Low-rank constraint bipartite graph learning
Neurocomputing(2022)
摘要
Bipartite Graph-based multi-view clustering has become an active topic recently due to its efficiency in tackling large scale multi-view data. However, most existing bipartite graph-based multi-view clustering methods have the following disadvantages: 1) the clustering performance heavily depends on the predefined bipartite graph; 2) they fail to explore the complementary information embedded in multiple bipartite graphs and spatial low-rank structure hidden in each bipartite graph. To address these issues, we propose an efficient multi-view clustering method, Learning Constraint Bipartite Graphs for Multi-view Clustering (LCBG). LCBG adaptively learns each graph S(v)∈RN×M such that it well characterizes the relationship between M (M≪N) anchors and N samples in the v-th view, and takes both the intra-view and inter-view spatial low-rank structures of the learned bipartite graphs into account by minimizing tensor Schatten p-norm and nuclear norm, respectively. Finally, an efficient algorithm, which scales linearly with the data size, is proposed to solve LCBG. Extensive experimental results on five benchmark datasets indicate our proposed LCBG is superior to the state-of-the-art methods.
更多查看译文
关键词
Multi-view clustering,Graph clustering,Unsupervised learning
AI 理解论文
溯源树
样例
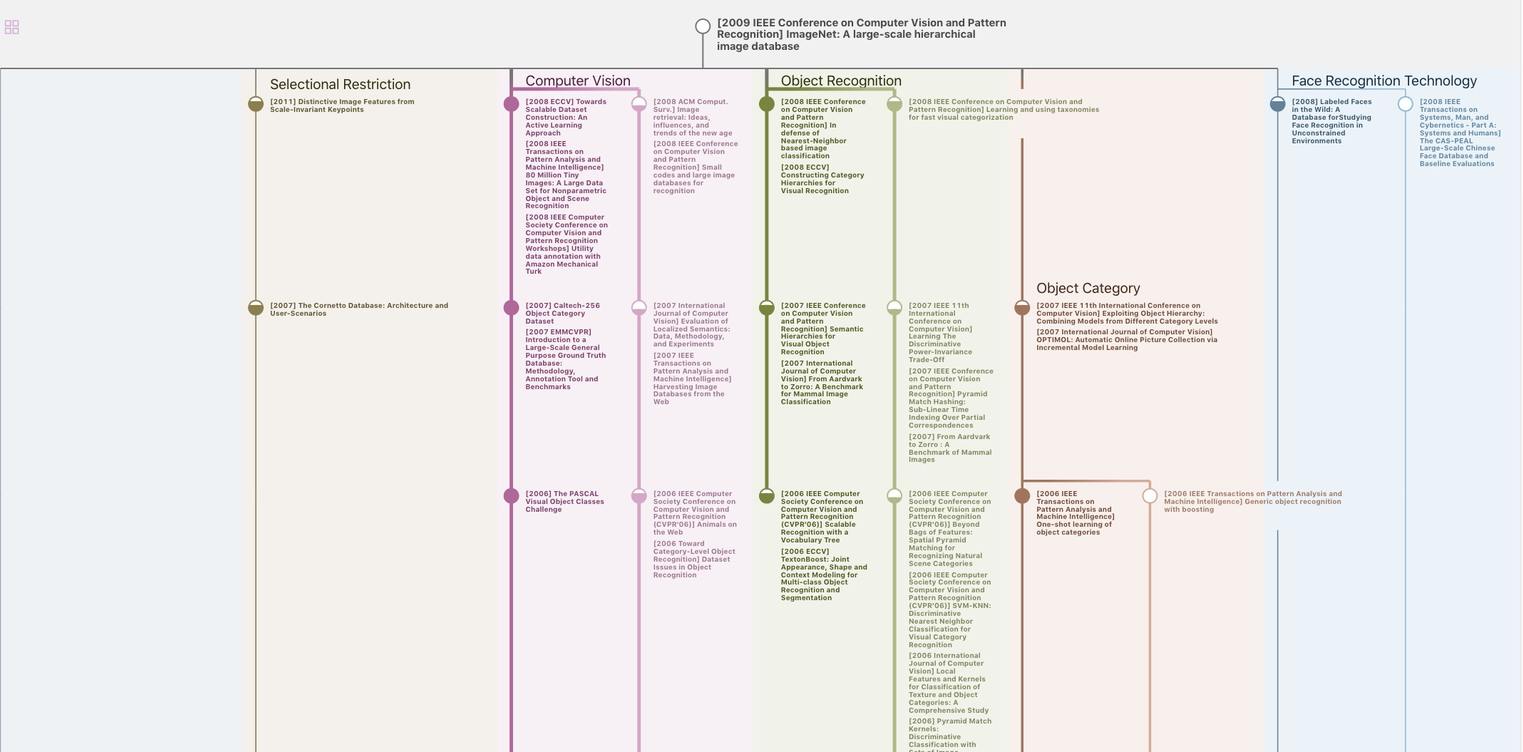
生成溯源树,研究论文发展脉络
Chat Paper
正在生成论文摘要