Early Stimulated Immune Responses Predict Clinical Disease Severity in Hospitalized COVID-19 Patients.
Communications medicine(2023)
摘要
Background:The immune pathogenesis underlying the diverse clinical course of COVID-19 is poorly understood. Currently, there is an unmet need in daily clinical practice for early biomarkers and improved risk stratification tools to help identify and monitor COVID-19 patients at risk of severe disease.Methods:We performed longitudinal assessment of stimulated immune responses in 30 patients hospitalized with COVID-19. We used the TruCulture whole-blood ligand-stimulation assay applying standardized stimuli to activate distinct immune pathways, allowing quantification of cytokine responses. We further characterized immune cell subsets by flow cytometry and used this deep immunophenotyping data to map the course of clinical disease within and between patients.Results:Here we demonstrate impairments in innate immune response pathways at time of COVID-19 hospitalization that are associated with the development of severe disease. We show that these impairments are transient in those discharged from hospital, as illustrated by functional and cellular immune reconstitution. Specifically, we identify lower levels of LPS-stimulated IL-1β, and R848-stimulated IL-12 and IL-17A, at hospital admission to be significantly associated with increasing COVID-19 disease severity during hospitalization. Furthermore, we propose a stimulated immune response signature for predicting risk of developing severe or critical COVID-19 disease at time of hospitalization, to validate in larger cohorts.Conclusions:We identify early impairments in innate immune responses that are associated with subsequent COVID-19 disease severity. Our findings provide basis for early identification of patients at risk of severe disease which may have significant implications for the early management of patients hospitalized with COVID-19.
更多查看译文
关键词
Innate immunity,Viral infection,Medicine/Public Health,general
AI 理解论文
溯源树
样例
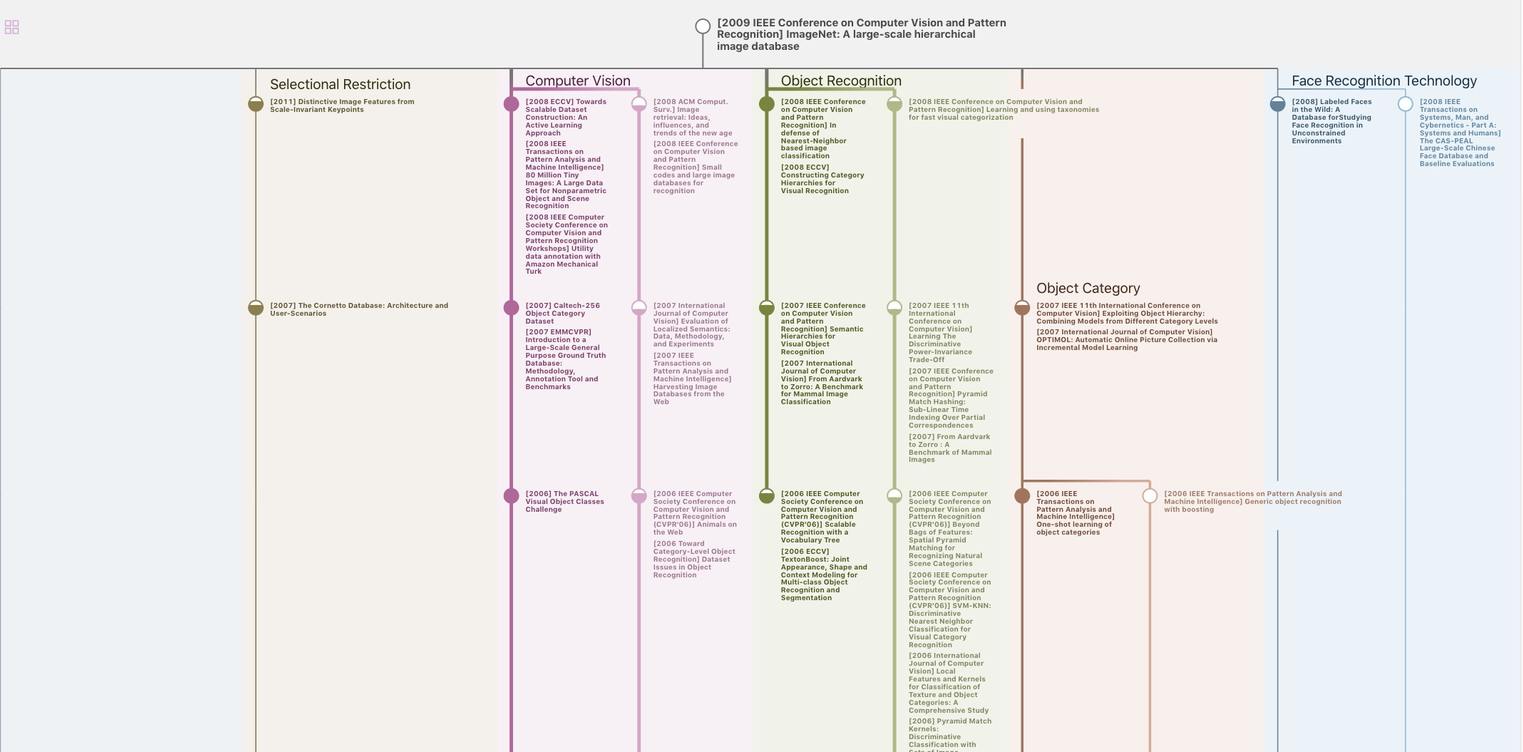
生成溯源树,研究论文发展脉络
Chat Paper
正在生成论文摘要