Finite Sample Guarantees for Distributed Online Parameter Estimation with Communication Costs
arxiv(2022)
摘要
We study the problem of estimating an unknown parameter in a distributed and online manner. Existing work on distributed online learning typically either focuses on asymptotic analysis, or provides bounds on regret. However, these results may not directly translate into bounds on the error of the learned model after a finite number of time-steps. In this paper, we propose a distributed online estimation algorithm which enables each agent in a network to improve its estimation accuracy by communicating with neighbors. We provide non-asymptotic bounds on the estimation error, leveraging the statistical properties of the underlying model. Our analysis demonstrates a trade-off between estimation error and communication costs. Further, our analysis allows us to determine a time at which the communication can be stopped (due to the costs associated with communications), while meeting a desired estimation accuracy. We also provide a numerical example to validate our results.
更多查看译文
关键词
online parameter estimation,costs
AI 理解论文
溯源树
样例
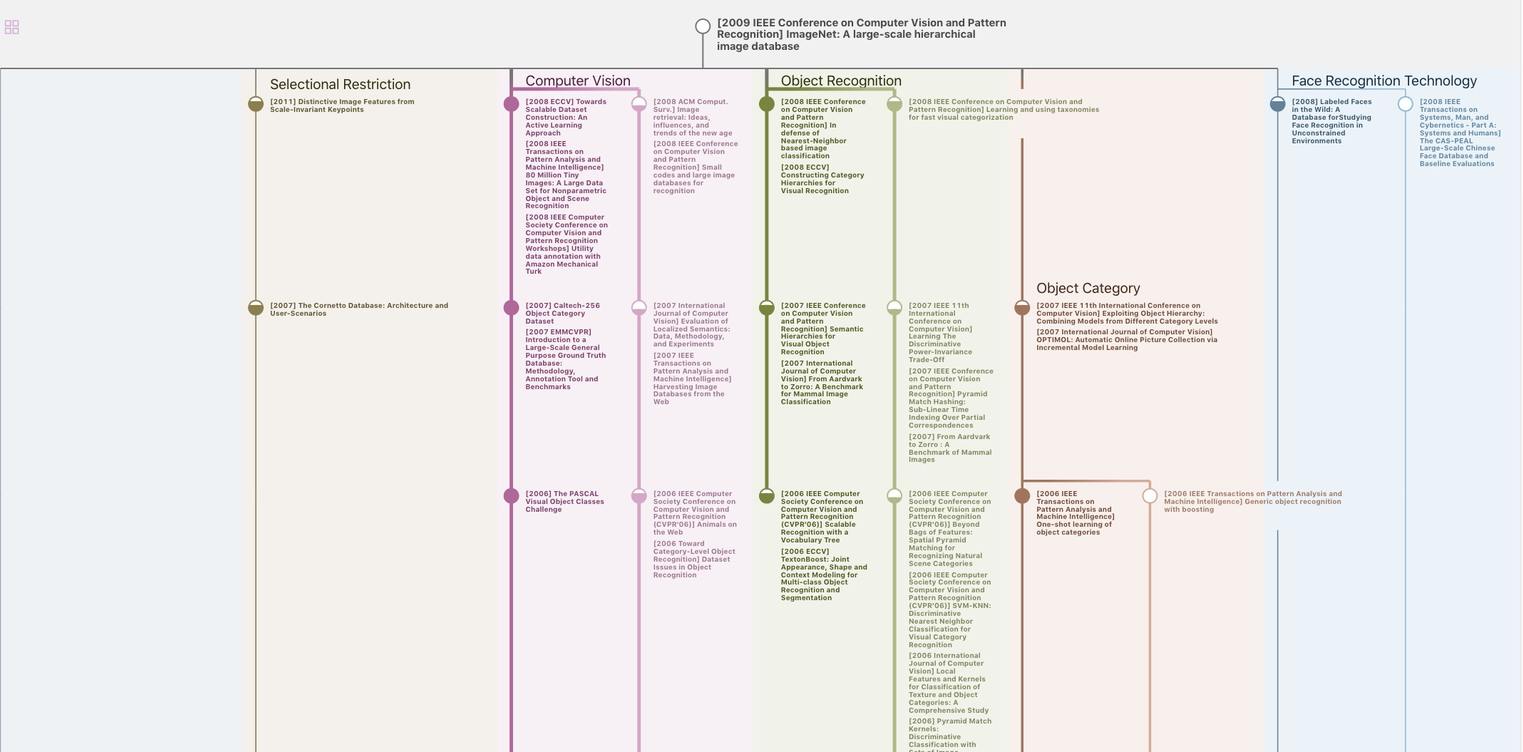
生成溯源树,研究论文发展脉络
Chat Paper
正在生成论文摘要