Deep Reinforcement Learning for Cryptocurrency Trading: Practical Approach to Address Backtest Overfitting
arxiv(2023)
摘要
Designing profitable and reliable trading strategies is challenging in the highly volatile cryptocurrency market. Existing works applied deep reinforcement learning methods and optimistically reported increased profits in backtesting, which may suffer from the false positive issue due to overfitting. In this paper, we propose a practical approach to address backtest overfitting for cryptocurrency trading using deep reinforcement learning. First, we formulate the detection of backtest overfitting as a hypothesis test. Then, we train the DRL agents, estimate the probability of overfitting, and reject the overfitted agents, increasing the chance of good trading performance. Finally, on 10 cryptocurrencies over a testing period from 05/01/2022 to 06/27/2022 (during which the crypto market crashed two times), we show that the less overfitted deep reinforcement learning agents have a higher return than that of more overfitted agents, an equal weight strategy, and the S&P DBM Index (market benchmark), offering confidence in possible deployment to a real market.
更多查看译文
关键词
cryptocurrency trading,deep reinforcement learning,backtest overfitting
AI 理解论文
溯源树
样例
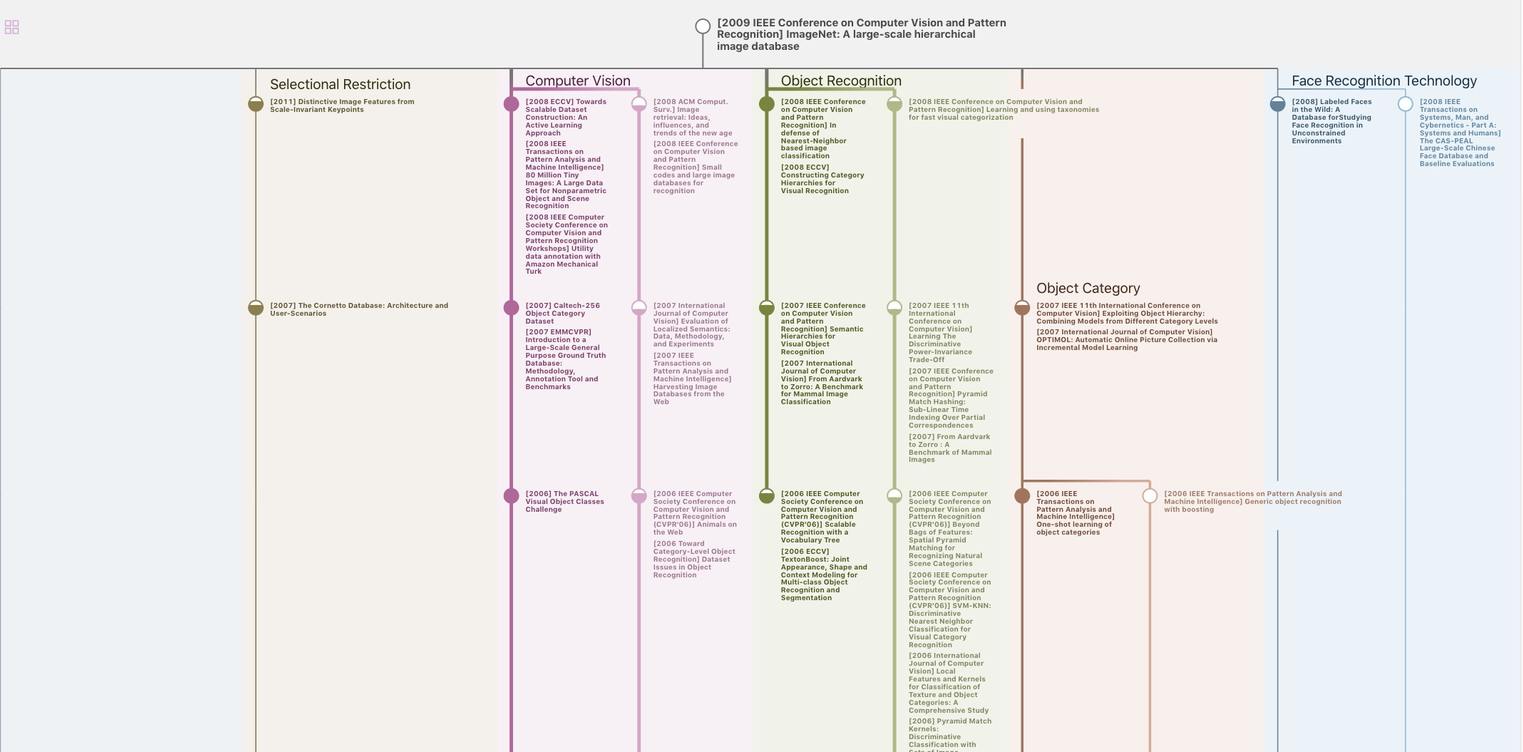
生成溯源树,研究论文发展脉络
Chat Paper
正在生成论文摘要