PrMFTP: Multi-functional therapeutic peptides prediction based on multi-head self-attention mechanism and class weight optimization.
PLOS COMPUTATIONAL BIOLOGY(2022)
摘要
Prediction of therapeutic peptide is a significant step for the discovery of promising therapeutic drugs. Most of the existing studies have focused on the mono-functional therapeutic peptide prediction. However, the number of multi-functional therapeutic peptides (MFTP) is growing rapidly, which requires new computational schemes to be proposed to facilitate MFTP discovery. In this study, based on multi-head self-attention mechanism and class weight optimization algorithm, we propose a novel model called PrMFTP for MFTP prediction. PrMFTP exploits multi-scale convolutional neural network, bi-directional long short-term memory, and multi-head self-attention mechanisms to fully extract and learn informative features of peptide sequence to predict MFTP. In addition, we design a class weight optimization scheme to address the problem of label imbalanced data. Comprehensive evaluation demonstrate that PrMFTP is superior to other state-of-the-art computational methods for predicting MFTP. We provide a user-friendly web server of PrMFTP, which is available at http://bioinfo.ahu.edu.cn/PrMFTP.
更多查看译文
关键词
peptides,multi-functional,multi-head,self-attention
AI 理解论文
溯源树
样例
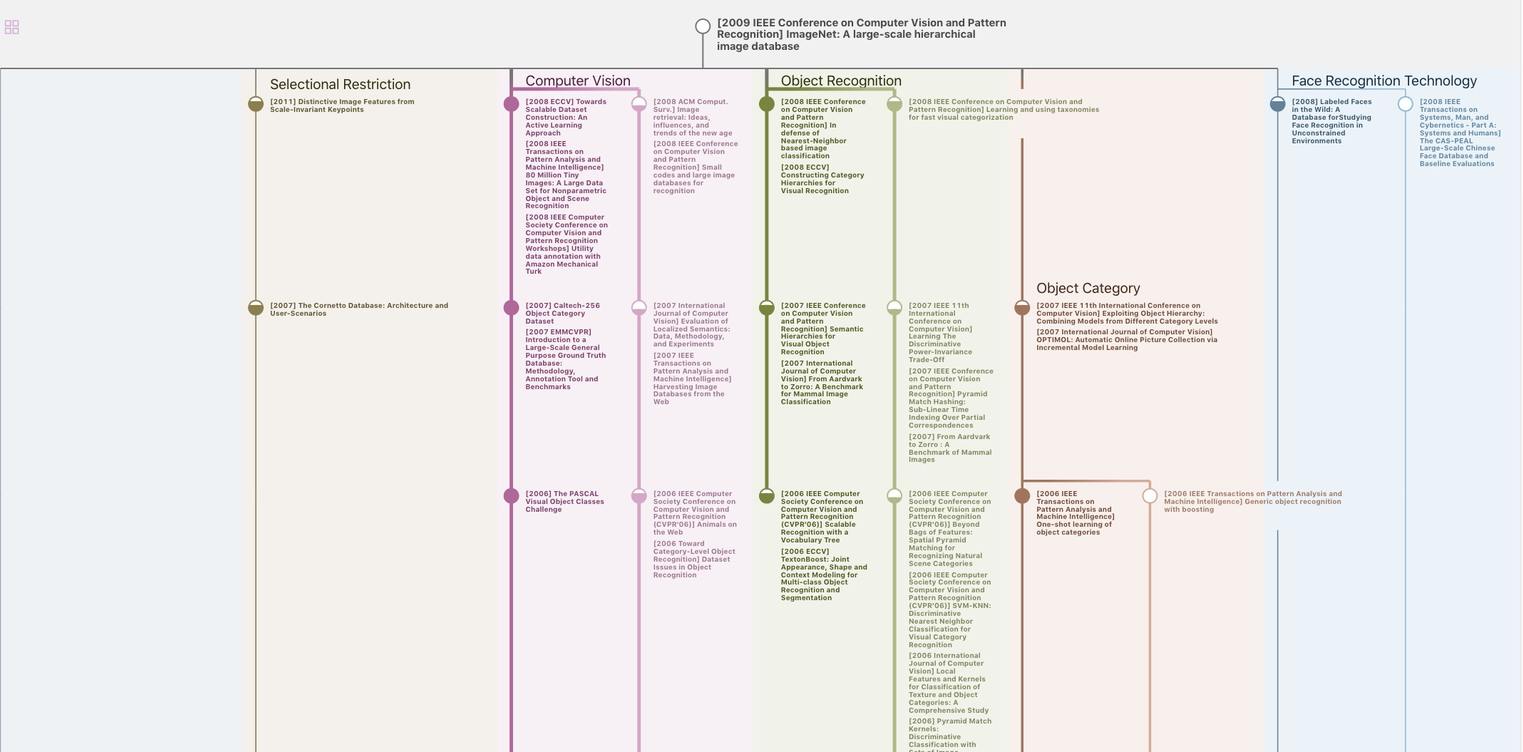
生成溯源树,研究论文发展脉络
Chat Paper
正在生成论文摘要