3-D Convolutional Neural Networks for RGB-D Salient Object Detection and Beyond
IEEE TRANSACTIONS ON NEURAL NETWORKS AND LEARNING SYSTEMS(2024)
摘要
RGB-depth (RGB-D) salient object detection (SOD) recently has attracted increasing research interest, and many deep learning methods based on encoder-decoder architectures have emerged. However, most existing RGB-D SOD models conduct explicit and controllable cross-modal feature fusion either in the single encoder or decoder stage, which hardly guarantees sufficient cross-modal fusion ability. To this end, we make the first attempt in addressing RGB-D SOD through 3-D convolutional neural networks. The proposed model, named, aims at prefusion in the encoder stage and in-depth fusion in the decoder stage to effectively promote the full integration of RGB and depth streams. Specifically, first conducts prefusion across RGB and depth modalities through a 3-D encoder obtained by inflating 2-D ResNet and later provides in-depth feature fusion by designing a 3-D decoder equipped with rich back-projection paths (RBPPs) for leveraging the extensive aggregation ability of 3-D convolutions. Toward an improved model, we propose to disentangle the conventional 3-D convolution into successive spatial and temporal convolutions and, meanwhile, discard unnecessary zero padding. This eventually results in a 2-D convolutional equivalence that facilitates optimization and reduces parameters and computation costs. Thanks to such a progressive-fusion strategy involving both the encoder and the decoder, effective and thorough interactions between the two modalities can be exploited and boost detection accuracy. As an additional boost, we also introduce channel-modality attention and its variant after each path of RBPP to attend to important features. Extensive experiments on seven widely used benchmark datasets demonstrate that and perform favorably against 14 state-of-the-art RGB-D SOD approaches in terms of five key evaluation metrics. Our code will be made publicly available at https://github.com/PPOLYpubki/RD3D.
更多查看译文
关键词
Feature extraction,Decoding,Solid modeling,Convolutional neural networks,Task analysis,Object detection,Computational modeling,3-D convolutional neural network (CNN),progressive fusion,RGB-depth (RGB-D) salient object detection (SOD),saliency detection
AI 理解论文
溯源树
样例
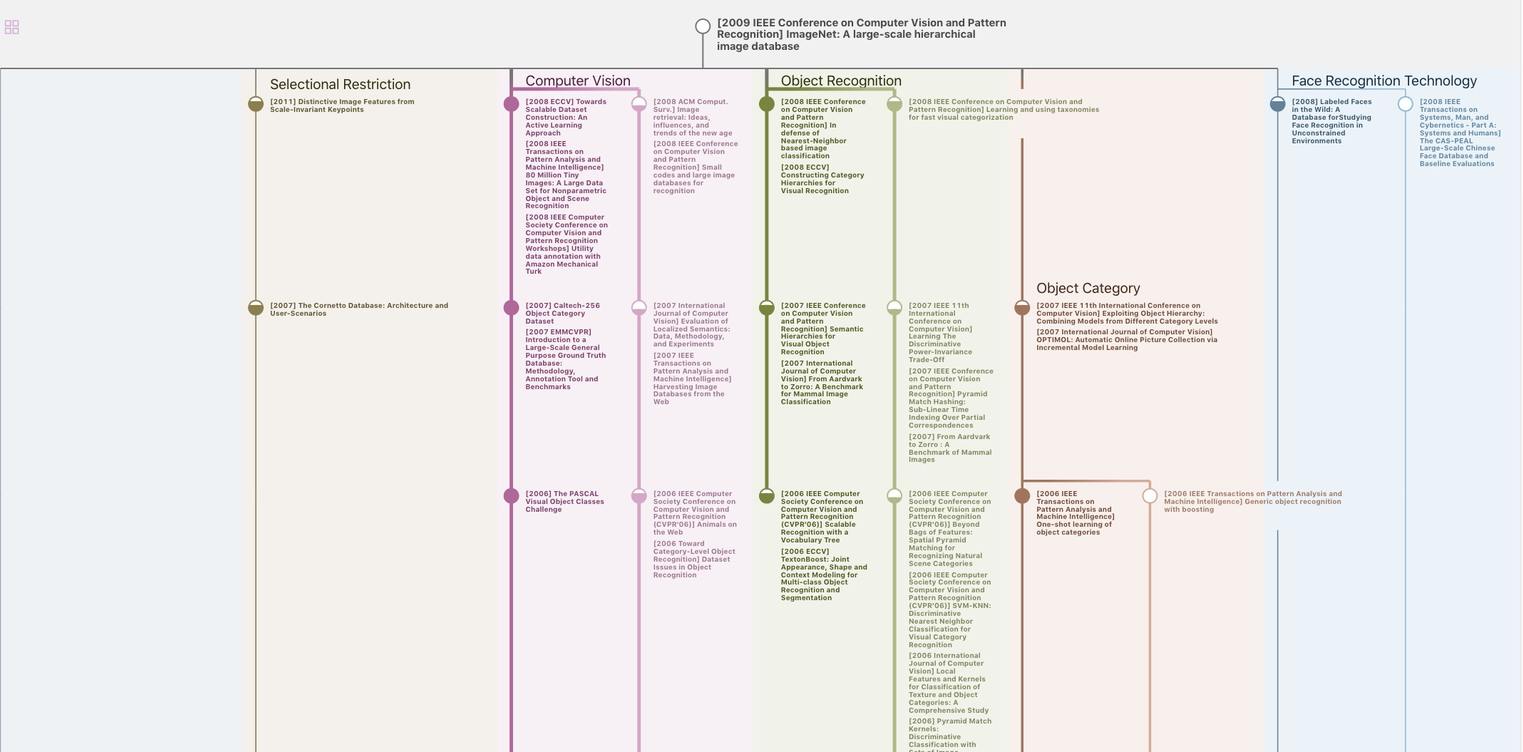
生成溯源树,研究论文发展脉络
Chat Paper
正在生成论文摘要