Style Variable and Irrelevant Learning for Generalizable Person Re-identification
arxiv(2022)
摘要
Recently, due to the poor performance of supervised person re-identification (ReID) to an unseen domain, Domain Generalization (DG) person ReID has attracted a lot of attention which aims to learn a domain-insensitive model and can resist the influence of domain bias. In this paper, we first verify through an experiment that style factors are a vital part of domain bias. Base on this conclusion, we propose a Style Variable and Irrelevant Learning (SVIL) method to eliminate the effect of style factors on the model. Specifically, we design a Style Jitter Module (SJM) in SVIL. The SJM module can enrich the style diversity of the specific source domain and reduce the style differences of various source domains. This leads to the model focusing on identity-relevant information and being insensitive to the style changes. Besides, we organically combine the SJM module with a meta-learning algorithm, maximizing the benefits and further improving the generalization ability of the model. Note that our SJM module is plug-and-play and inference cost-free. Extensive experiments confirm the effectiveness of our SVIL and our method outperforms the state-of-the-art methods on DG-ReID benchmarks by a large margin.
更多查看译文
关键词
generalizable person
AI 理解论文
溯源树
样例
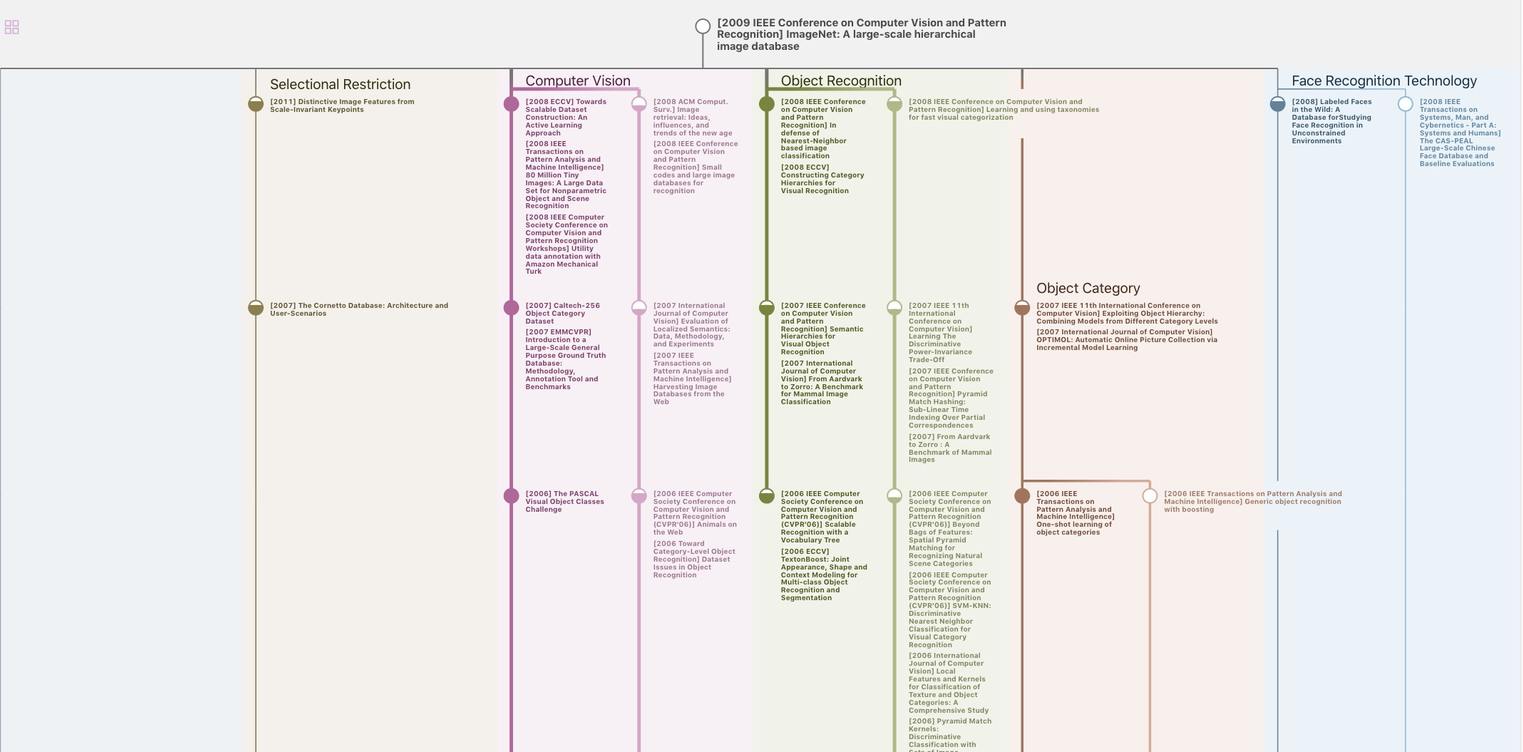
生成溯源树,研究论文发展脉络
Chat Paper
正在生成论文摘要