Multistage Temporal Convolution Transformer for Action Segmentation
SSRN Electronic Journal(2022)
摘要
This paper addresses fully supervised action segmentation. Transformers have been shown to have large model capacity and powerful sequence modeling abilities, and hence seem quite suitable for capturing action grammar in videos. However, their performance in video understanding still lags behind that of temporal convolutional networks, or ConvNets for short. We hypothesize that this is because: (i) ConvNets tend to generalize better than Transformers, and (ii) Transformer's large model capacity requires significantly larger training datasets than existing action segmentation benchmarks. We specify a new hybrid model, TCTr, that combines the strengths from both frameworks. TCTr seamlessly unifies depth-wise convolution and self-attention in a principled manner. Also, TCTr addresses the Transformer's quadratic computational and memory complexity in the sequence length by learning how to adaptively estimate attention from local temporal neighborhoods, instead of all frames. Our experiments show that TCTr significantly outperforms the state of the art on the Breakfast, GTEA, and 50Salads datasets.
更多查看译文
关键词
Action segmentation,Video understanding,Full supervision,Transformer network,Hybrid models,CNNs
AI 理解论文
溯源树
样例
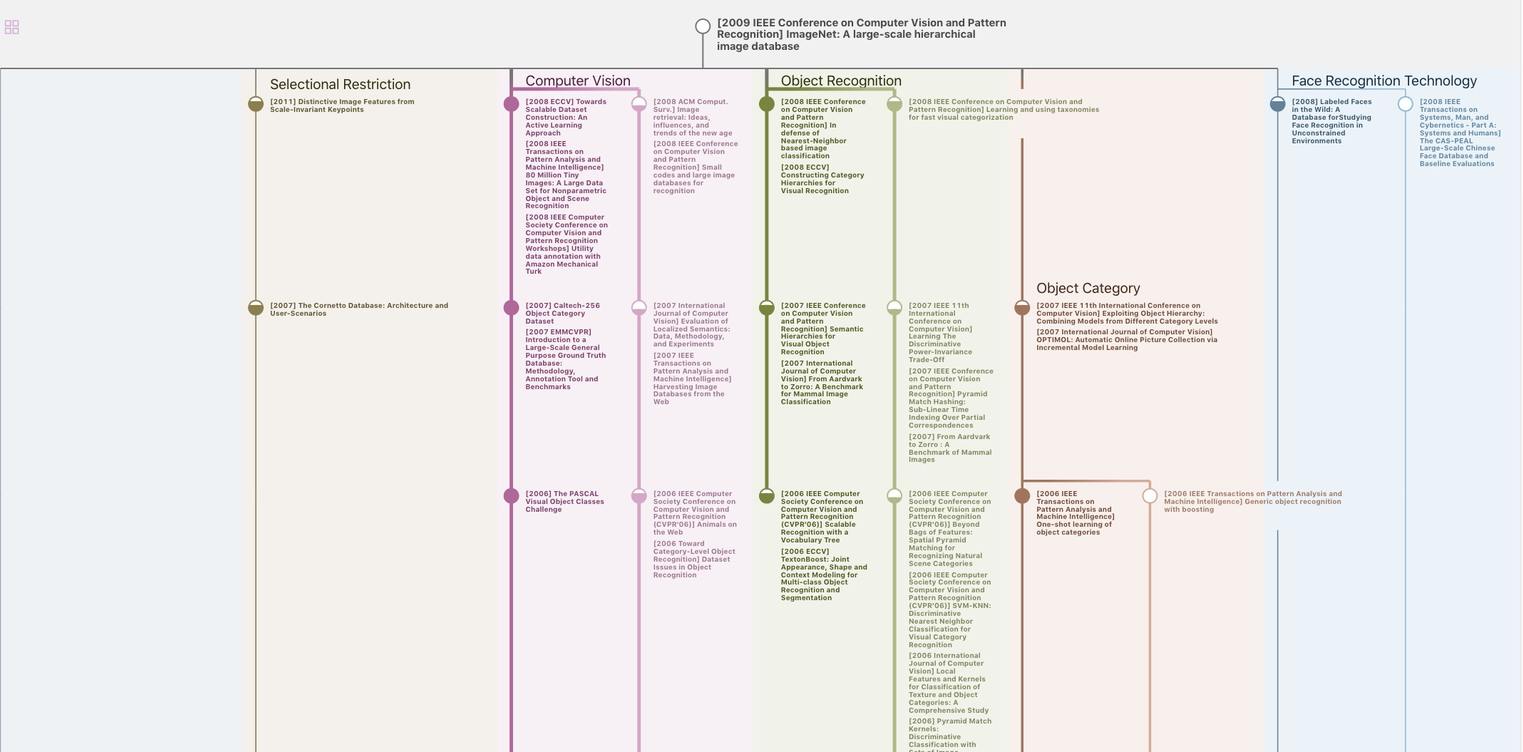
生成溯源树,研究论文发展脉络
Chat Paper
正在生成论文摘要