TRANS-Net: Transformer-Enhanced Residual-Error AlterNative Suppression Network for MRI Reconstruction
IEEE TRANSACTIONS ON INSTRUMENTATION AND MEASUREMENT(2022)
摘要
Since deep priors could exploit more intrinsic features than handcrafted prior knowledge, unrolled reconstruction methods significantly improve image quality for fast magnetic resonance imaging (MRI) reconstruction with the combination of iterative optimization and deep neural network-based regularization terms. One popular way for unrolled methods is to employ the regularization penalty on the reconstructed result within the image space. Dissimilarly, in this article, we innovatively propose a reconstruction framework termed Transformer-enhanced Residual-error AlterNative Suppression Network (TRANS-Net) for MRI reconstruction to extend the unrolled methods. Unlike some existing model-based algorithms, TRANS-Net additionally deploys the regularization term on the error maps in the residual image domain, which greatly emphasizes the high-frequency information contained in the MR images. Besides, to further explore the global spatial correlation of the reconstructed image, the transformer module is adopted to enlarge the receptive field of deep priors regularized on the reconstructed images for better tissue recovery. The quantitative and qualitative results show that the proposed TRANS-Net method has superior performance to the state-of-the-art reconstruction algorithms in anatomical structure restoration and perceptual detail preservation.
更多查看译文
关键词
Image reconstruction,Magnetic resonance imaging,Neural networks,Imaging,Reconstruction algorithms,Transformers,Convolutional neural networks,Deep prior,magnetic resonance imaging (MRI) imaging,residual image domain,transformer,unrolled reconstruction method
AI 理解论文
溯源树
样例
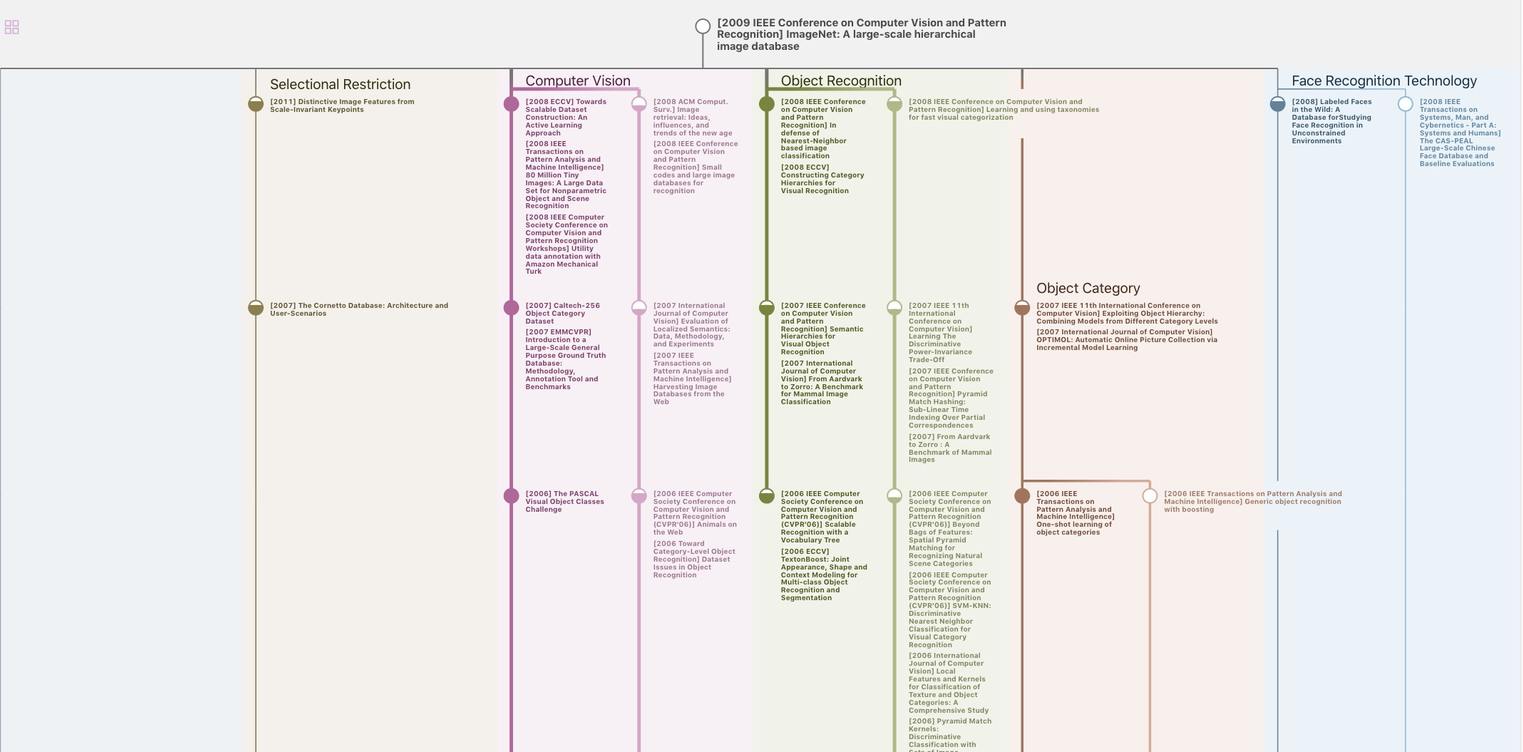
生成溯源树,研究论文发展脉络
Chat Paper
正在生成论文摘要