Unified State Representation Learning under Data Augmentation
CoRR(2022)
摘要
The capacity for rapid domain adaptation is important to increasing the applicability of reinforcement learning (RL) to real world problems. Generalization of RL agents is critical to success in the real world, yet zero-shot policy transfer is a challenging problem since even minor visual changes could make the trained agent completely fail in the new task. We propose USRA: Unified State Representation Learning under Data Augmentation, a representation learning framework that learns a latent unified state representation by performing data augmentations on its observations to improve its ability to generalize to unseen target domains. We showcase the success of our approach on the DeepMind Control Generalization Benchmark for the Walker environment and find that USRA achieves higher sample efficiency and 14.3% better domain adaptation performance compared to the best baseline results.
更多查看译文
关键词
data augmentation,representation,learning,state
AI 理解论文
溯源树
样例
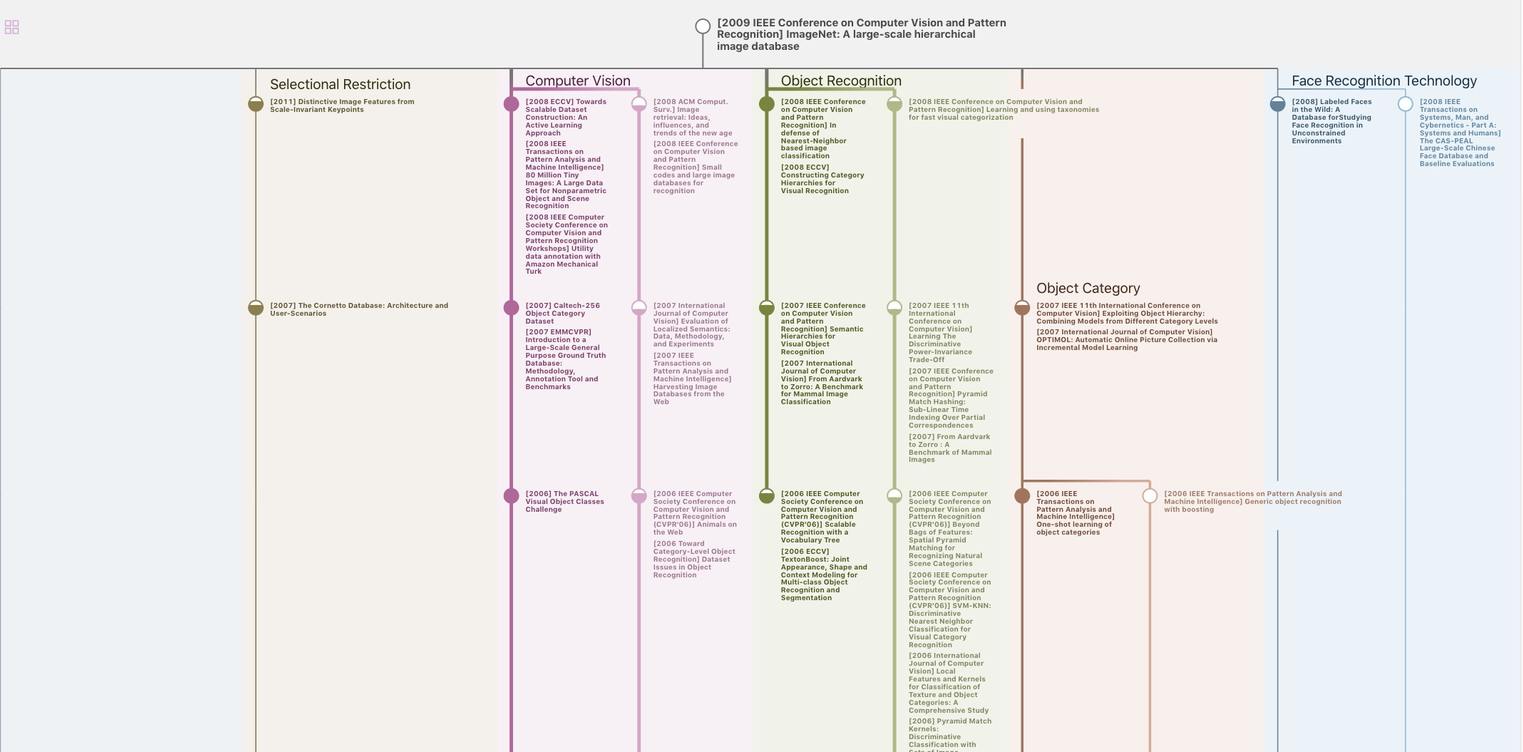
生成溯源树,研究论文发展脉络
Chat Paper
正在生成论文摘要