Alternating Direction Method of Multipliers for Decomposable Saddle-Point Problems
2022 58th Annual Allerton Conference on Communication, Control, and Computing (Allerton)(2022)
摘要
Saddle-point problems appear in various settings including machine learning, zero-sum stochastic games, and regression problems. We consider decomposable saddle-point problems and study an extension of the alternating direction method of multipliers to such saddle-point problems. Instead of solving the original saddle-point problem directly, this algorithm solves smaller saddle-point problems by exploiting the decomposable structure. We show the convergence of this algorithm for convex-concave saddle-point problems under a mild assumption. We also provide a sufficient condition for which the assumption holds. We demonstrate the convergence properties of the saddle-point alternating direction method of multipliers with numerical examples on a power allocation problem in communication channels and a network routing problem with adversarial costs.
更多查看译文
关键词
Saddle-point problems,decomposable optimization,alternating direction method of multipliers
AI 理解论文
溯源树
样例
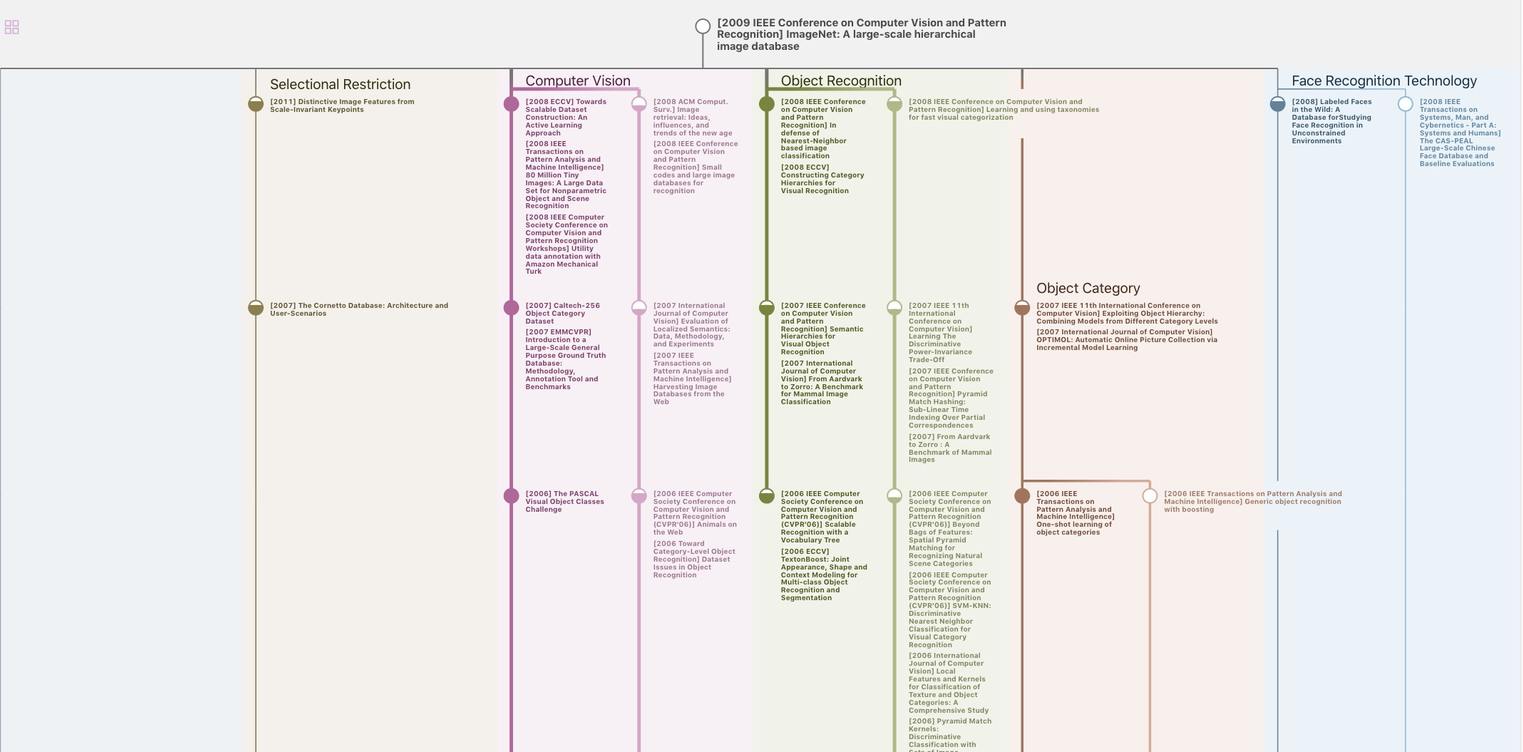
生成溯源树,研究论文发展脉络
Chat Paper
正在生成论文摘要