Group Ensemble Block: Subspace Diversity Improves Coarse-to-Fine Image Retrieval
IEEE Transactions on Artificial Intelligence(2023)
摘要
The random subspace method is an ensemble learning technique of great potential. However, its popularity does not match its potential because of its prohibitive cost and the lack of plug-and-play reusable modules. To fill this gap, we propose the random-subspace-based group ensemble block. The proposed ensemble block increases the diversity among subspaces by masking each sampled subspace. It also utilizes the diversity among a large number of subspaces, e.g., 1024, which is facilitated by combining the advantages of both averaging and concatenating strategies for merging different subspaces' outcomes. In terms of cost, the proposed ensemble block contributes few parameters by parameter sharing, negligible computations by the linear transformation, and fast running speed by parallel processing for every subspace. In terms of reusability, it encapsulates every necessary step in one small block, and can be interchangeably used as a linear layer in neural networks. We show that our group ensemble block is complementary to existing methods for image retrieval such as class-level and instance-level discrimination, and can also be deployed for other tasks such as image classification. Experiments show that the proposed group ensemble block achieves the state-of-the-art accuracy on the CIFAR-100 and ImageNet datasets for the coarse-to-fine image retrieval problem, where the model is trained with coarse-level annotation (e.g., trees) and evaluated with fine-grained category (e.g., maple trees and oak trees).
更多查看译文
关键词
Coarse-to-fine,ensemble learning,image retrieval,label granularity,random subspaces,self-supervised learning
AI 理解论文
溯源树
样例
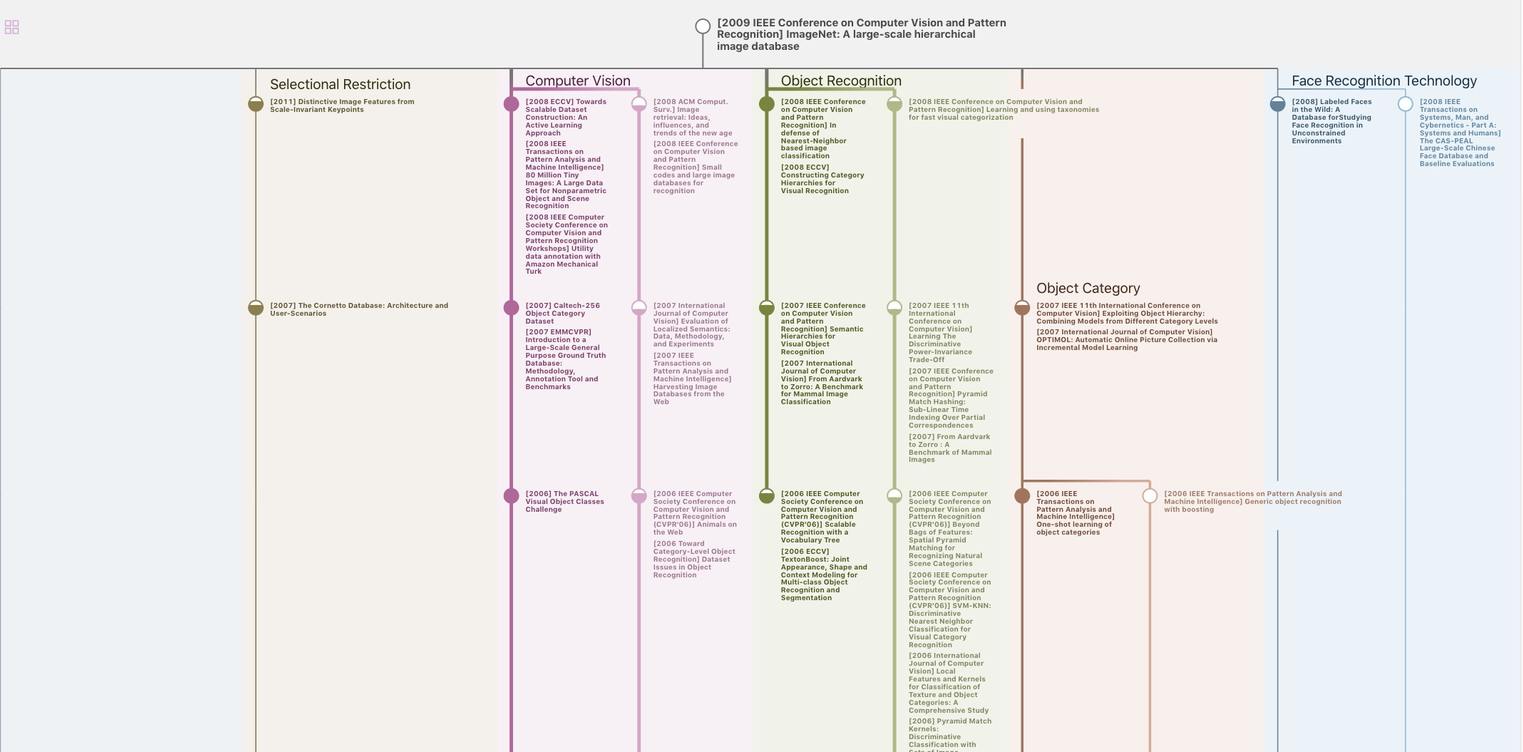
生成溯源树,研究论文发展脉络
Chat Paper
正在生成论文摘要