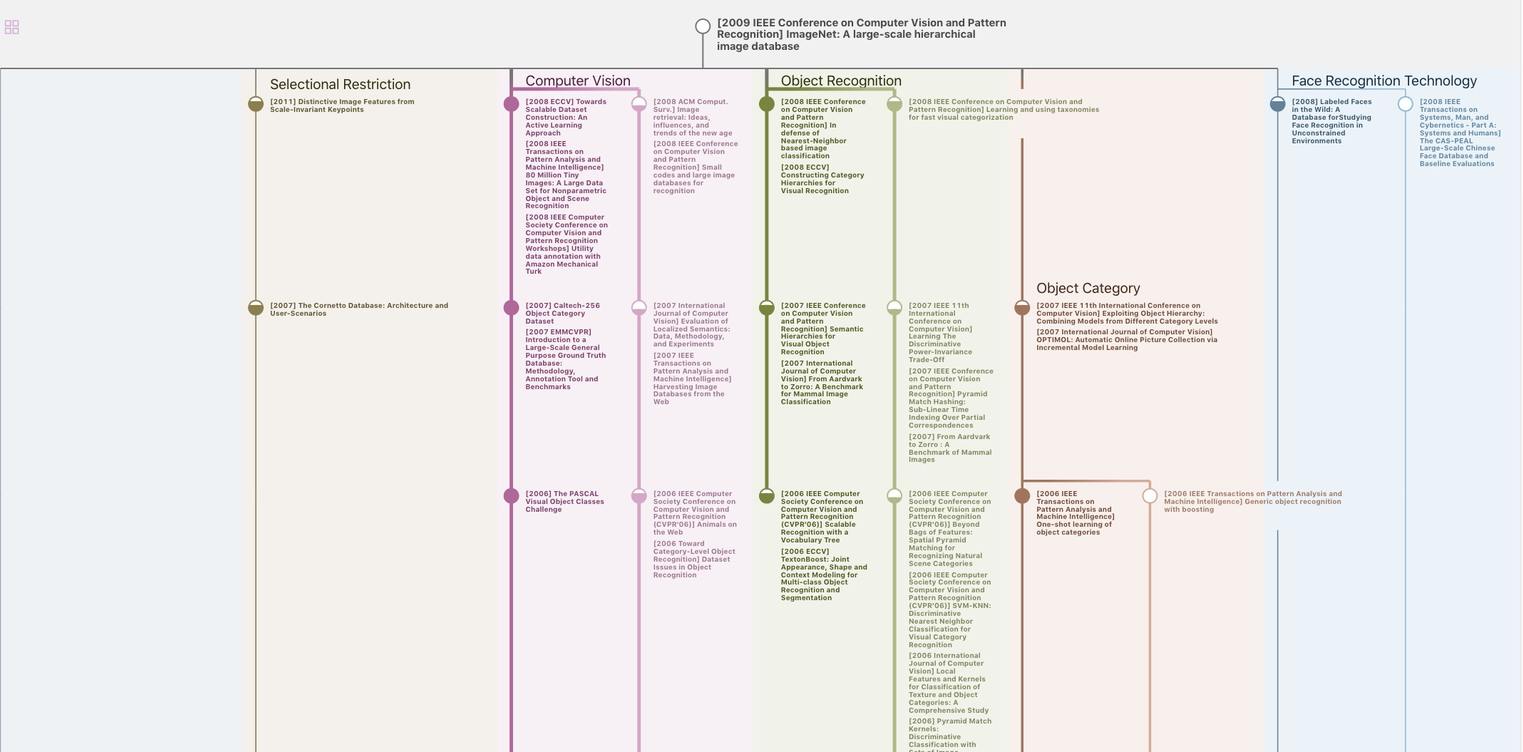
生成溯源树,研究论文发展脉络
Chat Paper
正在生成论文摘要