A Robust Learning Method for Low-Frequency Extrapolation in GPR Full Waveform Inversion
IEEE GEOSCIENCE AND REMOTE SENSING LETTERS(2022)
摘要
Full-waveform inversion (FWI) plays a significant role in producing high-resolution subsurface imaging in seismic prospecting and ground penetrating radar (GPR). However, FWI faces various challenges in practice. For example, the lack of low-frequency information due to acquisition limitations will make the FWI prone to falling to the local minimum. In this project, a deep learning-based approach is proposed to extrapolate the low-frequency data. Specifically, we propose a robust progressive learning (RPL) algorithm that combines physics-guided FWI and data-driven deep learning technology. The proposed method is robust against the choice of the initial model. Experimental results show that our method can achieve high efficiency and accuracy by using a limited amount of training data. The subsurface structures are successfully reconstructed with our extrapolated low-frequency data.
更多查看译文
关键词
Training,Data models,Training data,Predictive models,Extrapolation,Testing,Image reconstruction,Bandwidth extension (BWE),full waveform inversion (FWI),ground penetrating radar (GPR),low-frequency extrapolation
AI 理解论文
溯源树
样例
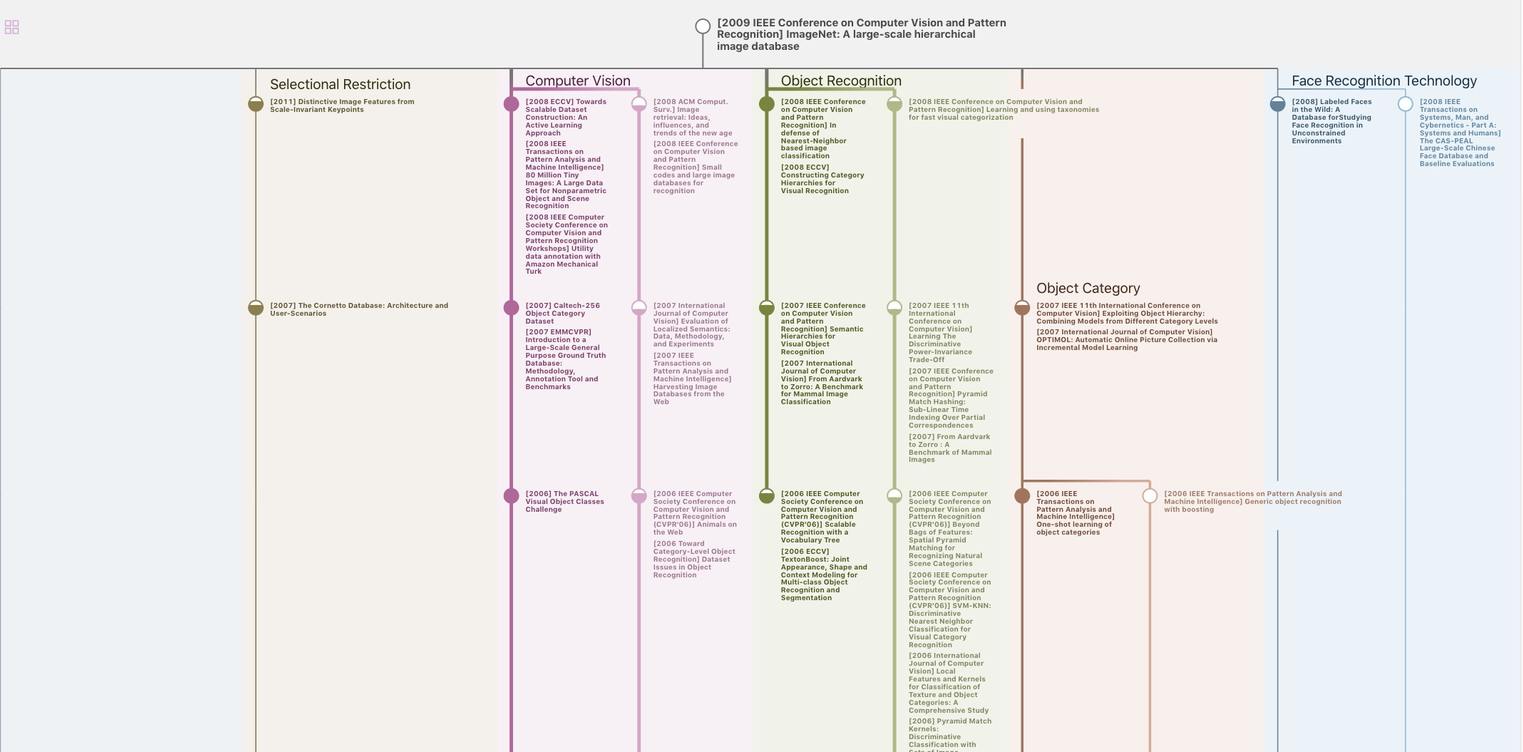
生成溯源树,研究论文发展脉络
Chat Paper
正在生成论文摘要