Short-Term Electric Net Load Forecasting for Solar-Integrated Distribution Systems Based on Bayesian Neural Networks and Statistical Post-Processing
SSRN Electronic Journal(2022)
摘要
The increasing integration of variable renewable technologies at distribution feeders, mainly solar photovoltaic (PV) systems, presents new challenges to grid operators for accurately forecasting demand. This renders the transitioning from load to net load forecasting (NLF) imperative. A new methodology was proposed in this paper for direct short-term NLF at the distribution level, using a Bayesian neural network model. The proposed model was optimized with decision heuristics based on a statistical post-processing stage (i.e., clustering of daily irradiance patterns) for improved performance. Model validation was performed using historical numerical weather predictions and net load data from three distribution feeders (with PV shares ranging from 2.5% to 34.2%) in Cyprus. The optimally constructed model achieved high forecasting accuracies, exhibiting normalized root mean square error (nRMSE) <5% when applied to the distribution feeders. Statistical post-processing further improved the model's forecasting accuracy, achieving nRMSE values <1.3%. Finally, the results demonstrated the suitability of the NLF methodology for distribution feeders with diverse PV penetration shares, rendering the proposed method applicable to distribution system operators for decision making and efficient planning.
更多查看译文
关键词
bayesian neural networks,forecasting,short-term,solar-integrated,post-processing
AI 理解论文
溯源树
样例
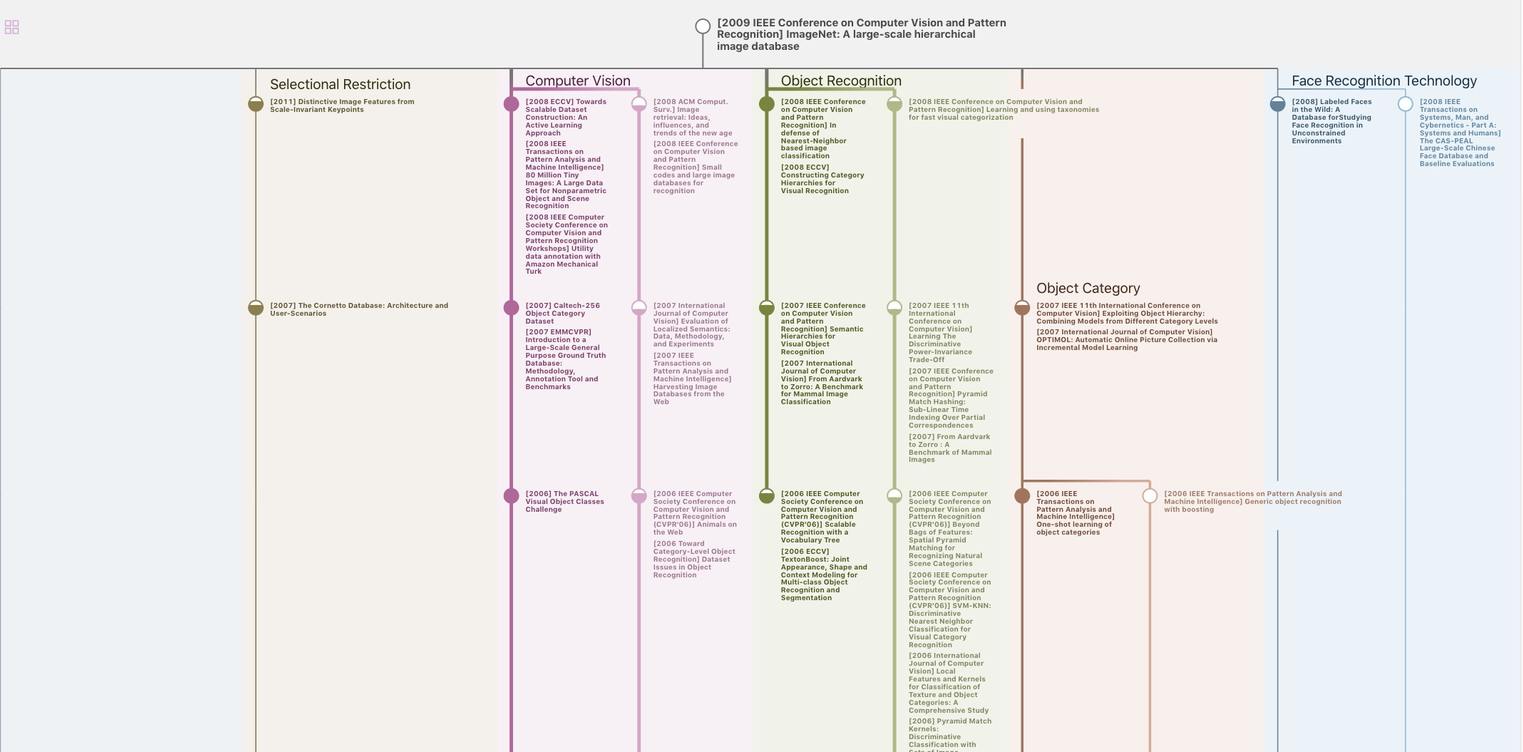
生成溯源树,研究论文发展脉络
Chat Paper
正在生成论文摘要