Multimodal Information Fusion for High-Robustness and Low-Drift State Estimation of UGVs in Diverse Scenes
IEEE TRANSACTIONS ON INSTRUMENTATION AND MEASUREMENT(2022)
摘要
Currently, the autonomous positioning of unmanned ground vehicles (UGVs) still faces the problems of insufficient persistence and poor reliability, especially in the challenging scenarios where satellites are denied, or the sensing modalities such as vision or laser are degraded. Based on multimodal information fusion and failure detection (FD), this article proposes a high-robustness and low-drift state estimation system suitable for multiple scenes, which integrates light detection and ranging (LiDAR), inertial measurement units (IMUs), stereo camera, encoders, attitude and heading reference system (AHRS) in a loose coupling way. Firstly, a state estimator with variable fusion mode is designed based on the error-state extended Kalman filtering (ES-EKF), which can fuse encoder-AHRS subsystem (EAS), visual-inertial subsystem (VIS), and LiDAR subsystem (LS) and change its integration structure online by selecting a fusion mode. Secondly, in order to improve the robustness of the whole system in challenging environments, an information manager is created, which judges the health status of subsystems by degeneration metrics, and then online selects appropriate information sources and variables to enter the estimator according to their health status. Finally, the proposed system is extensively evaluated using the datasets collected from six typical scenes: street, field, forest, forest-at-night, street-at-night and tunnel-at-night. The experimental results show our framework is better or comparable accuracy and robustness than existing publicly available systems.
更多查看译文
关键词
State estimation,Laser radar,Cameras,Reliability,Kalman filters,Feature extraction,Degradation,Error-state extended Kalman filter (ES-EKF),failure detection (FD) and handling,light detection and ranging (LiDAR)-inertial-visual-encoder odometry,multimodal information fusion,state estimation
AI 理解论文
溯源树
样例
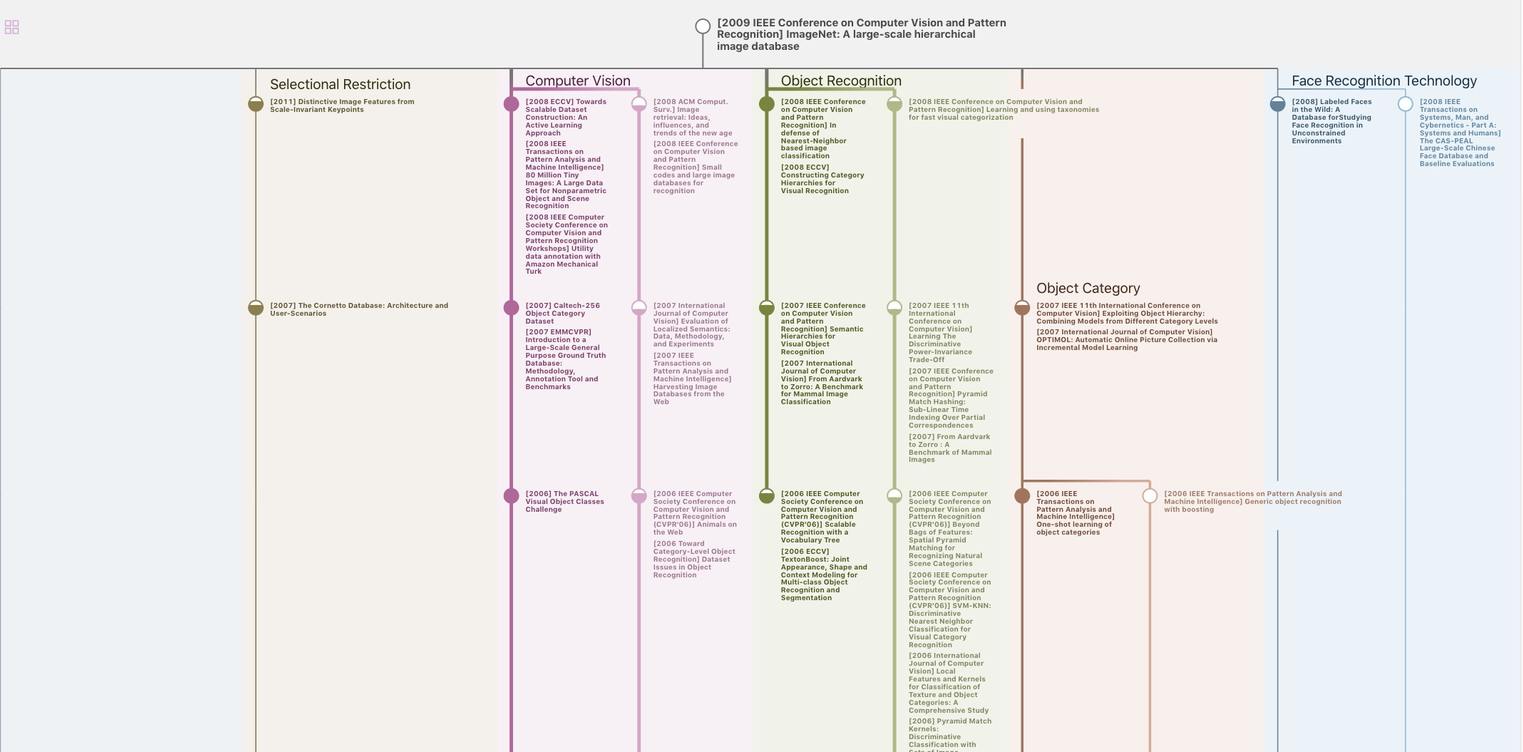
生成溯源树,研究论文发展脉络
Chat Paper
正在生成论文摘要