SELTO: Sample-Efficient Learned Topology Optimization
arxiv(2022)
摘要
We present a sample-efficient deep learning strategy for topology optimization. Our end-to-end approach is supervised and includes physics-based preprocessing and equivariant networks. We analyze how different components of our deep learning pipeline influence the number of required training samples via a large-scale comparison. The results demonstrate that including physical concepts not only drastically improves the sample efficiency but also the predictions' physical correctness. Finally, we publish two topology optimization datasets containing problems and corresponding ground truth solutions. We are confident that these datasets will improve comparability and future progress in the field.
更多查看译文
关键词
optimization,sample-efficient
AI 理解论文
溯源树
样例
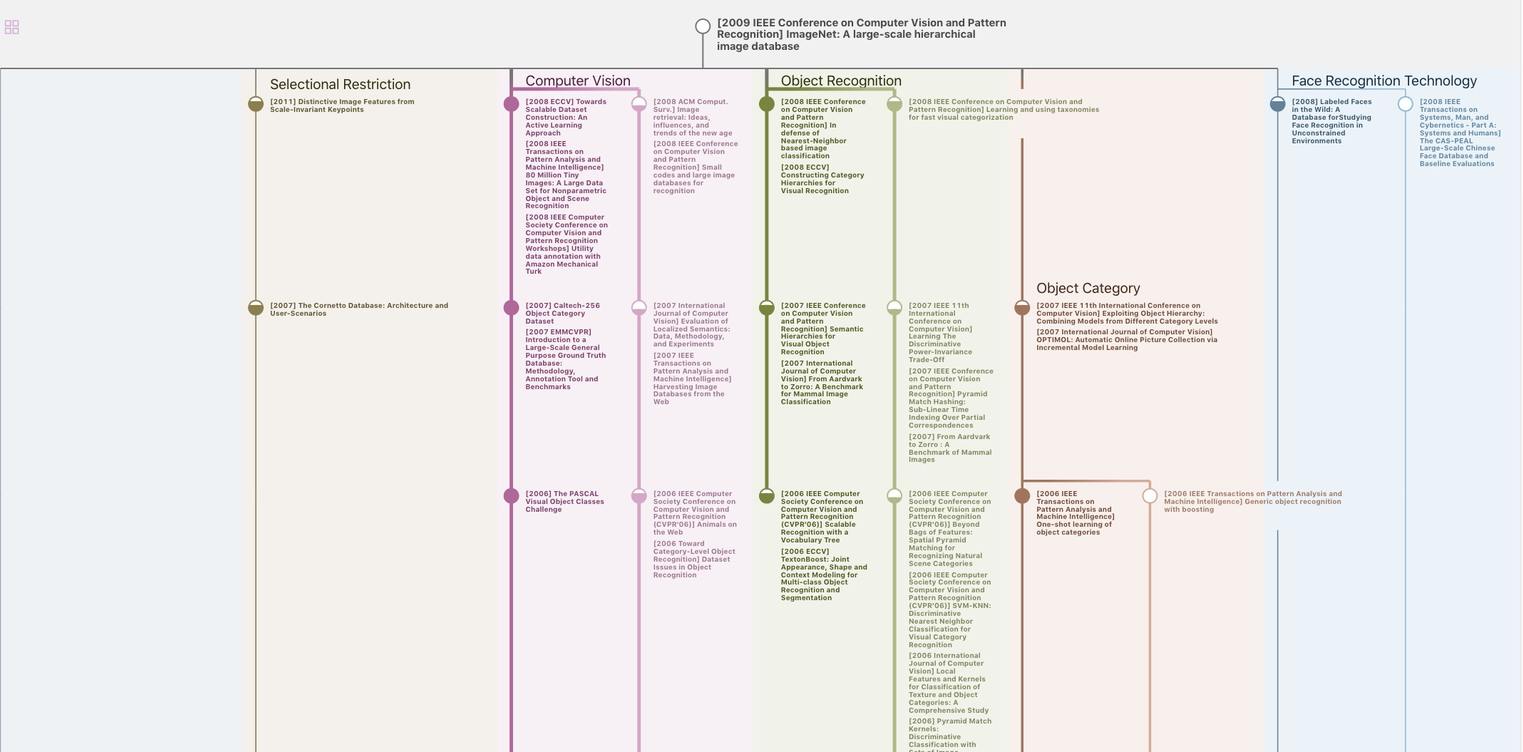
生成溯源树,研究论文发展脉络
Chat Paper
正在生成论文摘要