Data-adaptive Transfer Learning for Translation: A Case Study in Haitian and Jamaican.
International Conference on Computational Linguistics(2022)
摘要
Multilingual transfer techniques often improve low-resource machine translation (MT). Many of these techniques are applied without considering data characteristics. We show in the context of Haitian-to-English translation that transfer effectiveness is correlated with amount of training data and relationships between knowledge-sharing languages. Our experiments suggest that for some languages beyond a threshold of authentic data, back-translation augmentation methods are counterproductive, while cross-lingual transfer from a sufficiently related language is preferred. We complement this finding by contributing a rule-based French-Haitian orthographic and syntactic engine and a novel method for phonological embedding. When used with multilingual techniques, orthographic transformation makes statistically significant improvements over conventional methods. And in very low-resource Jamaican MT, code-switching with a transfer language for orthographic resemblance yields a 6.63 BLEU point advantage.
更多查看译文
关键词
translation,transfer,haitian
AI 理解论文
溯源树
样例
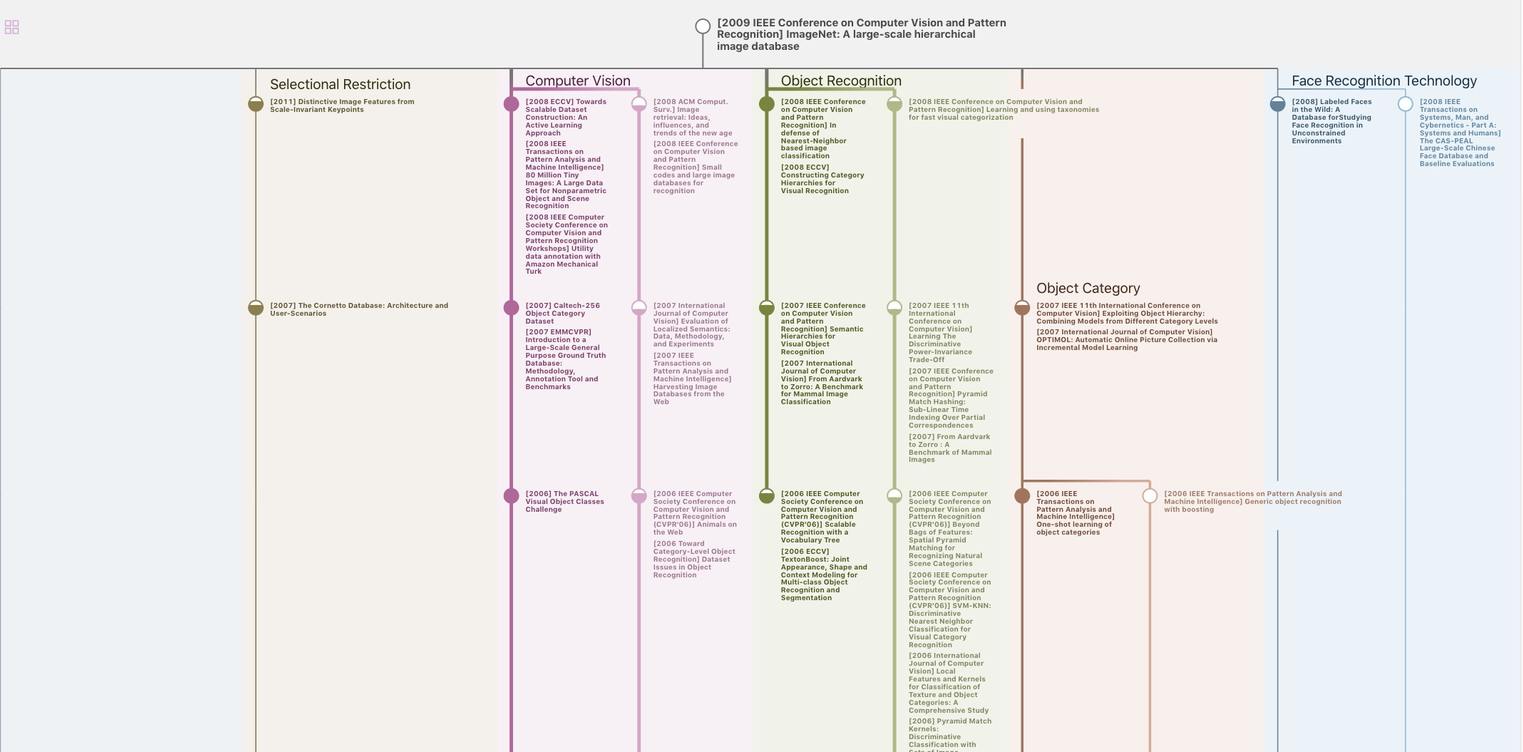
生成溯源树,研究论文发展脉络
Chat Paper
正在生成论文摘要